A Mutual Information Perspective on Federated Contrastive Learning
ICLR 2024(2024)
摘要
We extend SimCLR to the federated setting through the lens of multi-view mutual information maximization. In doing so, we uncover a connection between contrastive representation learning and user verification; by adding a user verification loss to each client's local SimCLR loss we recover a lower bound to the global multi-view mutual information. To accommodate for the case of when some labelled data are available at the clients, we extend our SimCLR variant to the federated semi-supervised setting. We see that a supervised SimCLR objective can be obtained with two changes: a) the contrastive loss is computed between datapoints that share the same label and b) we require an additional auxiliary head that predicts the correct labels from either of the two views. Along with the proposed SimCLR extensions, we also study how different sources of non-i.i.d.-ness can impact the performance of federated unsupervised learning through global mutual information maximization; we find that a global objective is beneficial for some sources of non-i.i.d.-ness but can be detrimental for others. We experimentally evaluate our proposed extensions in various tasks to validate our claims.
更多查看译文
关键词
federated learning,contrastive learning,self-supervised,semi-supervised,mutual information
AI 理解论文
溯源树
样例
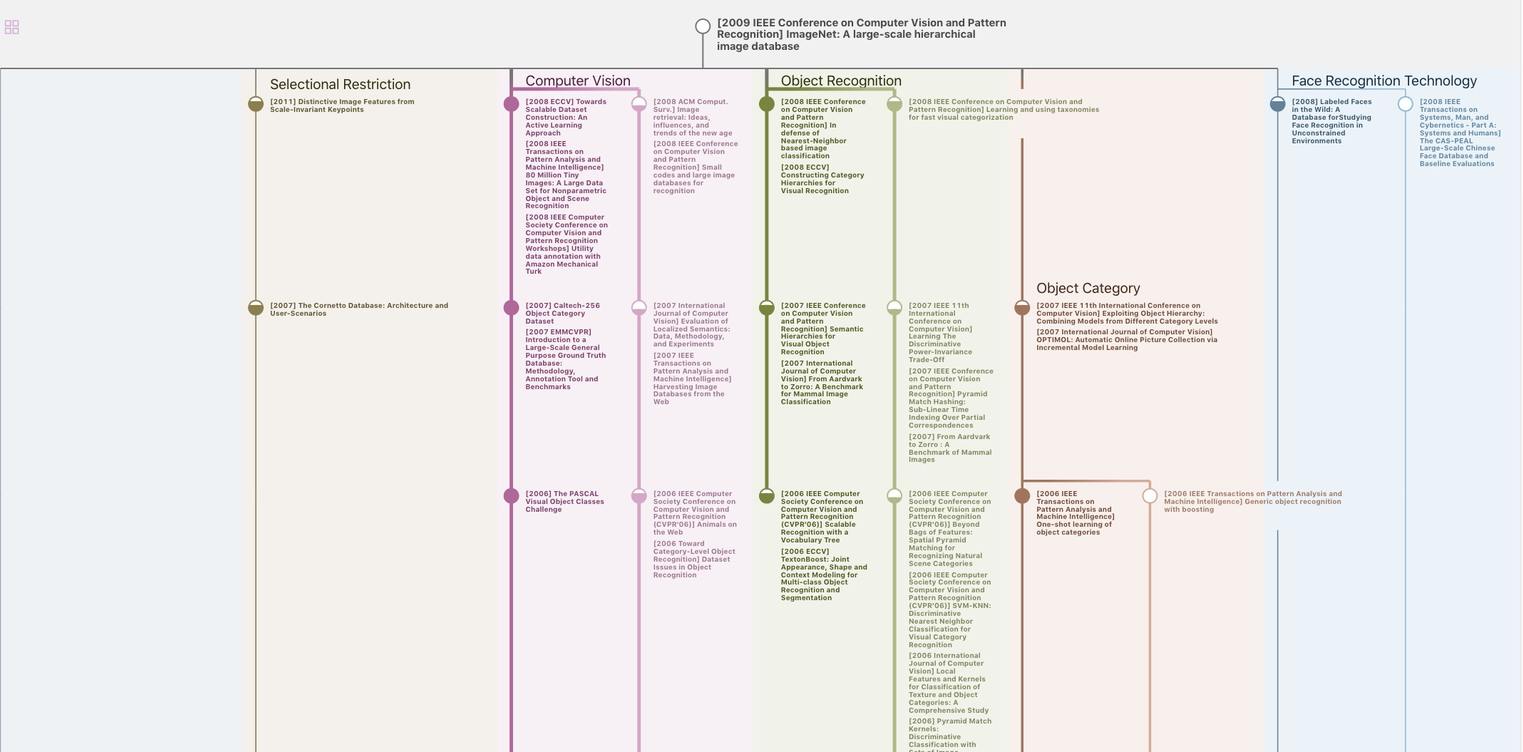
生成溯源树,研究论文发展脉络
Chat Paper
正在生成论文摘要