PILOT: An $\mathcal{O}(1/K)$-Convergent Approach for Policy Evaluation with Nonlinear Function Approximation
ICLR 2024(2024)
摘要
Learning an accurate value function for a given policy is a critical step in solving reinforcement learning (RL) problems. So far, however, the convergence speed and sample complexity performances of most existing policy evaluation algorithms remain unsatisfactory, particularly with non-linear function approximation. This challenge motivates us to develop a new path-integrated primal-dual stochastic gradient (PILOT) method, that is able to achieve a fast convergence speed for RL policy evaluation with nonlinear function approximation. To further alleviate the periodic full gradient evaluation requirement, we further propose an enhanced method with an adaptive-batch adjustment called PILOT$^+$. The main advantages of our methods include: i) PILOT allows the use of {\em{constant}} step sizes and achieves the $\mathcal{O}(1/K)$ convergence rate to first-order stationary points of non-convex policy evaluation problems; ii) PILOT is a generic {\em{single}}-timescale algorithm that is also applicable for solving a large class of non-convex strongly-concave minimax optimization problems; iii) By adaptively adjusting the batch size via historical stochastic gradient information, PILOT$^+$ is more sample-efficient empirically without loss of theoretical convergence rate. Our extensive numerical experiments verify our theoretical findings and showcase the high efficiency of the proposed PILOT and PILOT$^+$ algorithms compared with the state-of-the-art methods.
更多查看译文
关键词
min-max optimization,adaptive batch size,policy evaluation.
AI 理解论文
溯源树
样例
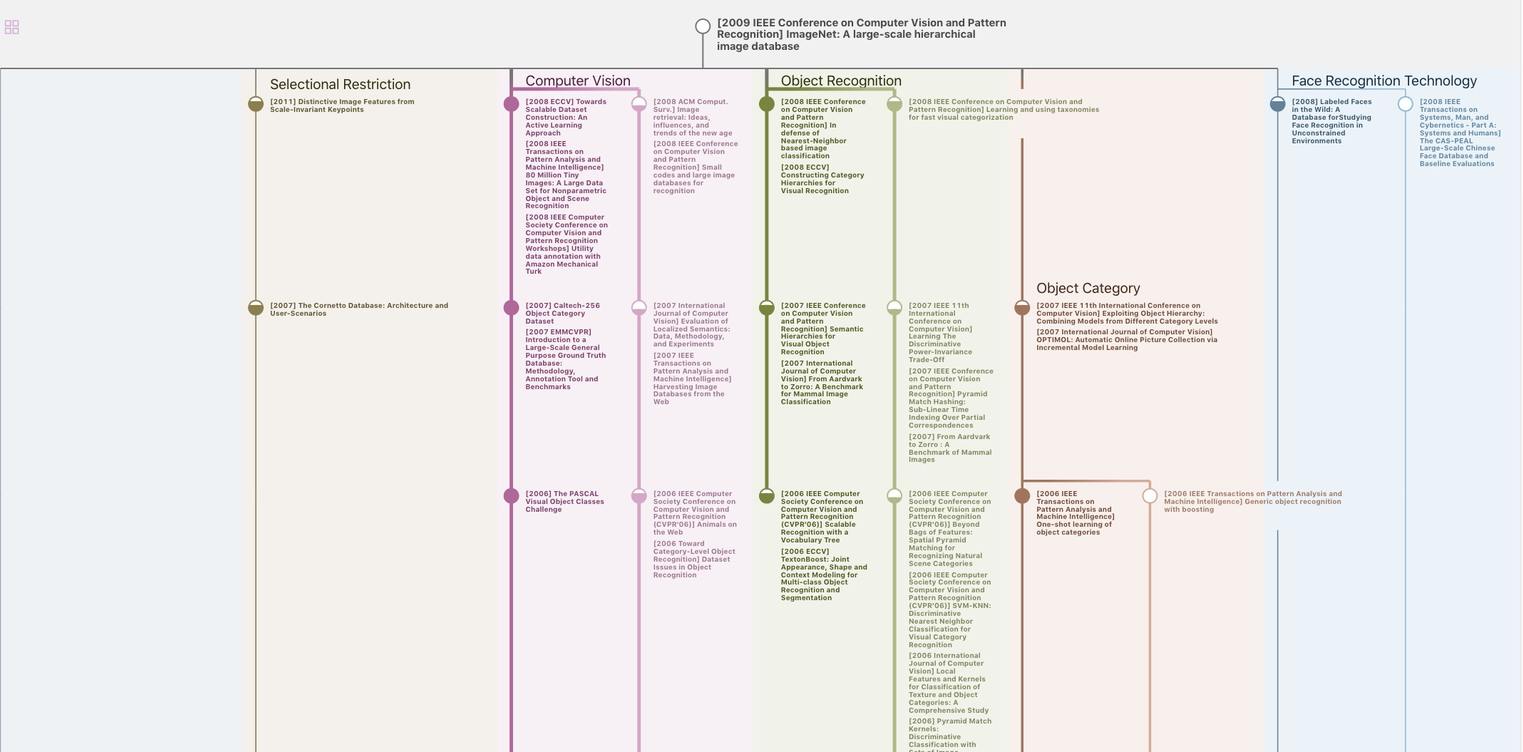
生成溯源树,研究论文发展脉络
Chat Paper
正在生成论文摘要