Multisize Dataset Condensation
ICLR 2024(2024)
摘要
While dataset condensation effectively enhances training efficiency, its
application in on-device scenarios brings unique challenges. 1) Due to the
fluctuating computational resources of these devices, there's a demand for a
flexible dataset size that diverges from a predefined size. 2) The limited
computational power on devices often prevents additional condensation
operations. These two challenges connect to the "subset degradation problem" in
traditional dataset condensation: a subset from a larger condensed dataset is
often unrepresentative compared to directly condensing the whole dataset to
that smaller size. In this paper, we propose Multisize Dataset Condensation
(MDC) by compressing N condensation processes into a single condensation
process to obtain datasets with multiple sizes. Specifically, we introduce an
"adaptive subset loss" on top of the basic condensation loss to mitigate the
"subset degradation problem". Our MDC method offers several benefits: 1) No
additional condensation process is required; 2) reduced storage requirement by
reusing condensed images. Experiments validate our findings on networks
including ConvNet, ResNet and DenseNet, and datasets including SVHN, CIFAR-10,
CIFAR-100 and ImageNet. For example, we achieved 6.40
on condensing CIFAR-10 to ten images per class. Code is available at:
https://github.com/he-y/Multisize-Dataset-Condensation.
更多查看译文
关键词
Dataset Condensation,Dataset Distillation,Image Classification
AI 理解论文
溯源树
样例
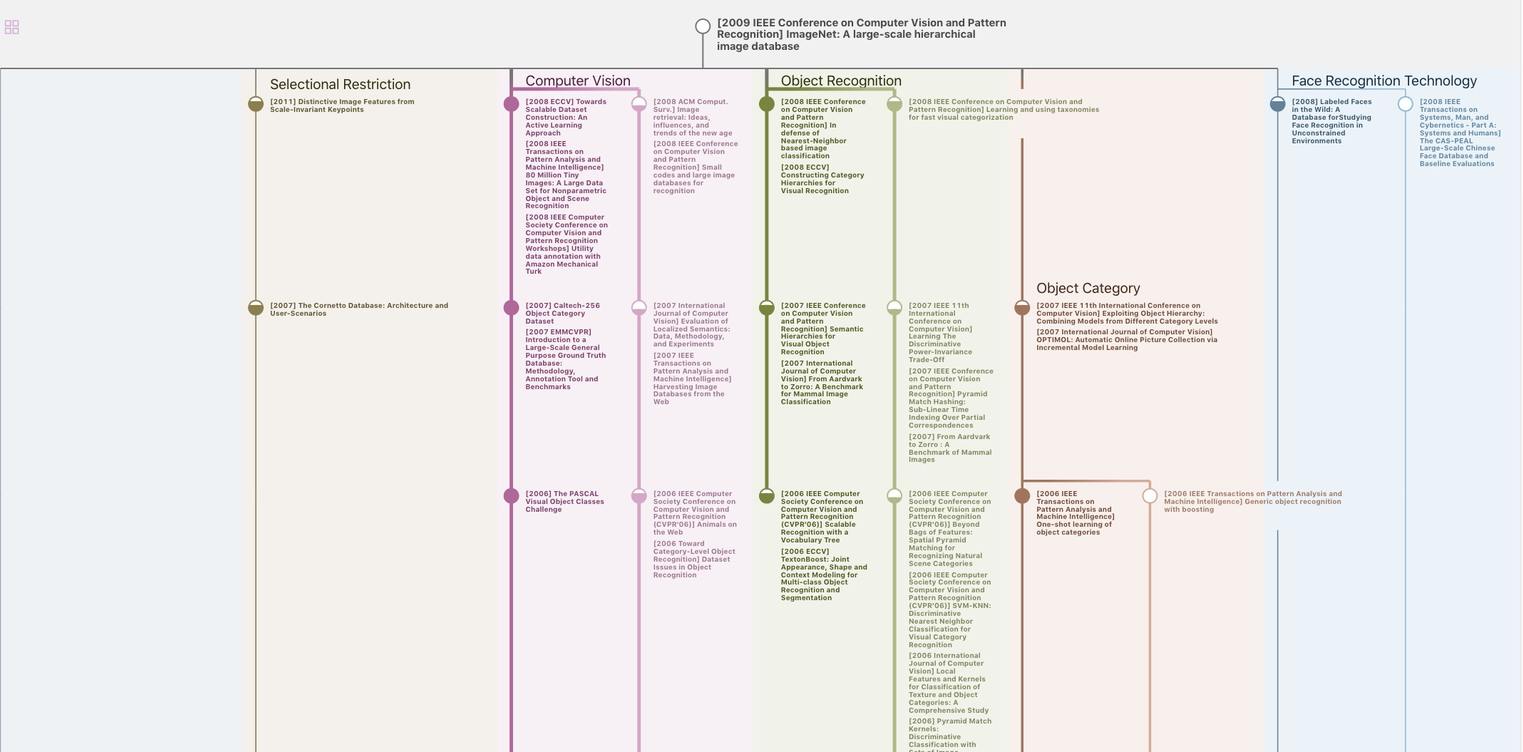
生成溯源树,研究论文发展脉络
Chat Paper
正在生成论文摘要