Convergence of Bayesian Bilevel Optimization
ICLR 2024(2024)
摘要
This paper presents the first theoretical guarantee for Bayesian bilevel optimization (BBO) that we term for the prevalent bilevel framework combining Bayesian optimization at the outer level to tune hyperparameters, including the inner-level stochastic gradient descent (SGD). We prove sublinear regret bounds suggesting simultaneous convergence of the inner-level model parameters and outer-level hyperparameters to optimal configurations for generalization capability. A pivotal, technical novelty in the proofs is modeling the excess risk of the SGD-trained parameters as evaluation noise during Bayesian optimization. Our theory implies the inner unit horizon, defined as the number of SGD iterations, shapes the convergence behavior of BBO. This suggests practical guidance on configuring the inner unit horizon to enhance training efficiency and model performance.
更多查看译文
关键词
Hyperparameter optimization,Bayesian optimization,Convergence rate,Bilevel optimization,Learning theory
AI 理解论文
溯源树
样例
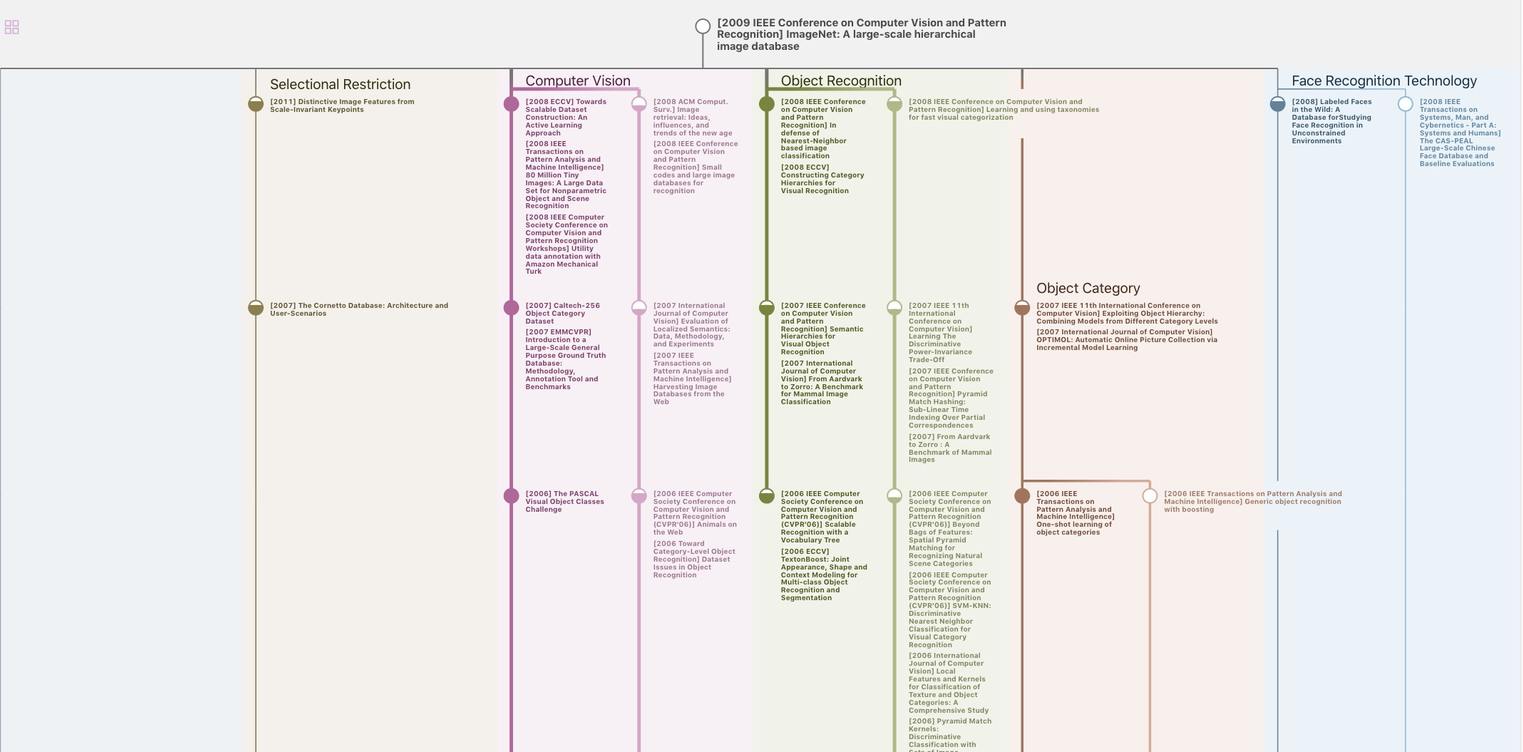
生成溯源树,研究论文发展脉络
Chat Paper
正在生成论文摘要