GraphGuard: Provably Robust Graph Classification against Adversarial Attacks
ICLR 2024(2024)
摘要
Graph classification, which aims to predict a label for a graph, has many real-world applications such as malware detection, fraud detection, and healthcare. However, many studies show an attacker could carefully perturb the structure and/or node features in a graph such that a graph classifier misclassifies the perturbed graph. Such vulnerability impedes the deployment of graph classification in security/safety-critical applications. Existing empirical defenses lack formal robustness guarantees and could be broken by adaptive or unknown attacks. Existing provable defenses have the following limitations: 1) they achieve sub-optimal robustness guarantees for graph structure perturbation, 2) they cannot provide robustness guarantees for arbitrarily node feature perturbations, 3) their robustness guarantees are probabilistic, meaning they could be incorrect with a non-zero probability, and 4) they incur large computation costs. We aim to address those limitations in this work. We propose GraphGuard, a certified defense against both graph structure and node feature perturbations for graph classification. Our GraphGuard provably predicts the same label for a graph when the number of perturbed edges and the number of nodes with perturbed features are bounded. Our results on 8 benchmark datasets show GraphGuard outperforms three state-of-the-art methods.
更多查看译文
关键词
Adversarial attacks to graph classification,provable robustness
AI 理解论文
溯源树
样例
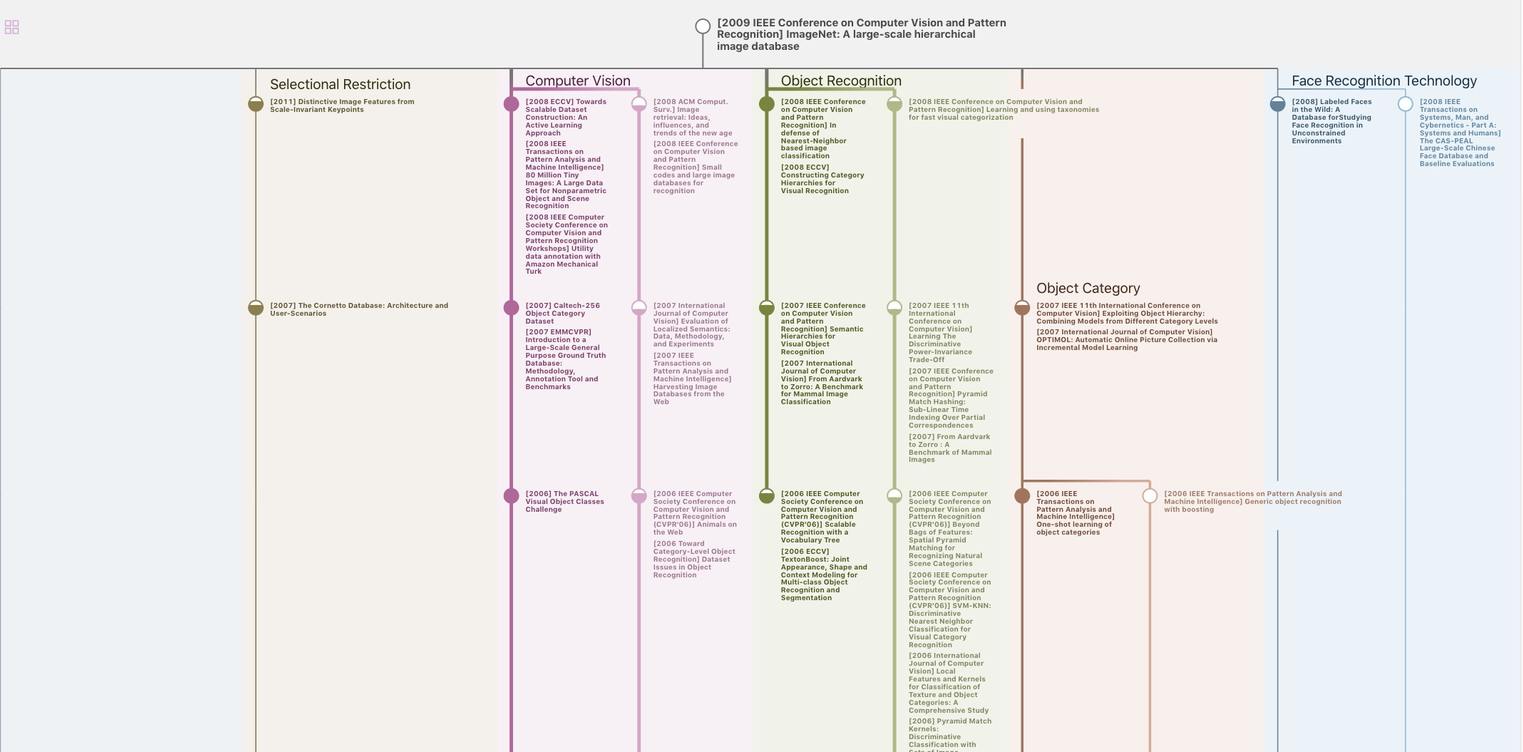
生成溯源树,研究论文发展脉络
Chat Paper
正在生成论文摘要