Mastering Memory Tasks with World Models
ICLR 2024(2024)
摘要
Current model-based reinforcement learning (MBRL) agents struggle with
long-term dependencies. This limits their ability to effectively solve tasks
involving extended time gaps between actions and outcomes, or tasks demanding
the recalling of distant observations to inform current actions. To improve
temporal coherence, we integrate a new family of state space models (SSMs) in
world models of MBRL agents to present a new method, Recall to Imagine (R2I).
This integration aims to enhance both long-term memory and long-horizon credit
assignment. Through a diverse set of illustrative tasks, we systematically
demonstrate that R2I not only establishes a new state-of-the-art for
challenging memory and credit assignment RL tasks, such as BSuite and POPGym,
but also showcases superhuman performance in the complex memory domain of
Memory Maze. At the same time, it upholds comparable performance in classic RL
tasks, such as Atari and DMC, suggesting the generality of our method. We also
show that R2I is faster than the state-of-the-art MBRL method, DreamerV3,
resulting in faster wall-time convergence.
更多查看译文
关键词
model-based reinforcement learning,state space models,memory in reinforcement learning
AI 理解论文
溯源树
样例
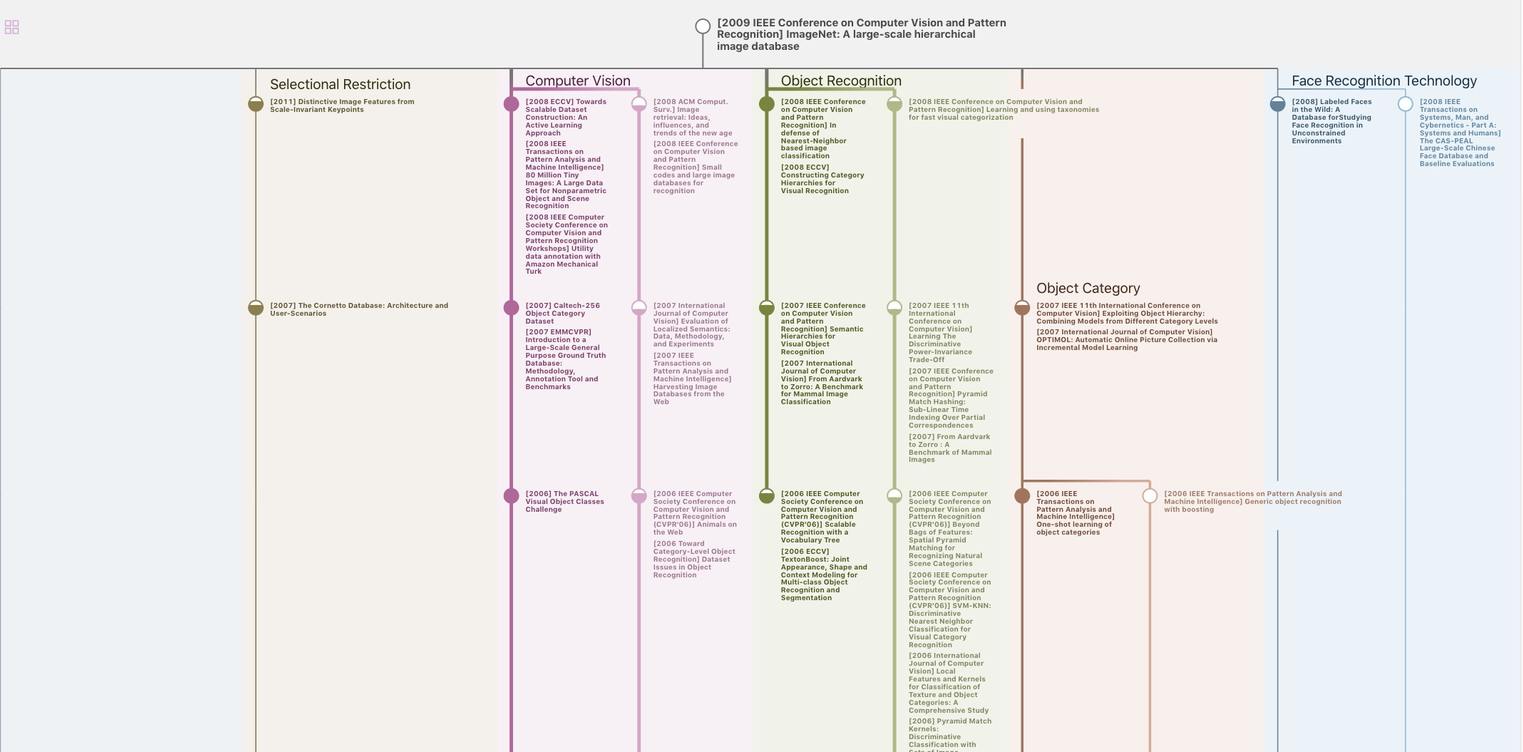
生成溯源树,研究论文发展脉络
Chat Paper
正在生成论文摘要