Proxy-RLHF: Decoupling Generation and Alignment in Large Language Model with Proxy
arxiv(2024)
摘要
Reinforcement Learning from Human Feedback (RLHF) is the prevailing approach to ensure Large Language Models (LLMs) align with human values. However, existing RLHF methods require a high computational cost, one main reason being that RLHF assigns both the generation and alignment tasks to the LLM simultaneously. In this paper, we introduce Proxy-RLHF, which decouples the generation and alignment processes of LLMs, achieving alignment with human values at a much lower computational cost. We start with a novel Markov Decision Process (MDP) designed for the alignment process and employ Reinforcement Learning (RL) to train a streamlined proxy model that oversees the token generation of the LLM, without altering the LLM itself. Experiments show that our method achieves a comparable level of alignment with only 1\% of the training parameters of other methods.
更多查看译文
AI 理解论文
溯源树
样例
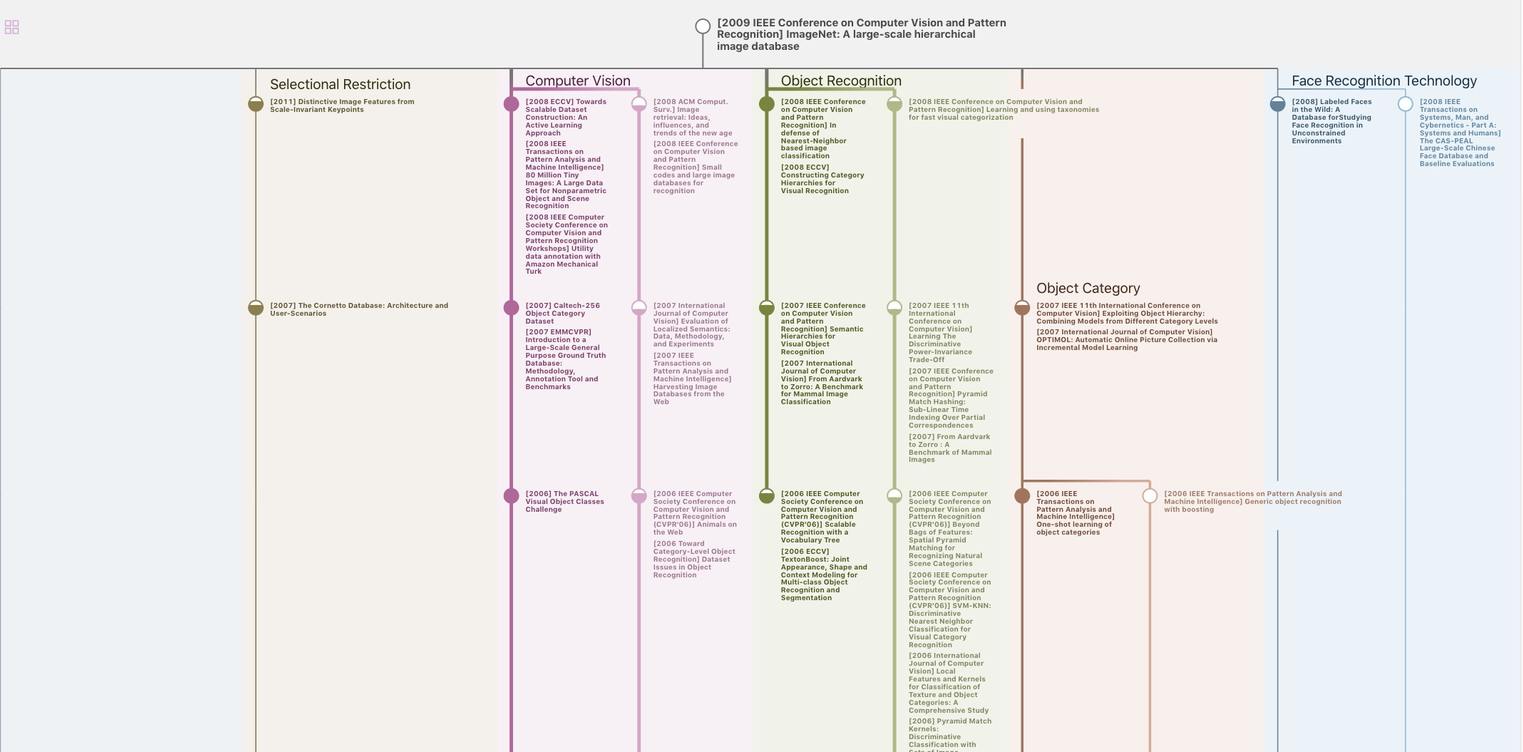
生成溯源树,研究论文发展脉络
Chat Paper
正在生成论文摘要