Online Learning with Unknown Constraints
arxiv(2024)
摘要
We consider the problem of online learning where the sequence of actions
played by the learner must adhere to an unknown safety constraint at every
round. The goal is to minimize regret with respect to the best safe action in
hindsight while simultaneously satisfying the safety constraint with high
probability on each round. We provide a general meta-algorithm that leverages
an online regression oracle to estimate the unknown safety constraint, and
converts the predictions of an online learning oracle to predictions that
adhere to the unknown safety constraint. On the theoretical side, our
algorithm's regret can be bounded by the regret of the online regression and
online learning oracles, the eluder dimension of the model class containing the
unknown safety constraint, and a novel complexity measure that captures the
difficulty of safe learning. We complement our result with an asymptotic lower
bound that shows that the aforementioned complexity measure is necessary. When
the constraints are linear, we instantiate our result to provide a concrete
algorithm with √(T) regret using a scaling transformation that balances
optimistic exploration with pessimistic constraint satisfaction.
更多查看译文
AI 理解论文
溯源树
样例
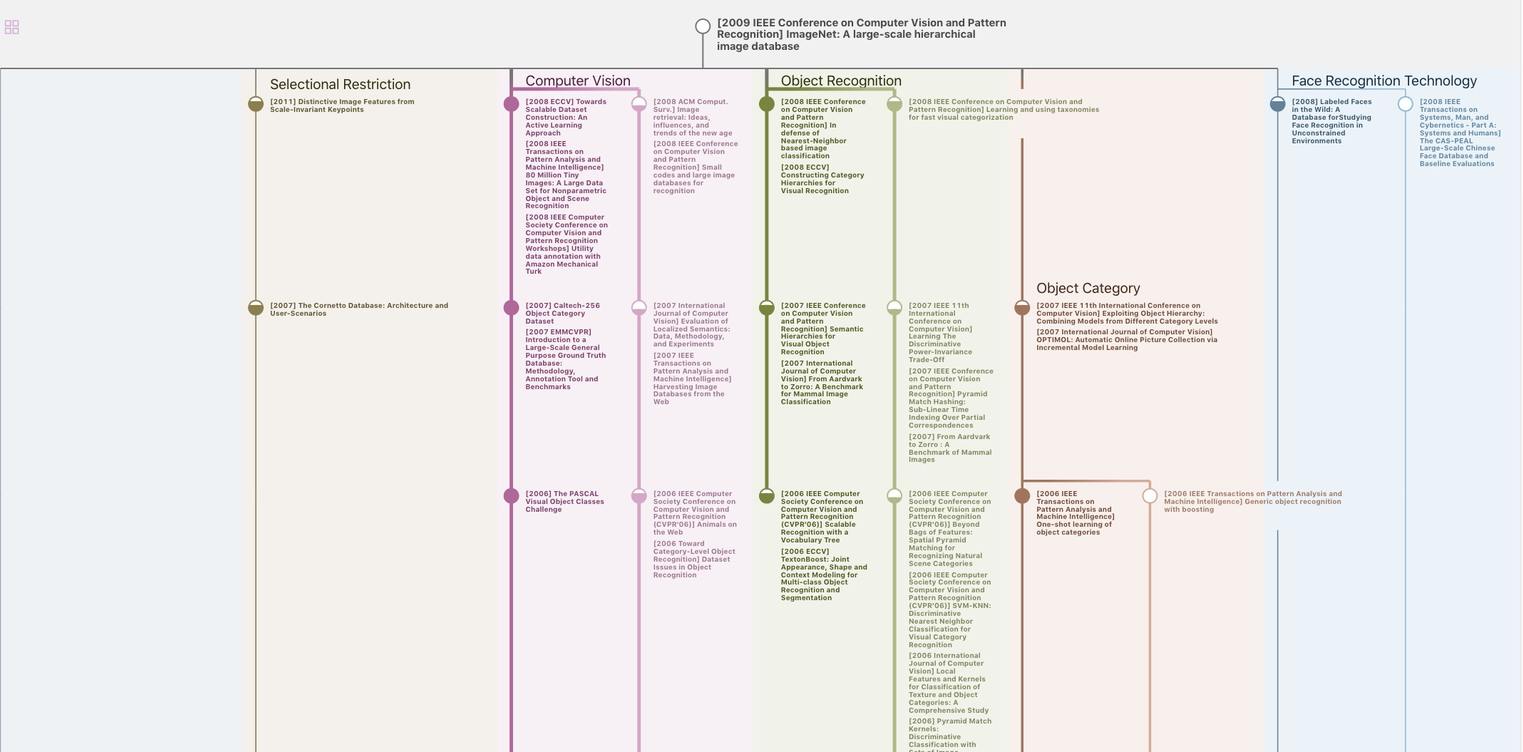
生成溯源树,研究论文发展脉络
Chat Paper
正在生成论文摘要