DreamFlow: High-Quality Text-to-3D Generation by Approximating Probability Flow
ICLR 2024(2024)
摘要
Recent progress in text-to-3D generation has been achieved through the
utilization of score distillation methods: they make use of the pre-trained
text-to-image (T2I) diffusion models by distilling via the diffusion model
training objective. However, such an approach inevitably results in the use of
random timesteps at each update, which increases the variance of the gradient
and ultimately prolongs the optimization process. In this paper, we propose to
enhance the text-to-3D optimization by leveraging the T2I diffusion prior in
the generative sampling process with a predetermined timestep schedule. To this
end, we interpret text-to3D optimization as a multi-view image-to-image
translation problem, and propose a solution by approximating the probability
flow. By leveraging the proposed novel optimization algorithm, we design
DreamFlow, a practical three-stage coarseto-fine text-to-3D optimization
framework that enables fast generation of highquality and high-resolution
(i.e., 1024x1024) 3D contents. For example, we demonstrate that DreamFlow is 5
times faster than the existing state-of-the-art text-to-3D method, while
producing more photorealistic 3D contents. Visit our project page
(https://kyungmnlee.github.io/dreamflow.github.io/) for visualizations.
更多查看译文
关键词
Text-to-3D generation,Diffusion model,Score-based Generative Model,Score Distillation Sampling
AI 理解论文
溯源树
样例
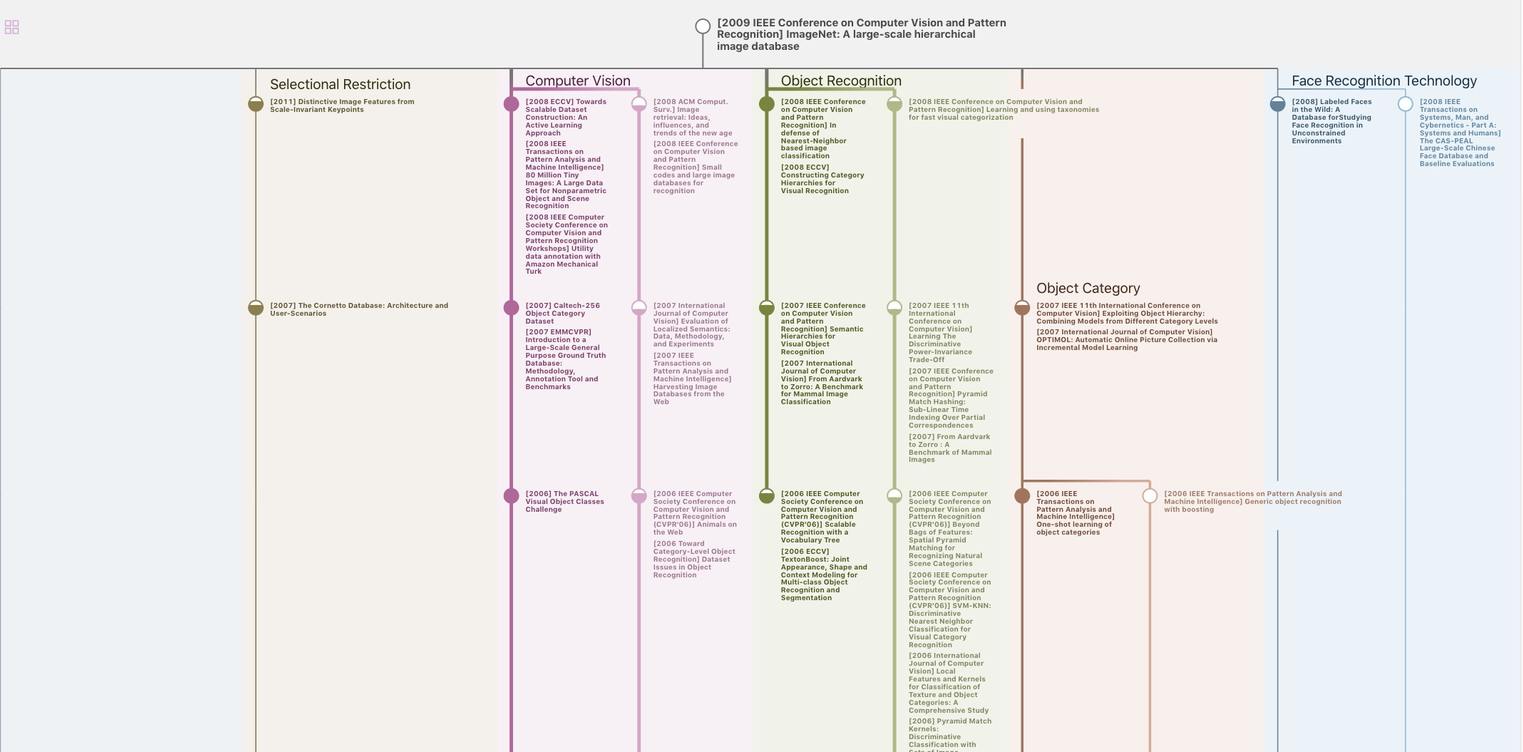
生成溯源树,研究论文发展脉络
Chat Paper
正在生成论文摘要