Multitask feature selection within structural datasets
Data-Centric Engineering(2024)
摘要
Population-based structural health monitoring (PBSHM) systems use data from multiple structures to make inferences of health states. An area of PBSHM that has recently been recognized for potential development is the use of multitask learning (MTL) algorithms that differ from traditional single-task learning. This study presents an application of the MTL approach, Joint Feature Selection with LASSO, to provide automatic feature selection. The algorithm is applied to two structural datasets. The first dataset covers a binary classification between the port and starboard side of an aircraft tailplane, for samples from two aircraft of the same model. The second dataset covers normal and damaged conditions for pre- and postrepair of the same aircraft wing. Both case studies demonstrate that the MTL results are interpretable, highlighting features that relate to structural differences by considering the patterns shared between tasks. This is opposed to single-task learning, which improved accuracy at the cost of interpretability and selected features, which failed to generalize in previously unobserved experiments.
更多查看译文
关键词
feature selection,generalization,multitask learning,sparsity,structural health monitoring
AI 理解论文
溯源树
样例
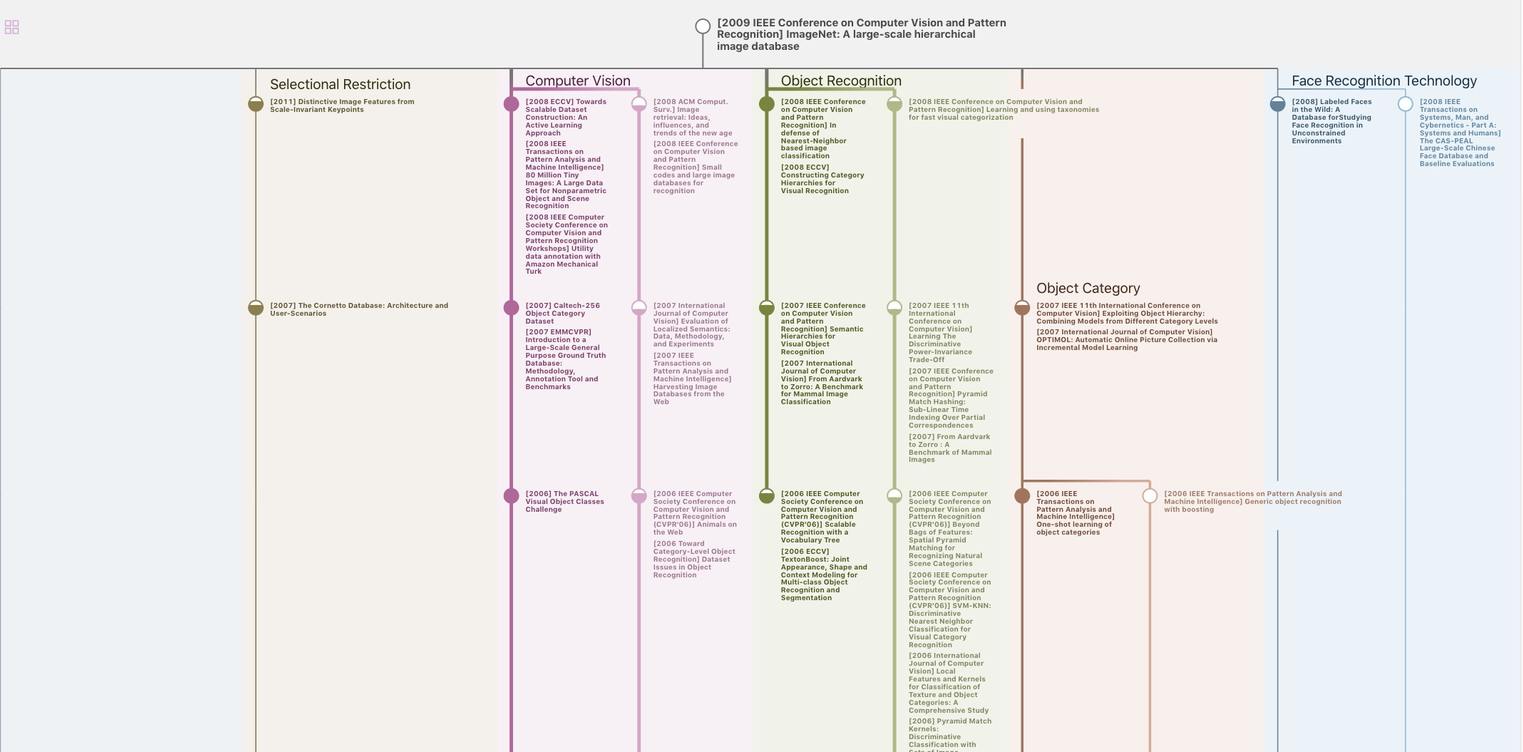
生成溯源树,研究论文发展脉络
Chat Paper
正在生成论文摘要