ModernTCN: A Modern Pure Convolution Structure for General Time Series Analysis
ICLR 2024(2024)
摘要
Recently, Transformer-based and MLP-based models have emerged rapidly and won dominance in time series analysis. In contrast, convolution is losing steam in time series tasks nowadays for inferior performance. This paper studies the open question of how to better use convolution in time series analysis and makes efforts to bring convolution back to the arena of time series analysis. To this end, we modernize the traditional TCN and conduct time series related modifications to make it more suitable for time series tasks. As the outcome, we propose ModernTCN and successfully solve this open question through a seldom-explored way in time series community. As a pure convolution structure, ModernTCN still achieves the consistent state-of-the-art performance on five mainstream time series analysis tasks (long-term and short-term forecasting, imputation, classification and anomaly detection) while maintaining the efficiency advantage of convolution-based models, therefore providing a better balance of efficiency and performance than state-of-the-art Transformer-based and MLP-based models. Our study further reveals that, compared with previous convolution-based models, our ModernTCN has much larger effective receptive fields (ERFs), therefore can better unleash the potential of convolution in time series analysis. The code will be publicly available.
更多查看译文
关键词
Time Series Analysis,Deep Learning
AI 理解论文
溯源树
样例
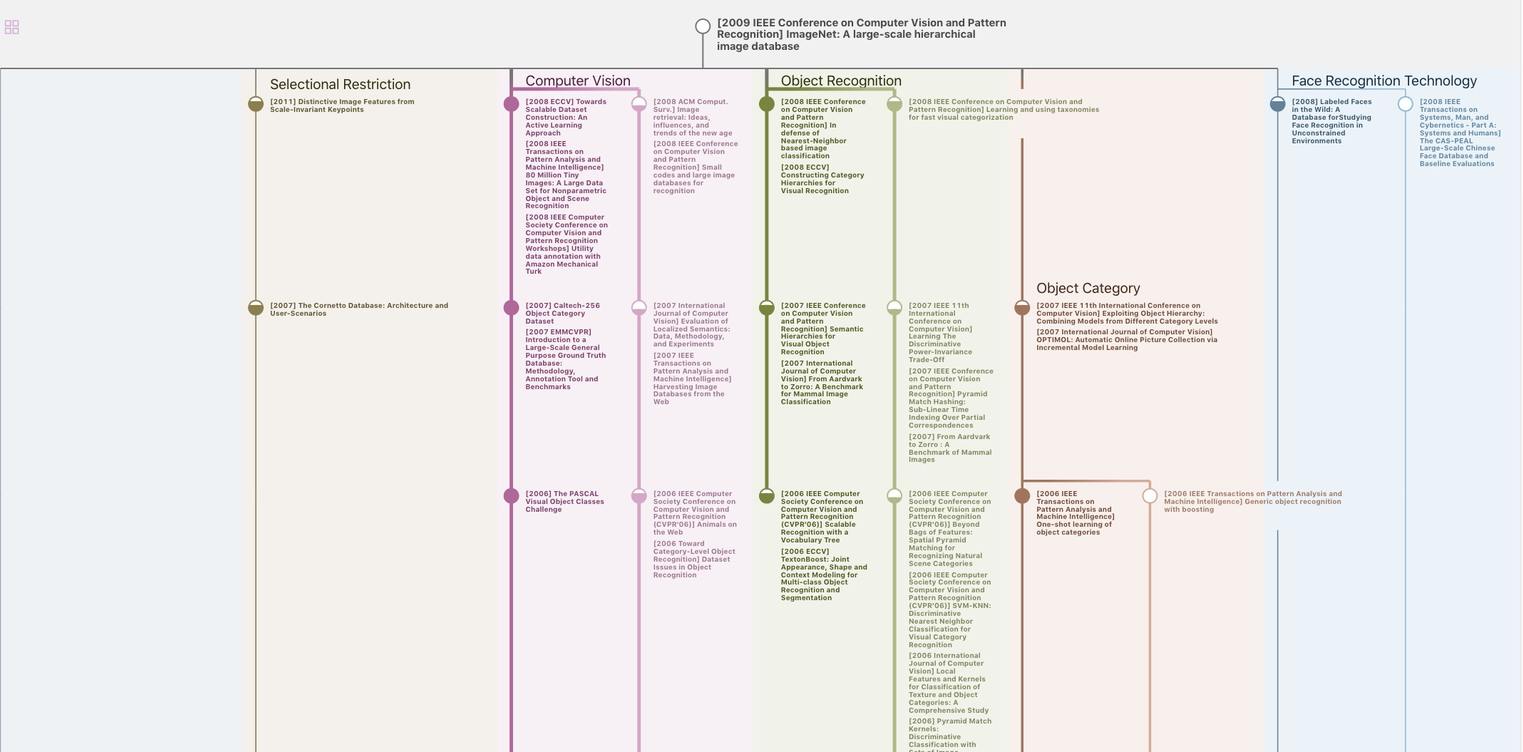
生成溯源树,研究论文发展脉络
Chat Paper
正在生成论文摘要