Posterior contraction rates for constrained deep Gaussian processes in density estimation and classification
COMMUNICATIONS IN STATISTICS-THEORY AND METHODS(2024)
摘要
We provide posterior contraction rates for constrained deep Gaussian processes in non parametric density estimation and classification. The constraints are in the form of bounds on the values and on the derivatives of the Gaussian processes in the layers of the compositional structure. The contraction rates are first given in a general framework, in terms of a new concentration function that we introduce and that takes the constraints into account. Then, the general framework is applied to integrated Brownian motions, Riemann-Liouville processes, and Matern processes. In each of these examples, we can recover existing rates, both when the compositional structure is dense and sparse.
更多查看译文
关键词
Gaussian priors,deep Gaussian priors[ Bayesian inference,non parametric density estimation,classification,posterior contraction,smoothness classes,Matern covariance functions
AI 理解论文
溯源树
样例
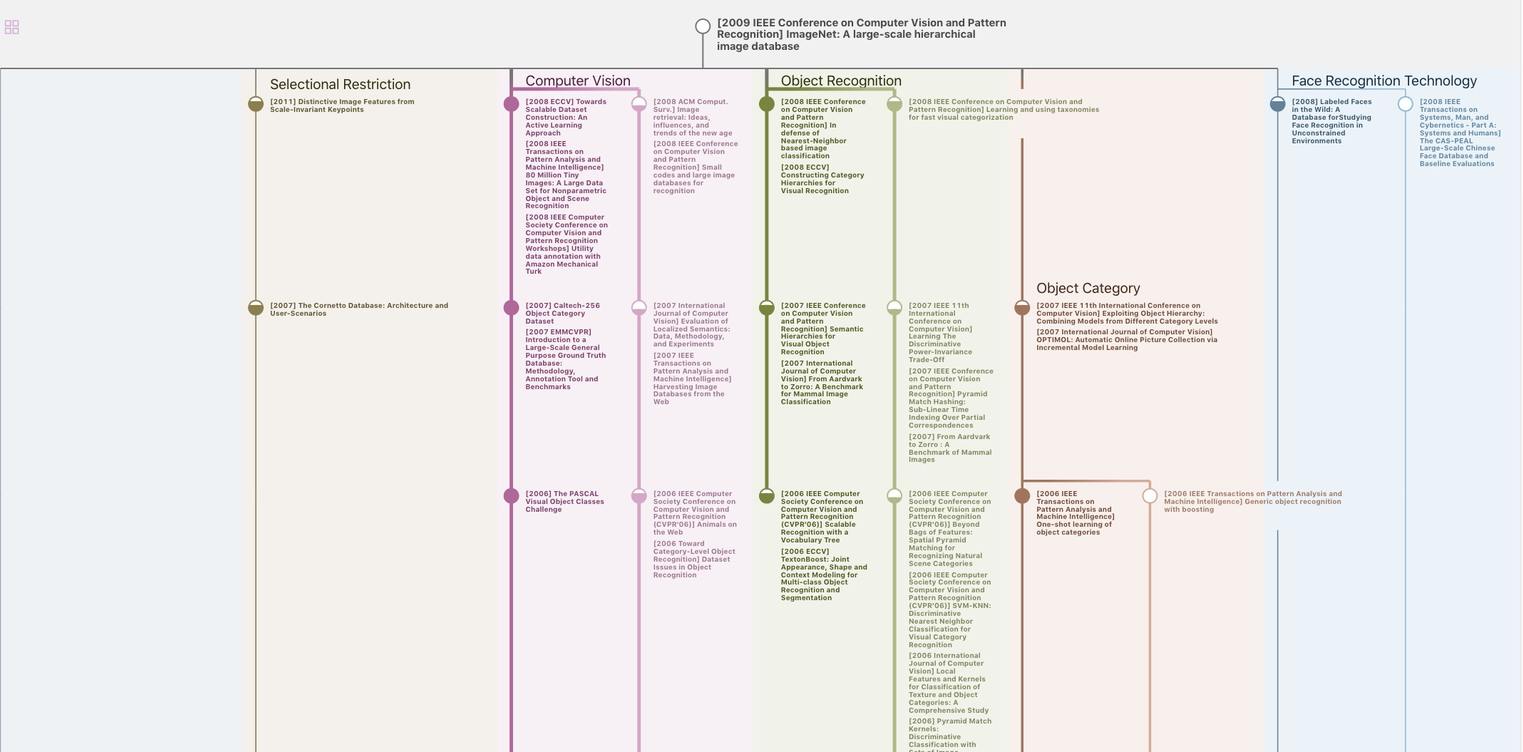
生成溯源树,研究论文发展脉络
Chat Paper
正在生成论文摘要