On the Hardness of Constrained Cooperative Multi-Agent Reinforcement Learning
ICLR 2024(2024)
摘要
Constrained cooperative multi-agent reinforcement learning (MARL) is an emerging learning framework that has been widely applied to manage multi-agent systems, and many primal-dual type algorithms have been developed for it. However, the convergence of primal-dual algorithms crucially relies on strong duality -- a condition that has not been formally proved in constrained cooperative MARL. In this work, we prove that strong duality fails to hold in constrained cooperative MARL, by revealing a nonconvex quadratic type constraint on the occupation measure induced by the product policy. Consequently, our reanalysis of the primal-dual algorithm shows that its convergence rate is hindered by the nonzero duality gap. Then, we propose a decentralized primal approach for constrained cooperative MARL to avoid the duality gap, and our analysis shows that its convergence is hindered by another gap induced by the advantage functions. Moreover, we compare these two types of algorithms via concrete examples, and show that neither of them always outperforms the other one. Our study reveals that constrained cooperative MARL is generally a challenging and highly nonconvex problem, and its fundamental structure is very different from that of single-agent constrained RL.
更多查看译文
关键词
Multi-Agent Reinforcement Learning,Constrained reinforcement learning,Primal-Dual,Duality gap,Primal algorithm
AI 理解论文
溯源树
样例
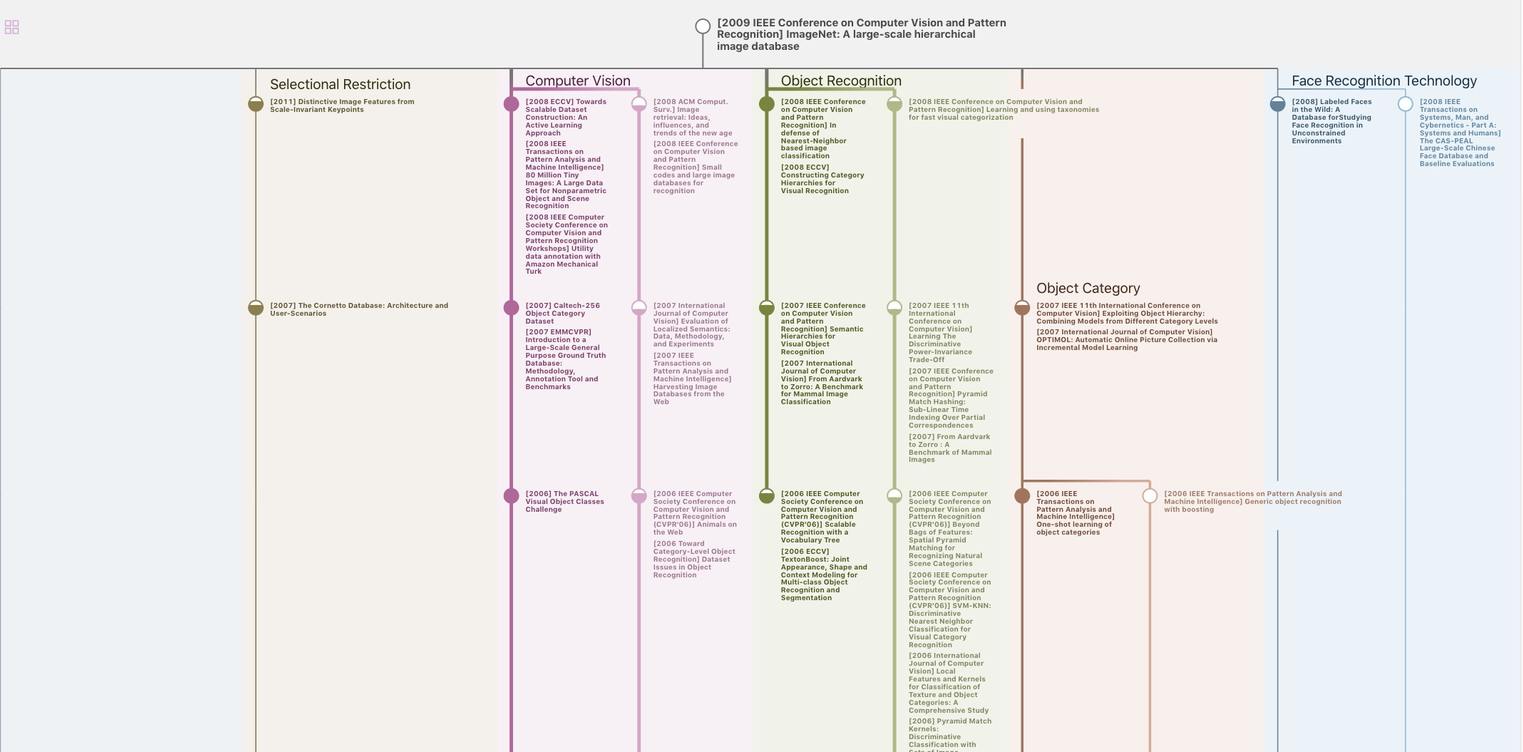
生成溯源树,研究论文发展脉络
Chat Paper
正在生成论文摘要