Overlapping Community Detection Based on Weak Equiconcept
IEEE ACCESS(2024)
摘要
Community discovery refers to the process of searching for clusters in a network that are sparsely connected to other nodes and formed dense connections internally. In many real networks, some communities often overlap with each other, meaning that a node may belong to multiple communities simultaneously. Revealing these (overlapping) community structures is an important issue in complex network analysis, as it helps to better analyze the characteristics and organizational structure of the network. Community expansion methods are very important in the study of community detection problems. However, one of the key issues in developing effective community expansion methods is that the position of seed nodes greatly affects the performance of these algorithms, resulting in low robustness of these algorithms. Meanwhile, it is also difficult for them to provide high-quality results for community detection task. To solve the above problem, this paper proposes a seed selection method based on the weak equiconcepts in a network formal context, which integrates the attribute information of nodes during random walk to detect overlapping communities. Specifically, the weak equiconcepts are constructed by establishing a network formal context to obtain seed sets, and an improved PageRank clustering algorithm is used to expand these seed sets to better reveal the overlapping community structure in the network. Experiments show that seed selection is helpful to improving the performance of overlapping community detection algorithms.
更多查看译文
关键词
Clustering algorithms,Complex networks,Task analysis,Optimization,Loss measurement,Knowledge discovery,Information entropy,Detection algorithms,Overlapping community discovery,network formal context,weak equiconcept,random walk
AI 理解论文
溯源树
样例
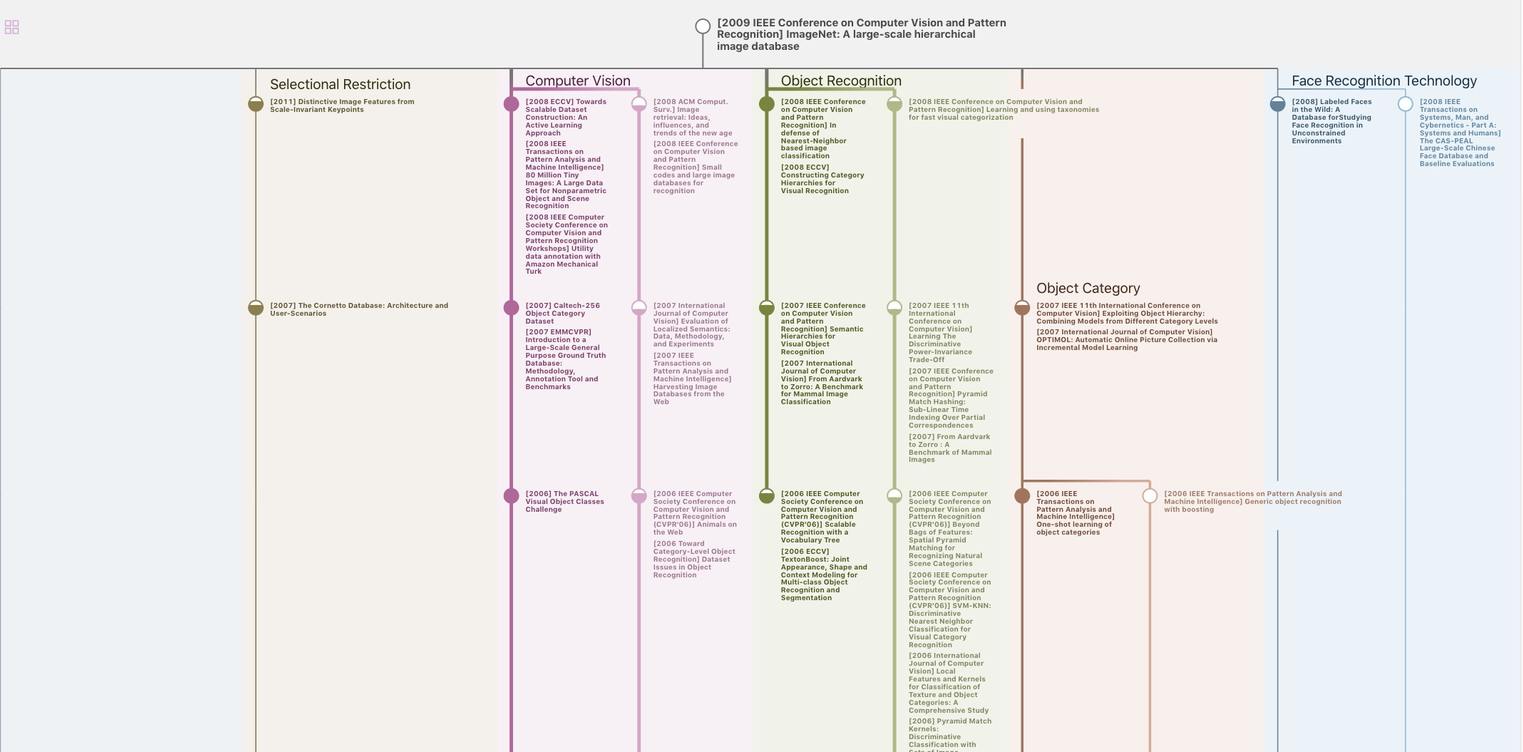
生成溯源树,研究论文发展脉络
Chat Paper
正在生成论文摘要