Kernel Metric Learning for In-Sample Off-Policy Evaluation of Deterministic RL Policies
ICLR 2024(2024)
摘要
We consider off-policy evaluation (OPE) of deterministic target policies for reinforcement learning (RL) in environments with continuous action spaces. While it is common to use importance sampling for OPE, it suffers from high variance when the behavior policy deviates significantly from the target policy. In order to address this issue, some recent works on OPE proposed in-sample learning with importance resampling. Yet, these approaches are not applicable to deterministic target policies for continuous action spaces. To address this limitation, we propose to relax the deterministic target policy using a kernel and learn the kernel metrics that minimize the overall mean squared error of the estimated temporal difference update vector of an action value function, where the action value function is used for policy evaluation. We derive the bias and variance of the estimation error due to this relaxation and provide analytic solutions for the optimal kernel metric. In empirical studies using various test domains, we show that the OPE with in-sample learning using the kernel with optimized metric achieves significantly improved accuracy than other baselines.
更多查看译文
关键词
off-policy evaluation,reinforcement learning,deterministic policy,continuous actions,metric learning
AI 理解论文
溯源树
样例
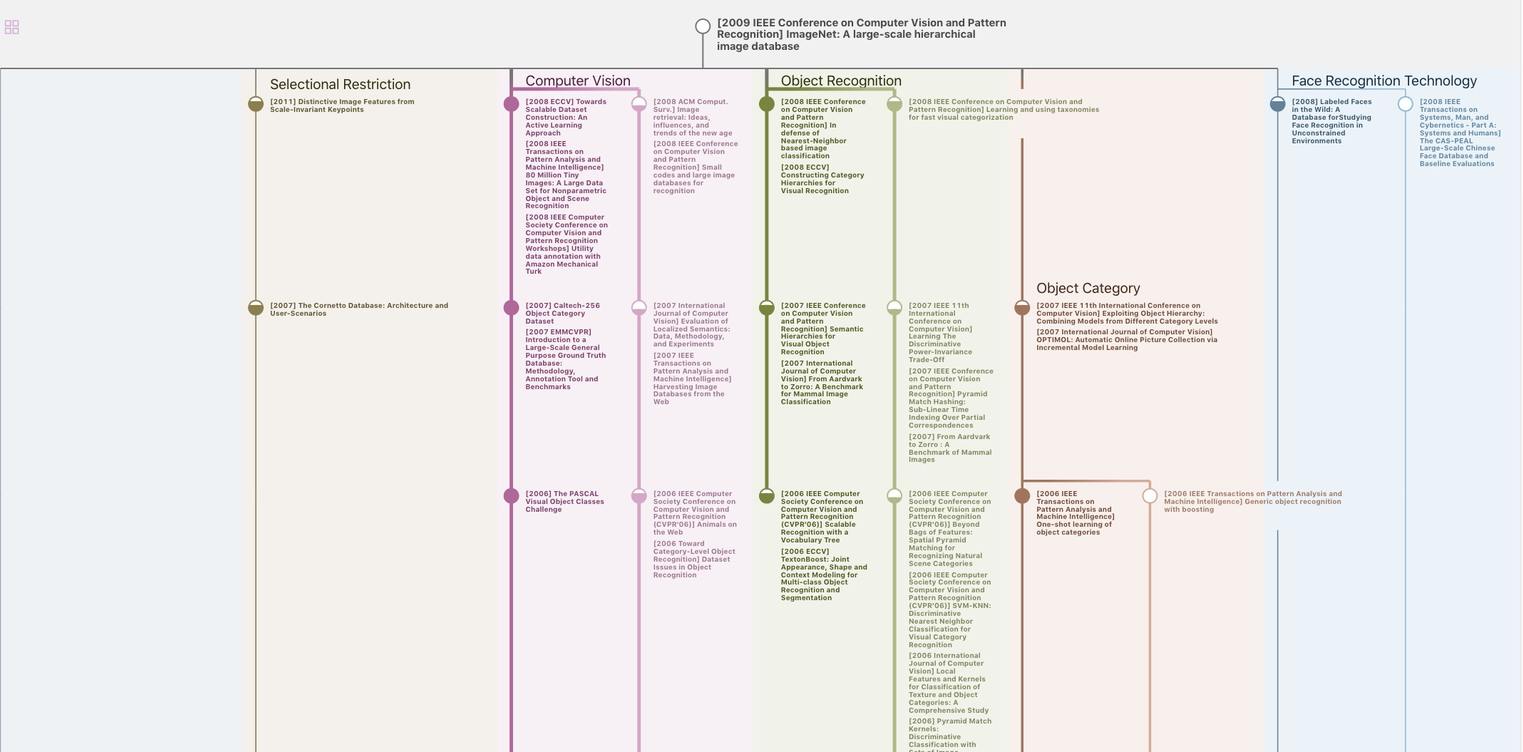
生成溯源树,研究论文发展脉络
Chat Paper
正在生成论文摘要