Do Generated Data Always Help Contrastive Learning?
ICLR 2024(2024)
摘要
Contrastive Learning (CL) has emerged as one of the most successful paradigms
for unsupervised visual representation learning, yet it often depends on
intensive manual data augmentations. With the rise of generative models,
especially diffusion models, the ability to generate realistic images close to
the real data distribution has been well recognized. These generated
high-equality images have been successfully applied to enhance contrastive
representation learning, a technique termed “data inflation”. However, we
find that the generated data (even from a good diffusion model like DDPM) may
sometimes even harm contrastive learning. We investigate the causes behind this
failure from the perspective of both data inflation and data augmentation. For
the first time, we reveal the complementary roles that stronger data inflation
should be accompanied by weaker augmentations, and vice versa. We also provide
rigorous theoretical explanations for these phenomena via deriving its
generalization bounds under data inflation. Drawing from these insights, we
propose Adaptive Inflation (AdaInf), a purely data-centric strategy without
introducing any extra computation cost. On benchmark datasets, AdaInf can bring
significant improvements for various contrastive learning methods. Notably,
without using external data, AdaInf obtains 94.70
with SimCLR, setting a new record that surpasses many sophisticated methods.
Code is available at https://github.com/PKU-ML/adainf.
更多查看译文
关键词
Contrastive Learning,Diffusion Model,Representation Learning,Self-supervised Learning,Deep Learning
AI 理解论文
溯源树
样例
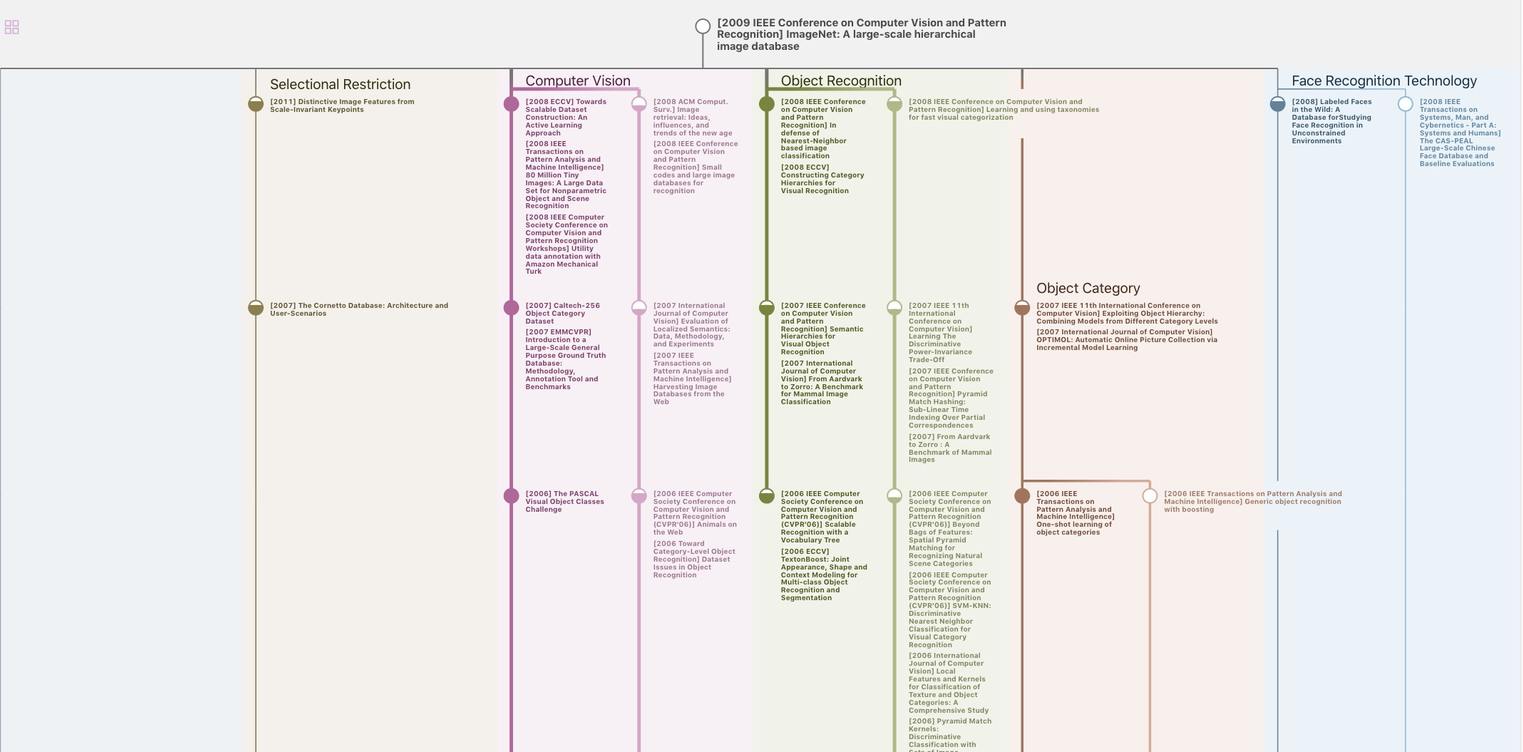
生成溯源树,研究论文发展脉络
Chat Paper
正在生成论文摘要