SPGC: Shape-Prior-Based Generated Content Data Augmentation for Remote Sensing Object Detection
IEEE TRANSACTIONS ON GEOSCIENCE AND REMOTE SENSING(2024)
摘要
While deep learning-based methods have made significant strides in remote sensing applications, the scarcity and inadequate quality of remote sensing images tend to curtail the improvement of follow-up research such as remote sensing object detection. However, the human visual system is able to quickly grasp the features of an unseen object given only a few examples, which is considered to be related to a strong shape bias. Inspired by how human toddlers learn shapes and the process of recognizing objects by shape, this article proposes a novel method known as shape-prior-based generated content (SPGC) data augmentation to overcome these challenges. Specifically, our method includes two main steps: shape data generation and stylization. Initially, the method begins with generating shape data regardless of training data availability. Next, we enhance the robustness of the generated shape data through stylization, forming a robust shape dataset (SD). Stylization is further bifurcated into two scenarios: when training data are unseen, self-stylization is employed where the shape data simultaneously serves as content and style data, resulting in significant performance improvements. When training data are accessible, data-specific stylization is applied, with the shape data as content and training data as style, leading to more substantial enhancements than self-stylization. Experimental results on mainstream remote sensing object detection datasets including NWPU VHR-10, DIOR, and FAIR1M demonstrate that our method significantly improves performance and underscores its effectiveness.
更多查看译文
关键词
Data augmentation,remote sensing object detection,shape-prior guidance,stylization
AI 理解论文
溯源树
样例
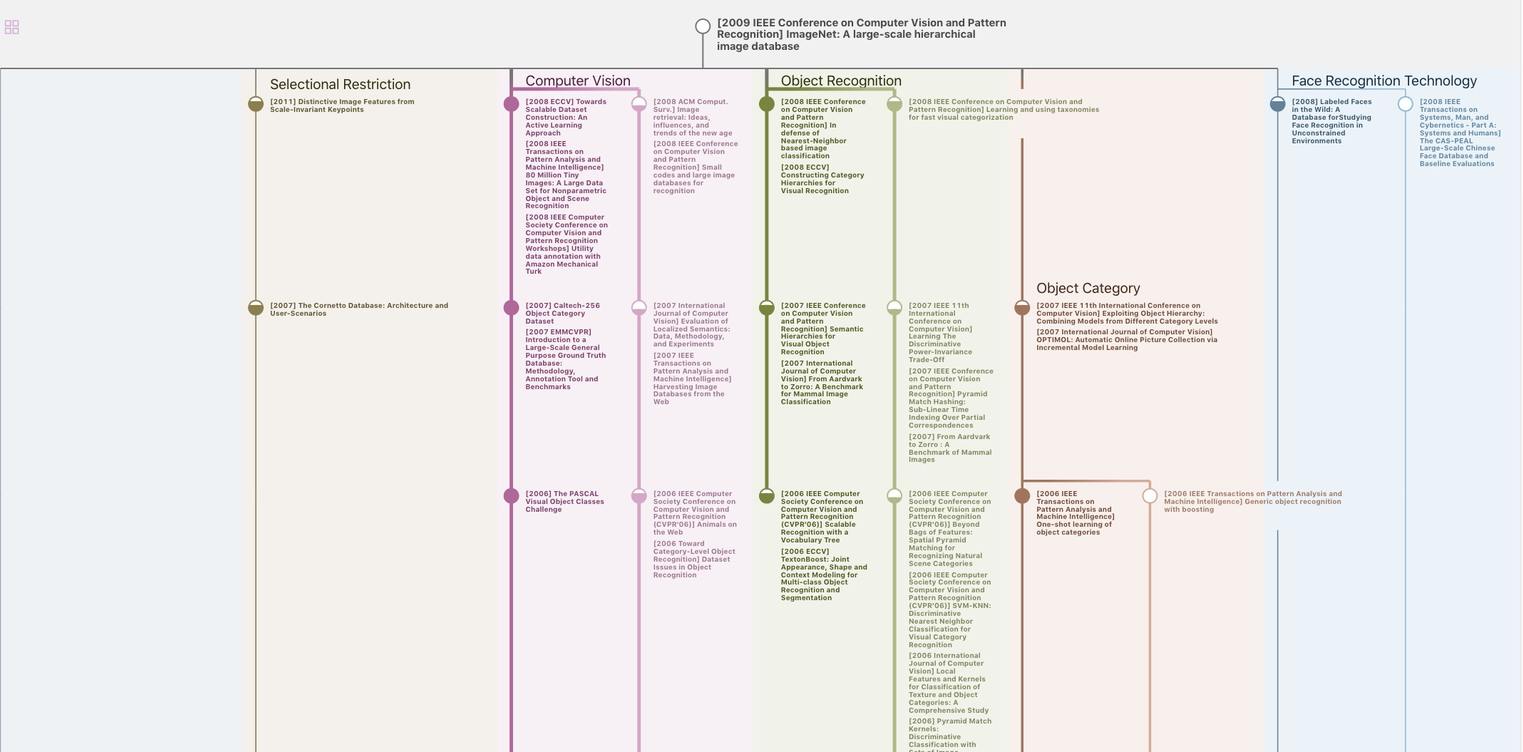
生成溯源树,研究论文发展脉络
Chat Paper
正在生成论文摘要