Backdoor Two-Stream Video Models on Federated Learning
ACM Transactions on Multimedia Computing, Communications, and Applications(2023)
Abstract
Video models on federated learning (FL) enable continual learning of the involved models for video tasks on end-user devices while protecting the privacy of end-user data. As a result, the security issues on FL, e.g., the backdoor attacks on FL and their defense have increasingly becoming the domains of extensive research in recent years. The backdoor attacks on FL are a class of poisoning attacks, in which an attacker, as one of the training participants, submits poisoned parameters and thus injects the backdoor into the global model after aggregation. Existing backdoor attacks against videos based on FL only poison RGB frames, which makes that the attack could be easily mitigated by two-stream model neutralization. Therefore, it is a big challenge to manipulate the most advanced two-stream video model with a high success rate by poisoning only a small proportion of training data in the framework of FL. In this paper, a new backdoor attack scheme incorporating the rich spatial and temporal structures of video data is proposed, which injects the backdoor triggers into both the optical flow and RGB frames of video data through multiple rounds of model aggregations. In addition, the adversarial attack is utilized on the RGB frames to further boost the robustness of the attacks. Extensive experiments on real-world datasets verify that our methods outperform the state-of-the-art backdoor attacks and show better performance in terms of stealthiness and persistence.
MoreTranslated text
Key words
Federated Learning,Backdoor Attack,Two-stream video Recognition,Adversarial Attack.
AI Read Science
Must-Reading Tree
Example
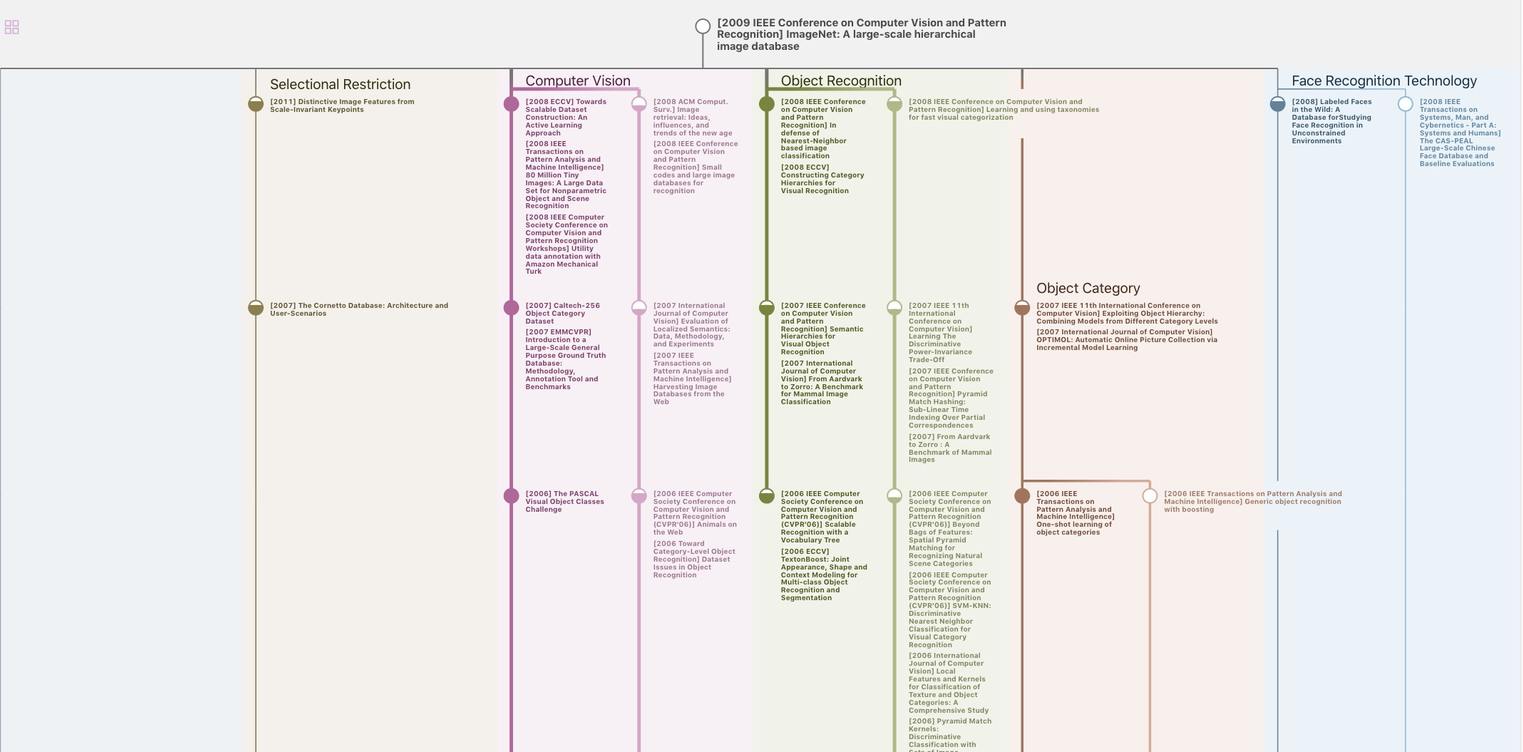
Generate MRT to find the research sequence of this paper
Chat Paper
Summary is being generated by the instructions you defined