Multi-Modal Attentive Prompt Learning for Few-shot Emotion Recognition in Conversations.
J. Artif. Intell. Res.(2024)
摘要
Emotion recognition in conversations (ERC) has emerged as an important research area in Natural Language Processing and Affective Computing, focusing on accurately identifying emotions within the conversational utterance. Conventional approaches typically rely on labeled training samples for fine-tuning pre-trained language models (PLMs) to enhance classification performance. However, the limited availability of labeled data in real-world scenarios poses a significant challenge, potentially resulting in diminished model performance. In response to this challenge, we present the Multi-modal Attentive Prompt (MAP) learning framework, tailored specifically for few-shot emotion recognition in conversations. The MAP framework consists of four integral modules: multi-modal feature extraction for the sequential embedding of text, visual, and acoustic inputs; a multi-modal prompt generation module that creates six manually-designed multi-modal prompts; an attention mechanism for prompt aggregation; and an emotion inference module for emotion prediction. To evaluate our proposed model’s efficacy, we conducted extensive experiments on two widely recognized benchmark datasets, MELD and IEMOCAP. Our results demonstrate that the MAP framework outperforms state-of-the-art ERC models, yielding notable improvements of 3.5% and 0.4% in micro F1 scores. These findings highlight the MAP learning framework’s ability to effectively address the challenge of limited labeled data in emotion recognition, offering a promising strategy for improving ERC model performance.
更多查看译文
AI 理解论文
溯源树
样例
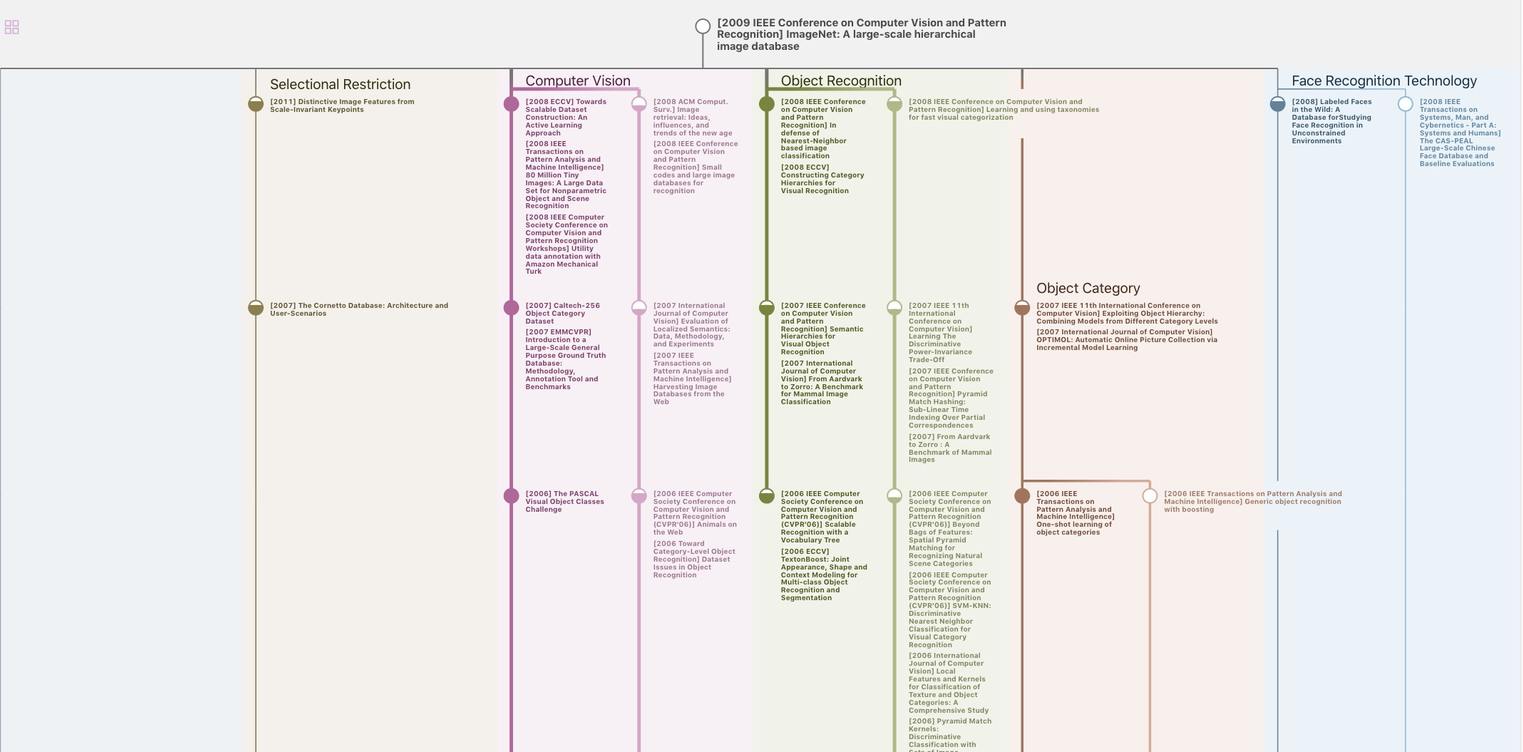
生成溯源树,研究论文发展脉络
Chat Paper
正在生成论文摘要