Explainable AI for binary and multi-class classification of leukemia using a modified transfer learning ensemble model
INTERNATIONAL JOURNAL ON SMART SENSING AND INTELLIGENT SYSTEMS(2024)
摘要
In leukemia diagnosis, automating the process of decision-making can reduce the impact of individual pathologists' expertise. While deep learning models have demonstrated promise in disease diagnosis, combining them can yield superior results. This research introduces an ensemble model that merges two pre-trained deep learning models, namely, VGG-16 and Inception, using transfer learning. It aims to accurately classify leukemia subtypes using real and standard dataset images, focusing on interpretability. Therefore, the use of Local Interpretable Model-Agnostic Explanations (LIME) is employed to achieve interpretability. The ensemble model achieves an accuracy of 83.33% in binary classification, outperforming individual models. In multi-class classification, VGG-16 and Inception reach accuracies of 83.335% and 93.33%, respectively, while the ensemble model reaches an accuracy of 100%.
更多查看译文
关键词
leukemia,classification,ensemble,VGG-16,Inception,SHAP,LIME,GradCAM
AI 理解论文
溯源树
样例
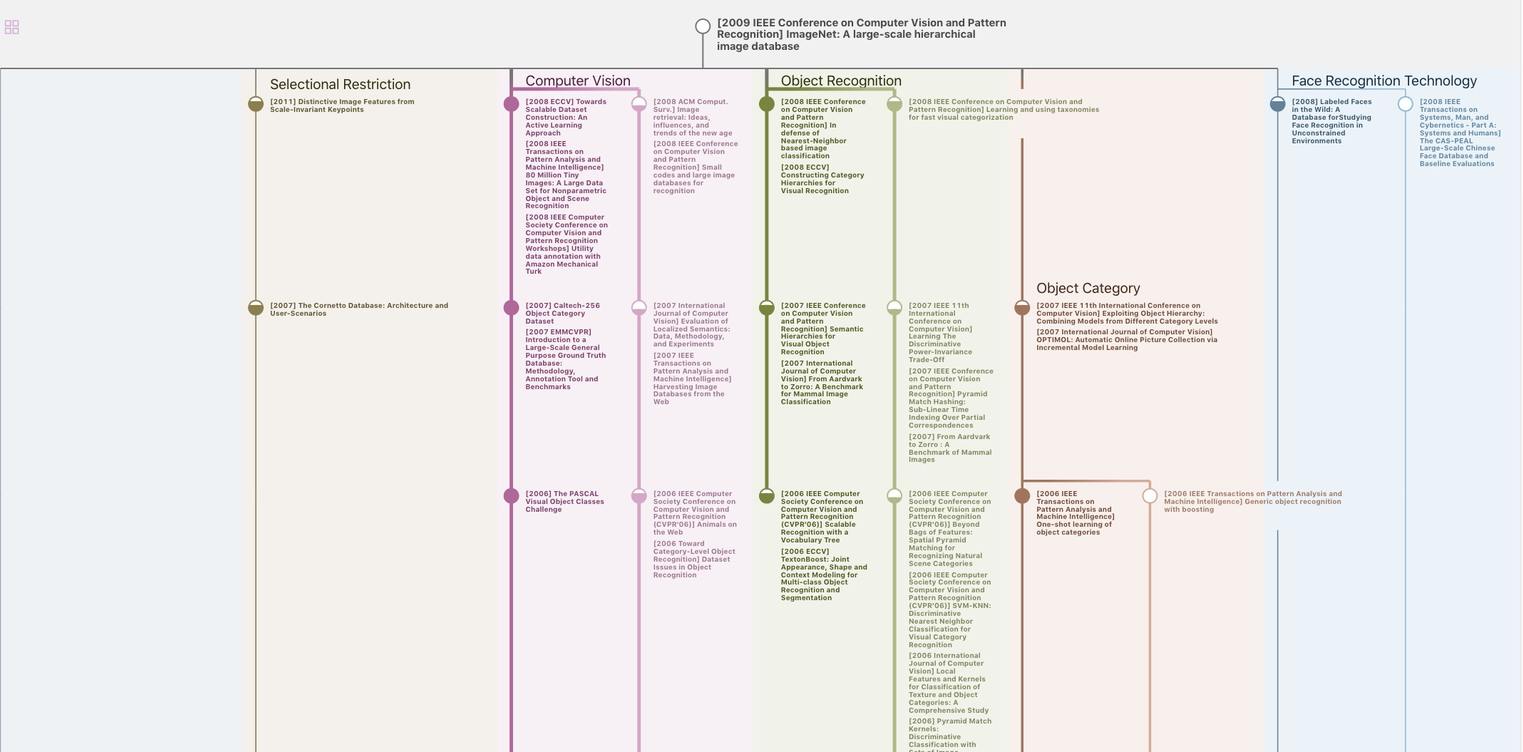
生成溯源树,研究论文发展脉络
Chat Paper
正在生成论文摘要