RECOMP: Improving Retrieval-Augmented LMs with Context Compression and Selective Augmentation
ICLR 2024(2024)
摘要
Retrieval-augmented language models improve language models (LMs) by retrieving documents and prepending them in-context.
However, these documents, often spanning hundreds of words, make inference substantially less efficient. We propose compressing the retrieved documents into textual summaries prior to in-context integration. This not only reduces the computational costs but also relieve the burden of LMs to identify relevant information in long retrieved documents. We present two compressors -- an extractive compressor which selects useful sentences from retrieved documents and an abstractive compressor which generates summary by synthesizing information from multiple documents. Both are trained to achieve performance gain in LMs when we prepend the generated summary from the compressor to LMs' input, while minimizing the summary length. When retrieved documents are irrelevant to the input or offer no additional information to LM, our compressors output an empty string, enabling selective augmentation. We evaluate our approach on the language modeling task and open domain question answering task. We achieve a compression rate of as low as 6% with minimal loss in performance for both tasks, significantly outperforming the off-the-shelf summarization models. We show that our compressors trained for one LM can transfer to other LMs on the language modeling task and provide a summary largely faithful to the retrieved documents.
更多查看译文
关键词
retrieval augmented language model,language modeling,question answering,summarization,distillation
AI 理解论文
溯源树
样例
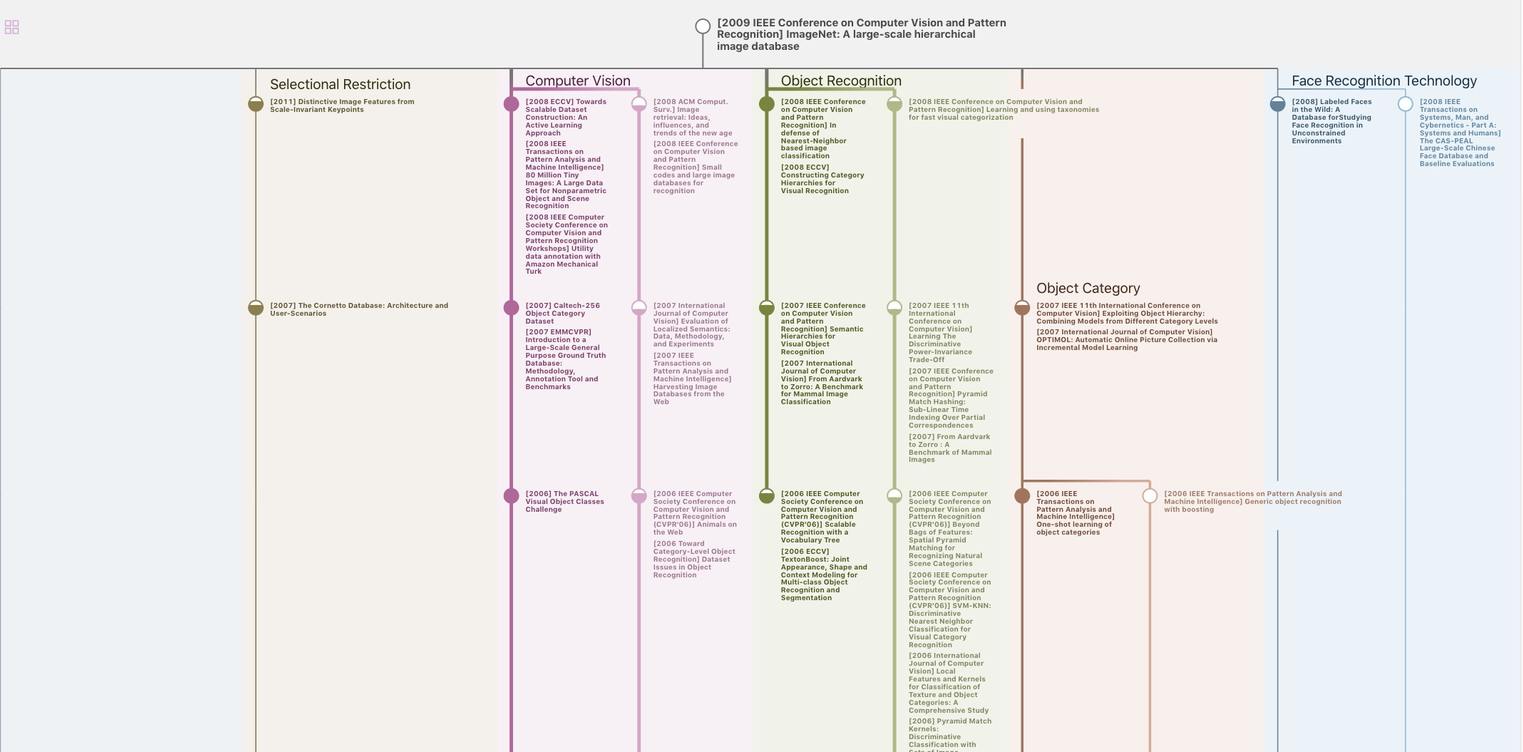
生成溯源树,研究论文发展脉络
Chat Paper
正在生成论文摘要