Learning Reusable Dense Rewards for Multi-Stage Tasks
ICLR 2024(2024)
摘要
The success of many RL techniques heavily relies on human-engineered dense rewards, which typically demands substantial domain expertise and extensive trial and error. In our work, we propose **DrS** (**D**ense **r**eward learning from **S**tages), a novel approach for learning *reusable* dense rewards for multi-stage tasks in a data-driven manner. By leveraging the stage structures of the task, DrS learns a high-quality dense reward from sparse rewards and demonstrations if given. The learned rewards can be *reused* in unseen tasks, thus reducing the human effort for reward engineering. Extensive experiments on three physical robot manipulation task families with 1000+ task variants demonstrate that our learned rewards can be reused in unseen tasks, resulting in improved performance and sample efficiency of RL algorithms. The learned rewards even achieve comparable performance to human-engineered rewards on some tasks. See our [project page](https://sites.google.com/view/iclr24drs) for videos.
更多查看译文
关键词
Reward Learning,Multi-stage Task
AI 理解论文
溯源树
样例
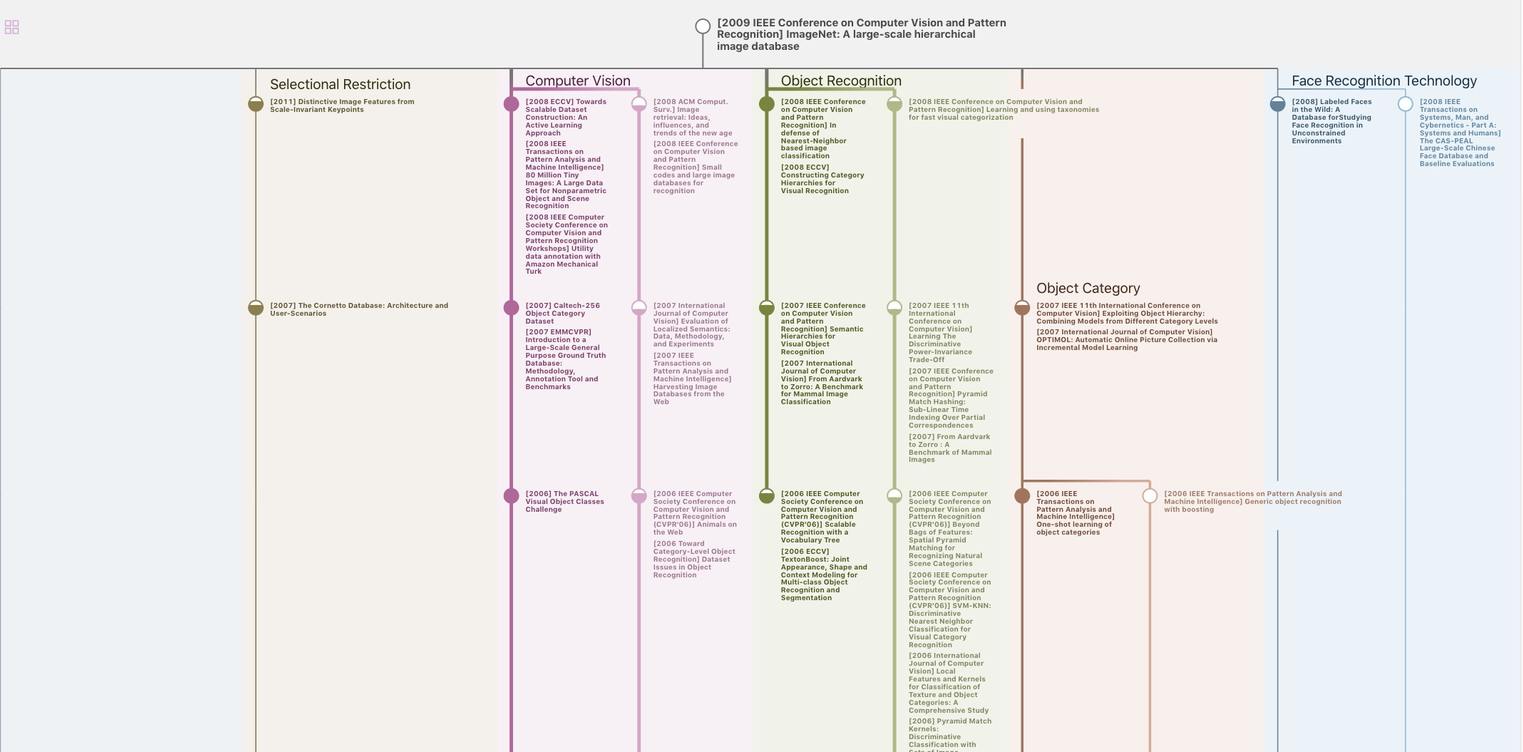
生成溯源树,研究论文发展脉络
Chat Paper
正在生成论文摘要