Learning Hierarchical Image Segmentation For Recognition and By Recognition
ICLR 2024(2024)
摘要
Image segmentation and recognition occur simultaneously, with recognition relying on the underlying segmentation to form a continuous visual grouping hierarchy. For example, the same object can be parsed into different part-to-whole structures, resulting in varying recognitions. Despite this, most prior works treated segmentation and recognition as separate tasks. In this paper, we aim to devise a learning framework that involves segmentation in the recognition process, utilizing hierarchical segmentation for recognition, which is learned by recognition. Specifically, we propose CAST, which realizes this concept through designs inspired by vision transformers, enabling concurrent segmentation and recognition with a single model. The core idea of CAST is to employ adaptive segment tokens that group the finest pixels into coarser segments, using the latest embedding to represent the entire image for recognition. Trained solely on image recognition objectives, CAST automatically discovers the hierarchy of segments. Our experiments demonstrate that CAST provides consistent hierarchical segmentation and recognition, which is impossible with state-of-the-art segmentation methods such as SAM. Additionally, CAST offers several advantages over the standard ViT, including improved semantic segmentation, computational efficiency, and object-centric attention.
更多查看译文
关键词
segmentation in the loop for recognition,hierarchical segmentation,part-to-whole recognition,vision transformer
AI 理解论文
溯源树
样例
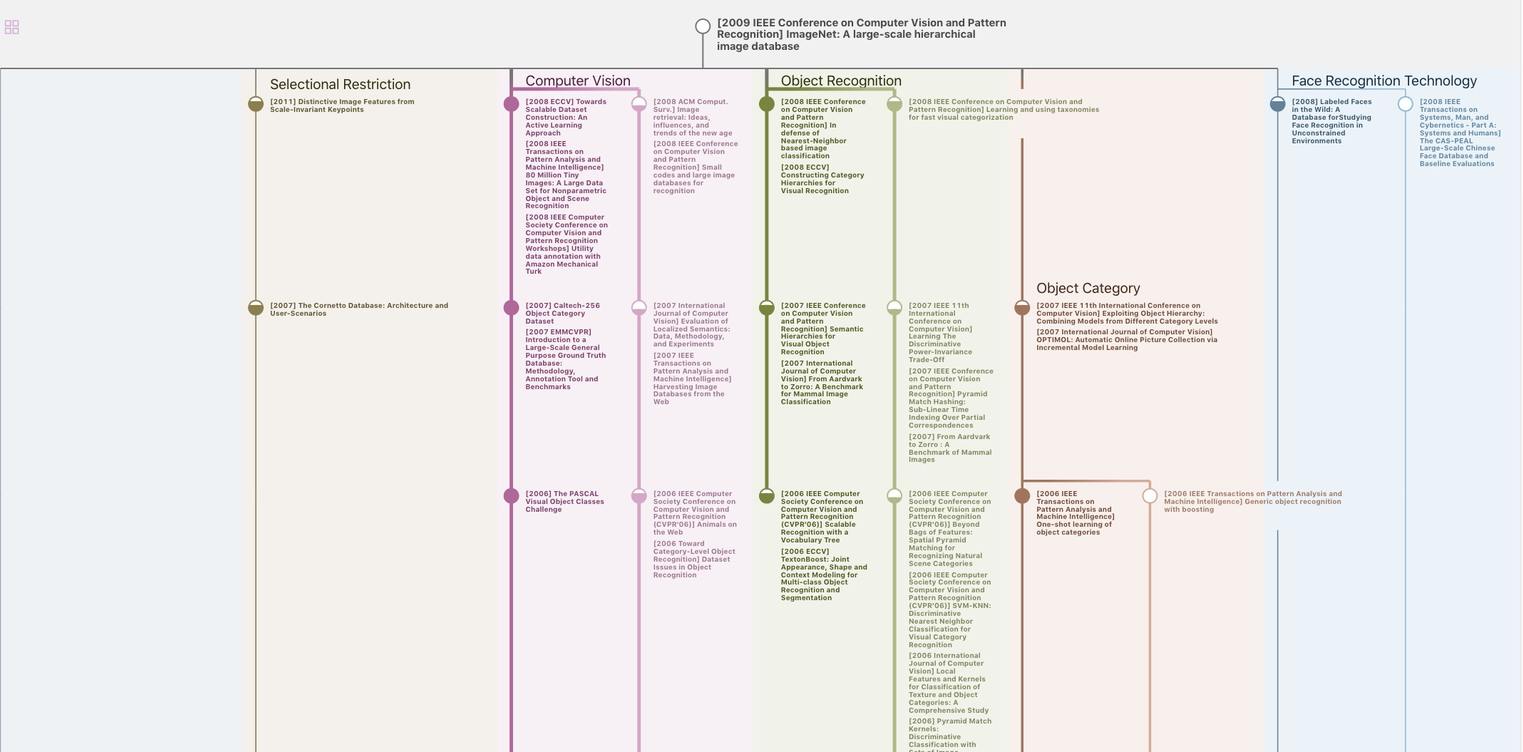
生成溯源树,研究论文发展脉络
Chat Paper
正在生成论文摘要