Consistent Multi-Class Classification from Multiple Unlabeled Datasets
ICLR 2024(2024)
摘要
Weakly supervised learning aims to construct effective predictive models from imperfectly labeled data. The recent trend of weakly supervised learning has focused on how to learn an accurate classifier from completely unlabeled data, given little supervised information such as class priors. In this paper, we consider a newly proposed weakly supervised learning problem called multi-class classification from multiple unlabeled datasets, where only multiple sets of unlabeled data and their class priors (i.e., the proportions of each class) are provided for training the classifier. To solve this problem, we first propose a classifier-consistent method (CCM) based on a probability transition matrix. However, CCM cannot guarantee risk consistency and lacks of purified supervision information during training. Therefore, we further propose a risk-consistent method (RCM) that progressively purifies supervision information during training by importance weighting. We provide comprehensive theoretical analyses for our methods to demonstrate the statistical consistency. Experimental results on multiple benchmark datasets and various prior matrices demonstrate the superiority of our proposed methods.
更多查看译文
关键词
mutli-class classification,multiple unlabeled datasets,learning consistency
AI 理解论文
溯源树
样例
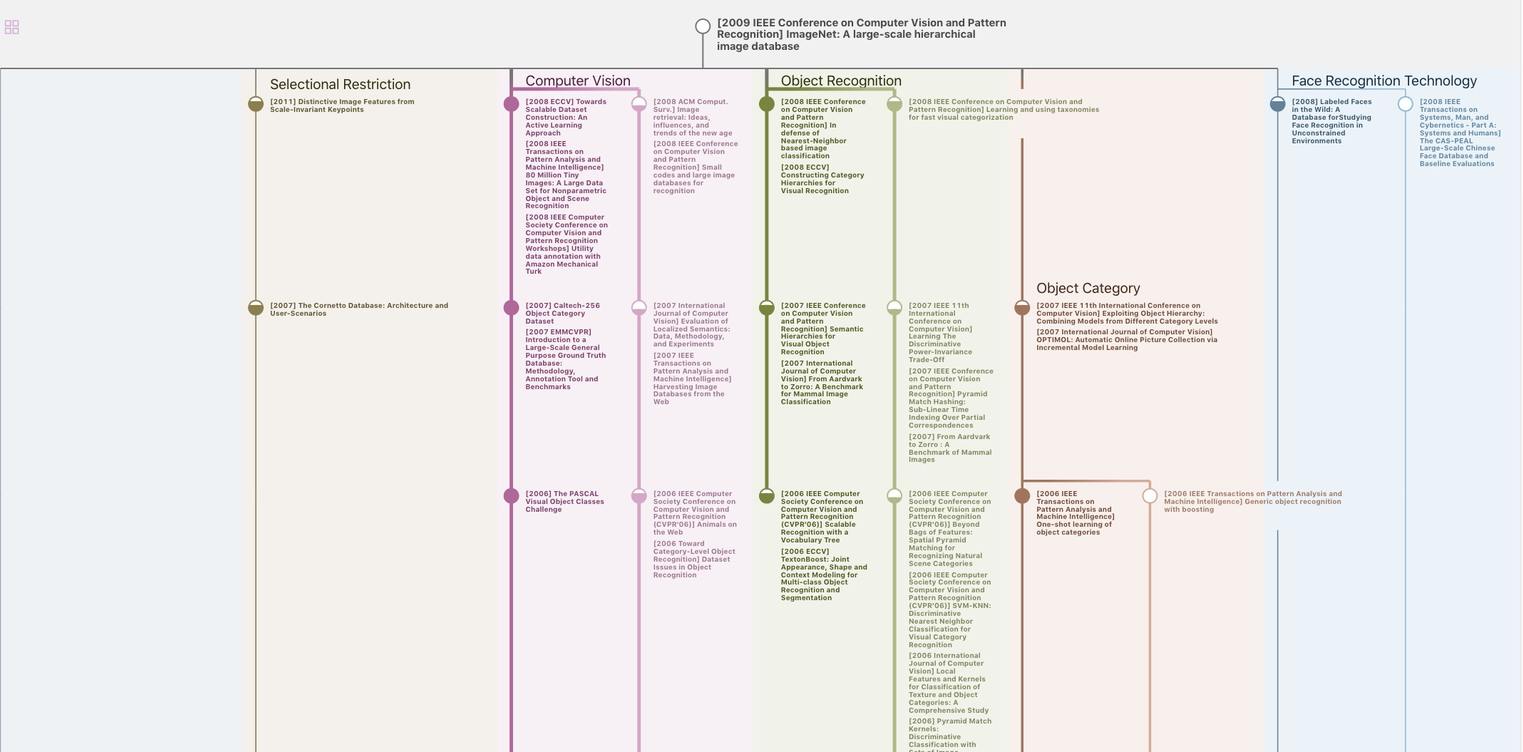
生成溯源树,研究论文发展脉络
Chat Paper
正在生成论文摘要