Does Confusion Really Hurt Novel Class Discovery?
International Journal of Computer Vision(2024)
摘要
When sampling data of specific classes (i.e., known classes) for a scientific task, collectors may encounter unknown classes (i.e., novel classes). Since these novel classes might be valuable for future research, collectors will also sample them and assign them to several clusters with the help of known-class data. This assigning process is known as novel class discovery (NCD). However, category confusion is common in the sampling process and may make the NCD unreliable. To tackle this problem, this paper introduces a new and more realistic setting, where collectors may misidentify known classes and even confuse known classes with novel classes—we name it NCD under unreliable sampling (NUSA). We find that NUSA will empirically degrade existing NCD methods if taking no care of sampling errors. To handle NUSA, we propose an effective solution, named hidden-prototype-based discovery network (HPDN): (1) we try to obtain relatively clean data representations even with the confusedly sampled data; (2) we propose a mini-batch K-means variant for robust clustering, alleviating the negative impact of residual errors embedded in the representations by detaching the noisy supervision timely. Experiments demonstrate that, under NUSA, HPDN significantly outperforms competitive baselines (e.g., 6% more than the best baseline on CIFAR-10) and remains robust when encountering serious sampling errors.
更多查看译文
关键词
Novel class discovery,Open-world recognition,Semi-supervised learning,Transfer learning
AI 理解论文
溯源树
样例
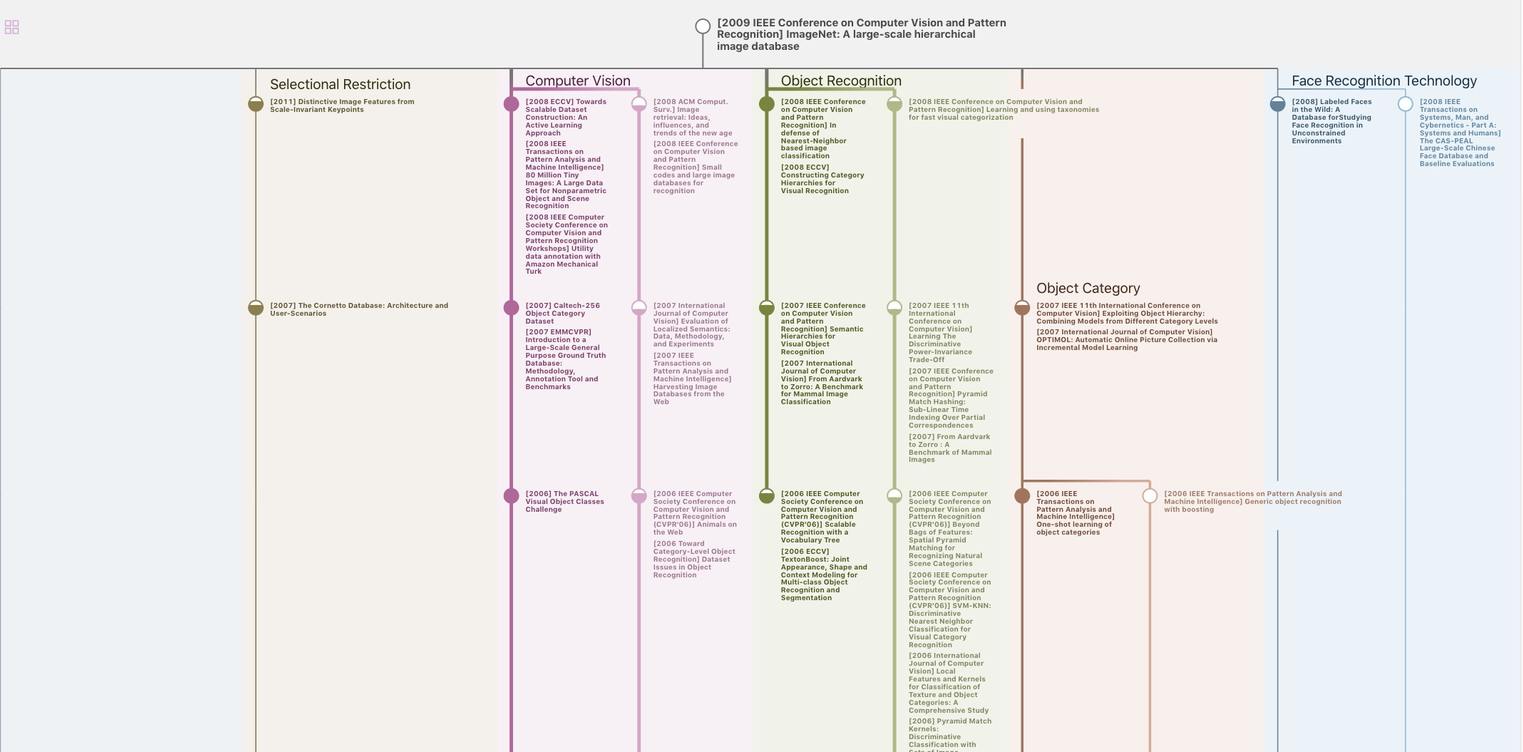
生成溯源树,研究论文发展脉络
Chat Paper
正在生成论文摘要