Building Individual Player Performance Profiles According to Pre-Game Expectations and Goal Difference in Soccer
SENSORS(2024)
摘要
Soccer player performance is influenced by multiple unpredictable factors. During a game, score changes and pre-game expectations affect the effort exerted by players. This study used GPS wearable sensors to track players' energy expenditure in 5-min intervals, alongside recording the goal timings and the win and lose probabilities from betting sites. A mathematical model was developed that considers pre-game expectations (e.g., favorite, non-favorite), endurance, and goal difference (GD) dynamics on player effort. Particle Swarm and Nelder-Mead optimization methods were used to construct these models, both consistently converging to similar cost function values. The model outperformed baselines relying solely on mean and median power per GD. This improvement is underscored by the mean absolute error (MAE) of 396.87 +/- 61.42 and root mean squared error (RMSE) of 520.69 +/- 88.66 achieved by our model, as opposed to the B1 MAE of 429.04 +/- 84.87 and RMSE of 581.34 +/- 185.84, and B2 MAE of 421.57 +/- 95.96 and RMSE of 613.47 +/- 300.11 observed across all players in the dataset. This research offers an enhancement to the current approaches for assessing players' responses to contextual factors, particularly GD. By utilizing wearable data and contextual factors, the proposed methods have the potential to improve decision-making and deepen the understanding of individual player characteristics.
更多查看译文
关键词
optimization,model fitting,energy expenditure,soccer,player profiling,GPS data
AI 理解论文
溯源树
样例
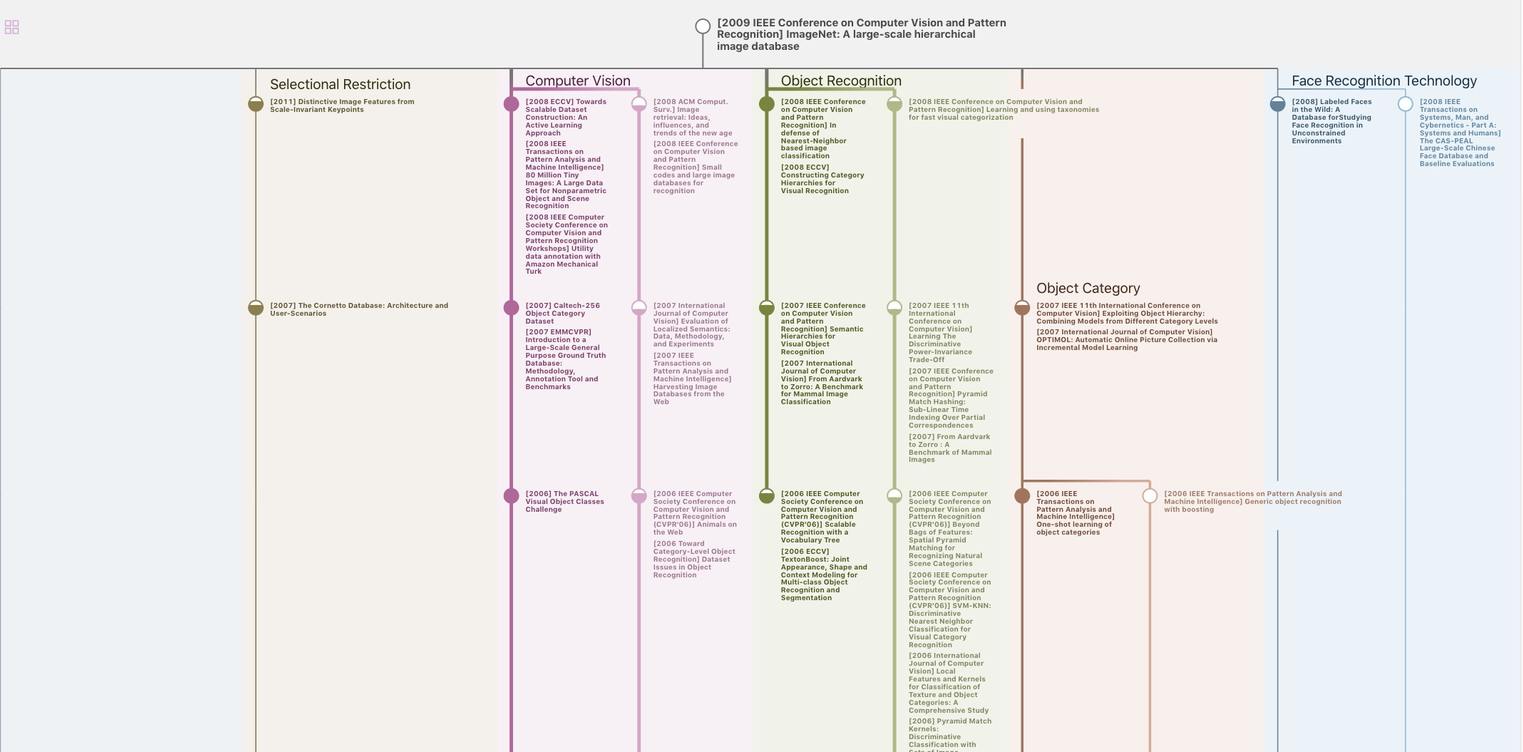
生成溯源树,研究论文发展脉络
Chat Paper
正在生成论文摘要