A Socio-Technical Approach to Trustworthy Semantic Biomedical Content Generation and Sharing
Information Sciences(2024)
摘要
The rapid growth of online biomedical content has presented a notable challenge in delivering timely and precise semantic annotations. Semantic annotations play a crucial role in contextually indexing data, thereby enhancing search accuracy. This intricate process involves the utilization of multiple coded ontologies, requiring extensive technical expertise and domain knowledge. While automated ontologies face limitations in balancing accuracy and speed, expert knowledge generation is also scarce and expensive. In response to these conflicting challenges, we propose a socio-technical content generation and sharing approach named ‘Semantically,’ which actively involves biomedical experts and scientists. Additionally, ‘Semantically’ leverages schema.org for incorporating additional semantic tags to enhance search engine performance. The outcome is high-quality, machine-understandable content that not only facilitates fast and accurate searches but also instills trust due to the collaborative nature of the annotation process. ‘Semantically’ generated biomedical content was evaluated in two scenarios: (1) search based solely on initial-level annotations and (2) search incorporating additional expert-recommended annotations. ‘Semantically’ enhances the user search experience when compared to benchmark data. In the first scenario, the unigram to 5-grams strategy efficiently recognizes biomedical terms, resulting in high precision, recall, F1, and accuracy scores, all around 0.9. ‘Semantically’ code is openly accessible at https://github.com/bukharilab/Semantically.
更多查看译文
关键词
Biomedical content,Semantic annotations,Annotation recommendation,Knowledge sharing,Socio-Technical content generation,Trust
AI 理解论文
溯源树
样例
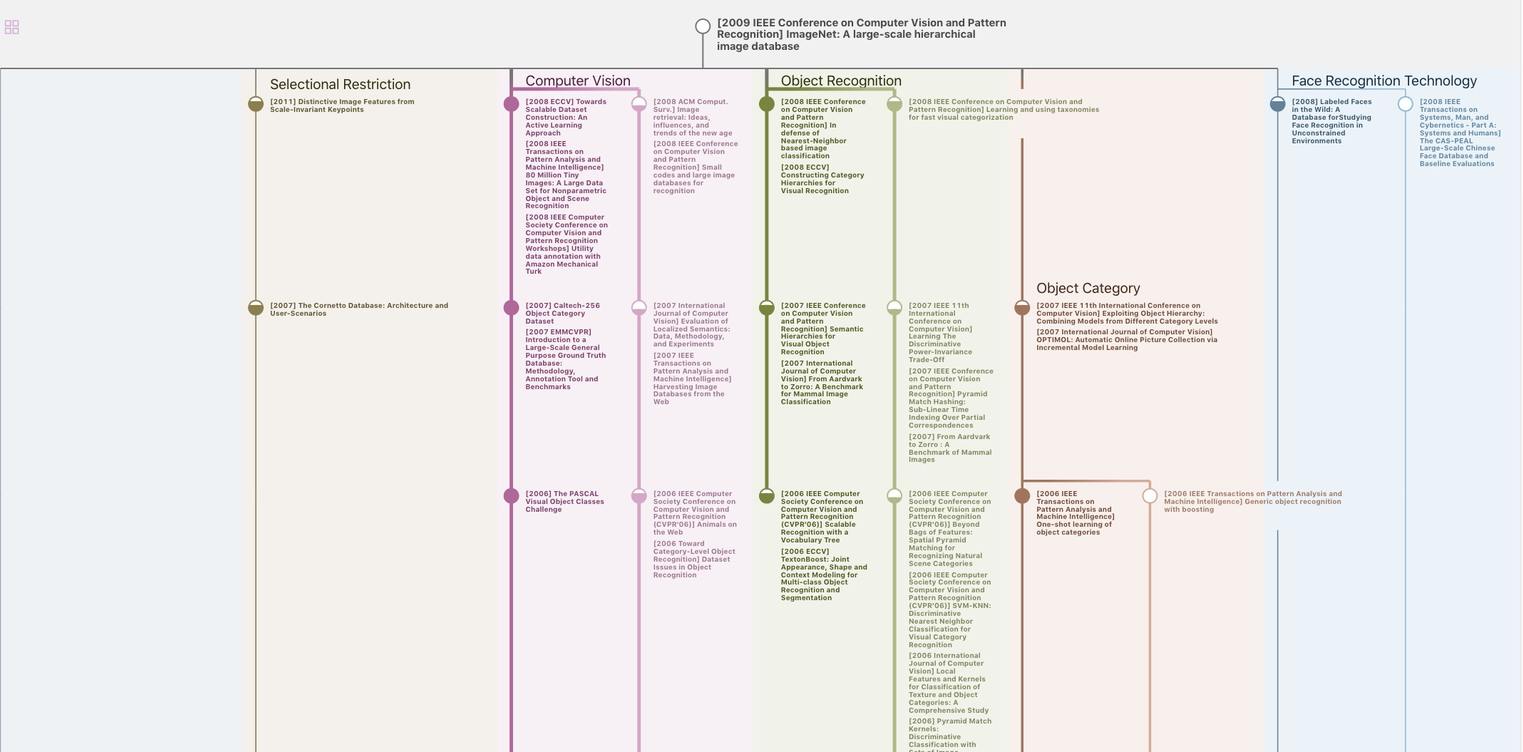
生成溯源树,研究论文发展脉络
Chat Paper
正在生成论文摘要