SegCLIP: Patch Aggregation with Learnable Centers for Open-Vocabulary Semantic Segmentation
arxiv(2022)
摘要
Recently, the contrastive language-image pre-training, e.g., CLIP, has demonstrated promising results on various downstream tasks. The pre-trained model can capture enriched visual concepts for images by learning from a large scale of text-image data. However, transferring the learned visual knowledge to open-vocabulary semantic segmentation is still under-explored. In this paper, we propose a CLIP-based model named SegCLIP for the topic of open-vocabulary segmentation in an annotation-free manner. The SegCLIP achieves segmentation based on ViT and the main idea is to gather patches with learnable centers to semantic regions through training on text-image pairs. The gathering operation can dynamically capture the semantic groups, which can be used to generate the final segmentation results. We further propose a reconstruction loss on masked patches and a superpixel-based KL loss with pseudo-labels to enhance the visual representation. Experimental results show that our model achieves comparable or superior segmentation accuracy on the PASCAL VOC 2012 (+1.4% mIoU), PASCAL Context (+2.4% mIoU), and COCO (+5.6% mIoU) compared with baselines. We release the code at https://github.com/ArrowLuo/SegCLIP.
更多查看译文
关键词
segmentation,patch aggregation,learnable centers,semantic,open-vocabulary
AI 理解论文
溯源树
样例
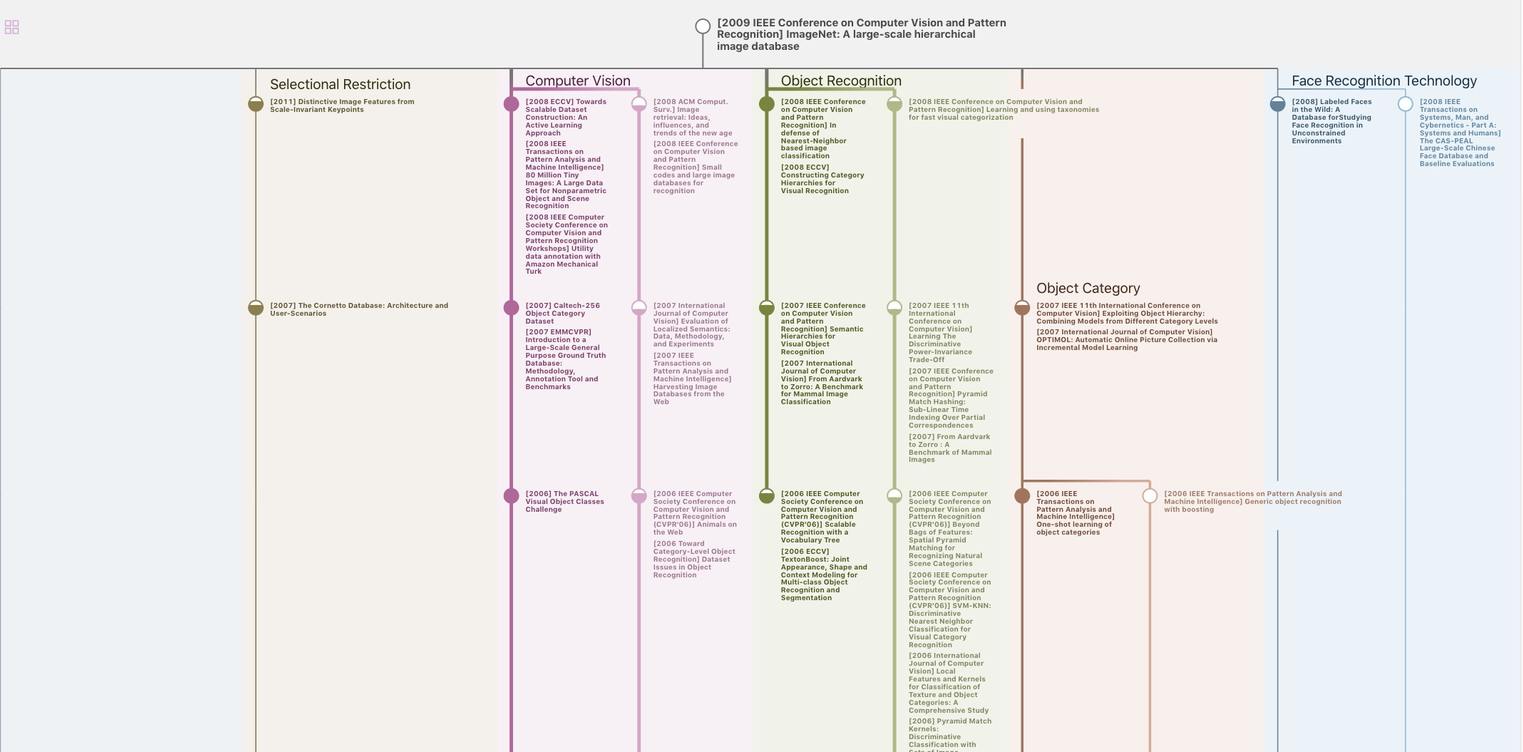
生成溯源树,研究论文发展脉络
Chat Paper
正在生成论文摘要