Machine learning-assisted design of low elastic modulus β-type medical titanium alloys and experimental validation
Computational Materials Science(2024)
摘要
In this study, a method combining physical metallurgical models with machine learning was used to design β-type medical titanium alloys with low modulus of elasticity in Ti-Mo-Nb-Zr-Sn system alloys. The prediction model used the Extreme Gradient Boosting (XGBoost) algorithm to predict the elastic modulus of the alloys, and the Mo equivalent (Moeq value) and valence electron concentration ratio (e/a), which characterize the elastic modulus, were modeled as feature parameters that can improved the generalization ability of the model and reduced overfitting. Furthermore, a machine learning-based medical titanium alloys development framework was established, guided by three physical metallurgy models (Moeq value, d-electron theory, and e/a). A quaternary alloy Ti79.08Mo1.96Nb18Sn0.96 (at.%, II-1) and a quinary alloy Ti75.11Mo0.24Nb23.32Zr0.5Sn0.83 (at.%, II-2) were proposed based on the objective function set in this alloy development framework. For alloy II-1, the predicted elastic modulus value was 45 GPa and the experimental value was 43 GPa. For alloy II-2, the predicted elastic modulus value was 40 GPa and the experimental value was 37 GPa. The experimental validation results matched the predicted value of elastic modulus for the new alloys, and the addition of the physical parameters made the framework for the design of this β-type medical titanium alloy follow the physical metallurgy principles more closely. Therefore, combining machine learning and physical metallurgical models to develop and design β-type medical alloy can significantly improve the efficiency of alloy design.
更多查看译文
关键词
Medical titanium alloy,Machine learning,Performance prediction,Alloy design,Low elastic modulus
AI 理解论文
溯源树
样例
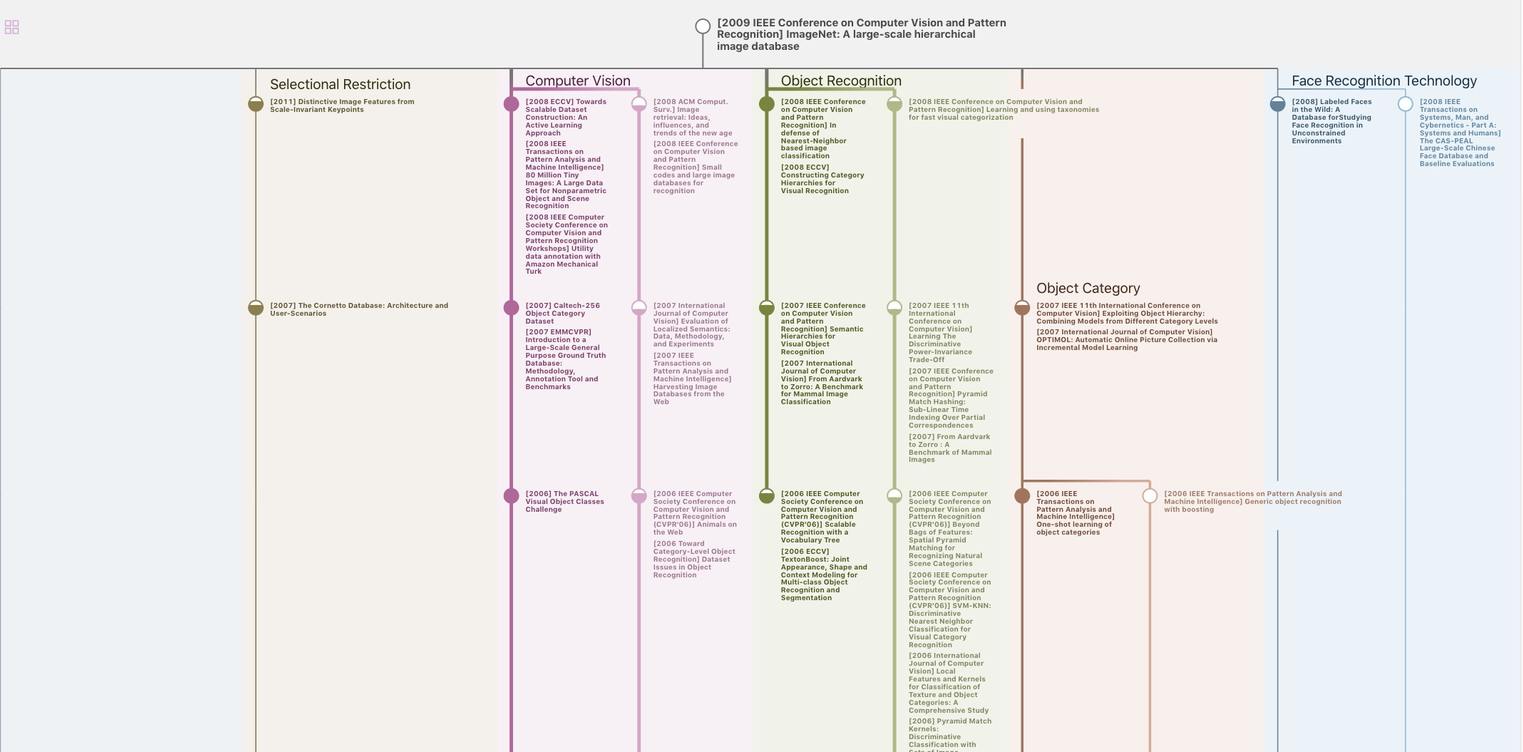
生成溯源树,研究论文发展脉络
Chat Paper
正在生成论文摘要