COLEP: Certifiably Robust Learning-Reasoning Conformal Prediction via Probabilistic Circuits
ICLR 2024(2024)
摘要
Conformal prediction has shown spurring performance in constructing
statistically rigorous prediction sets for arbitrary black-box machine learning
models, assuming the data is exchangeable. However, even small adversarial
perturbations during the inference can violate the exchangeability assumption,
challenge the coverage guarantees, and result in a subsequent decline in
empirical coverage. In this work, we propose a certifiably robust
learning-reasoning conformal prediction framework (COLEP) via probabilistic
circuits, which comprise a data-driven learning component that trains
statistical models to learn different semantic concepts, and a reasoning
component that encodes knowledge and characterizes the relationships among the
trained models for logic reasoning. To achieve exact and efficient reasoning,
we employ probabilistic circuits (PCs) within the reasoning component.
Theoretically, we provide end-to-end certification of prediction coverage for
COLEP in the presence of bounded adversarial perturbations. We also provide
certified coverage considering the finite size of the calibration set.
Furthermore, we prove that COLEP achieves higher prediction coverage and
accuracy over a single model as long as the utilities of knowledge models are
non-trivial. Empirically, we show the validity and tightness of our certified
coverage, demonstrating the robust conformal prediction of COLEP on various
datasets, including GTSRB, CIFAR10, and AwA2. We show that COLEP achieves up to
12
AwA2.
更多查看译文
关键词
conformal prediction,adversarial robustness,probabilistic circuits
AI 理解论文
溯源树
样例
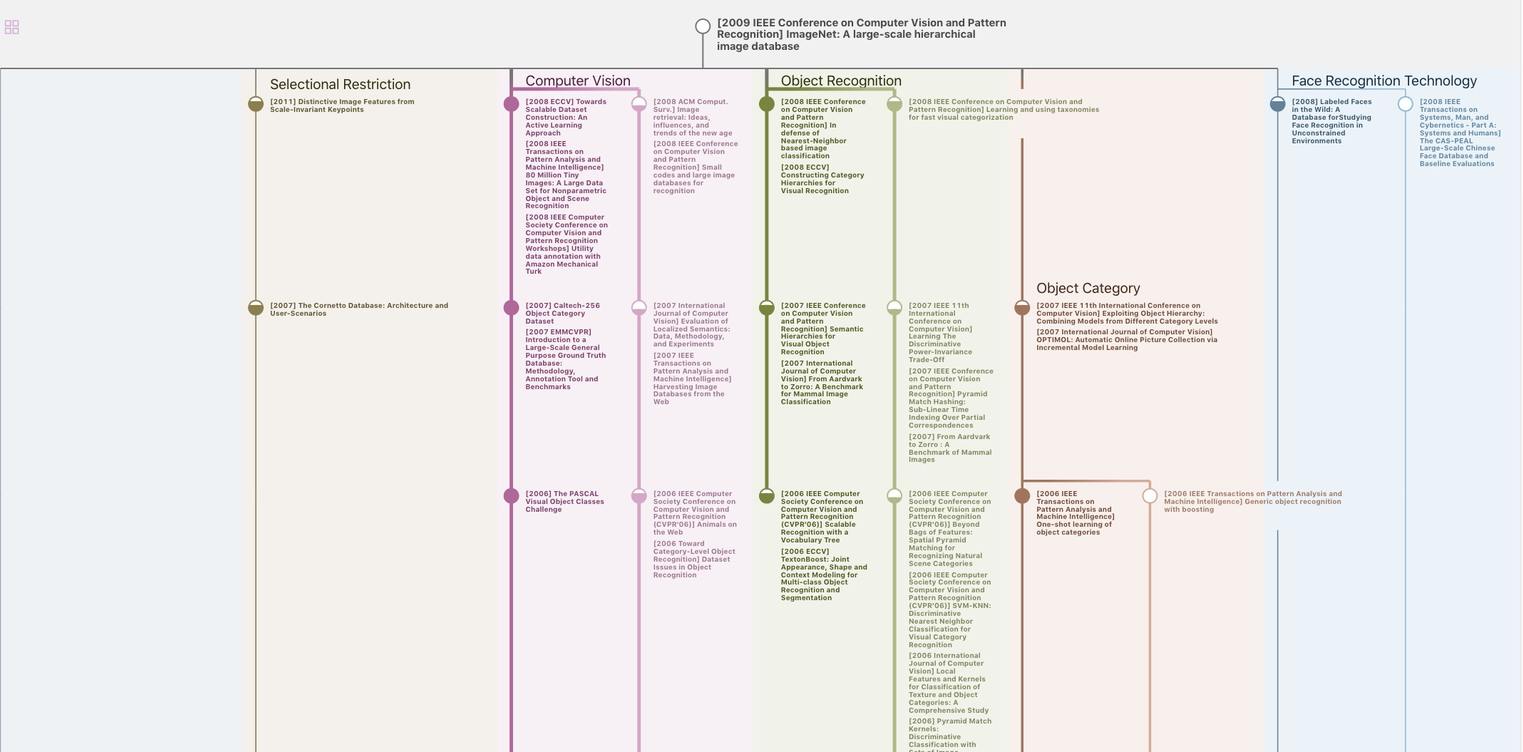
生成溯源树,研究论文发展脉络
Chat Paper
正在生成论文摘要