GNN-VPA: A Variance-Preserving Aggregation Strategy for Graph Neural Networks
arxiv(2024)
摘要
Graph neural networks (GNNs), and especially message-passing neural networks,
excel in various domains such as physics, drug discovery, and molecular
modeling. The expressivity of GNNs with respect to their ability to
discriminate non-isomorphic graphs critically depends on the functions employed
for message aggregation and graph-level readout. By applying signal propagation
theory, we propose a variance-preserving aggregation function (VPA) that
maintains expressivity, but yields improved forward and backward dynamics.
Experiments demonstrate that VPA leads to increased predictive performance for
popular GNN architectures as well as improved learning dynamics. Our results
could pave the way towards normalizer-free or self-normalizing GNNs.
更多查看译文
AI 理解论文
溯源树
样例
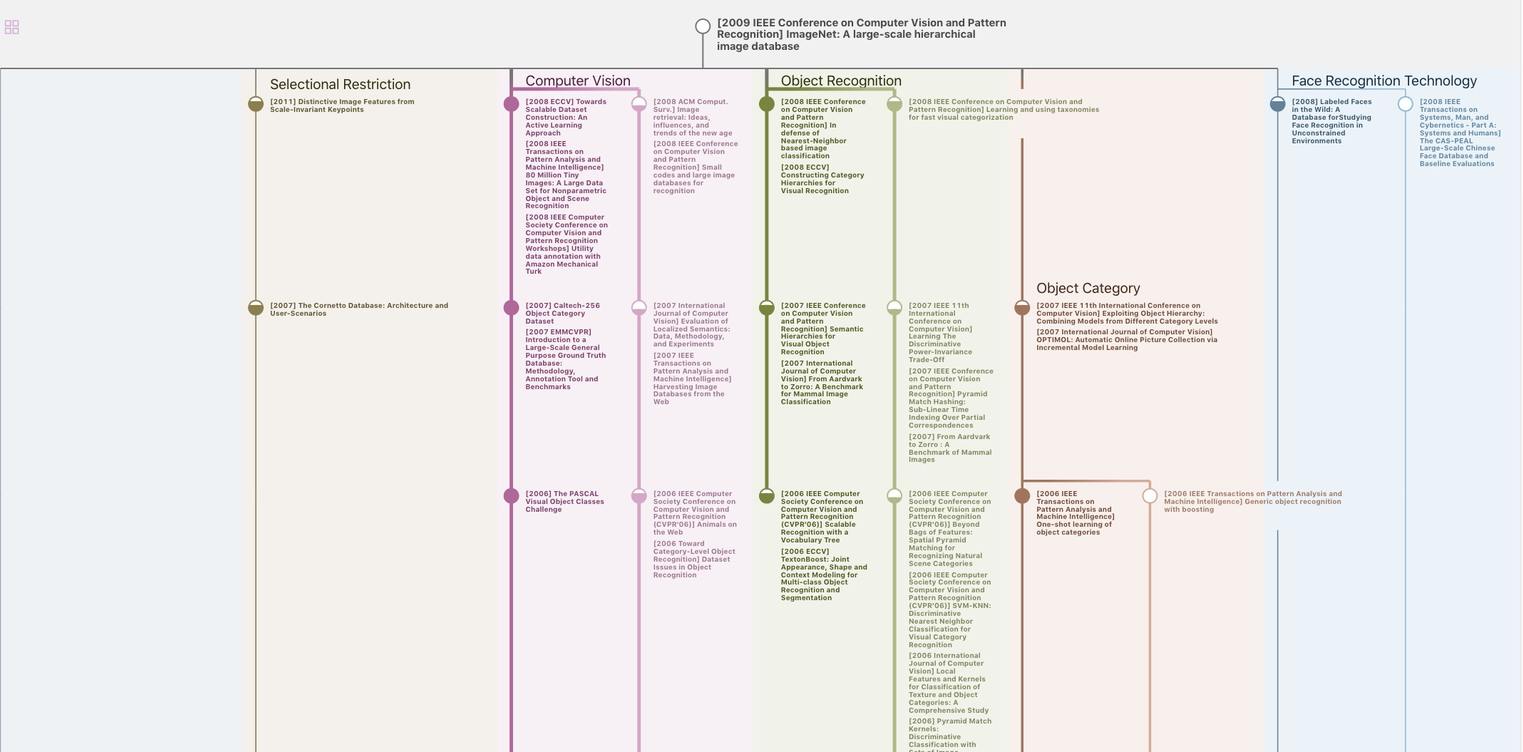
生成溯源树,研究论文发展脉络
Chat Paper
正在生成论文摘要