Efficient Stereo Matching Using Swin Transformer and Multilevel Feature Consistency in Autonomous Mobile Systems
IEEE TRANSACTIONS ON INDUSTRIAL INFORMATICS(2024)
摘要
In this article, we propose a Swin Transformer and multilevel Feature Consistency based Network (STFC-Net), which is a multilevel cascade stereo matching method to predict the disparity in a coarse-to-fine manner. 1) To alleviate the problem of the limited receptive field of existing convolutional neural network (CNN)-based methods, inspired by the capability of modeling the large-scale dependence of transformer, we adopt a multilevel feature extraction module combining CNN and Swin Transformer to capture long-range context information; a multiscale cascaded cost aggregation module is used to cover different image regions with less memory consumption. 2) To make full use of the hierarchical features, we checked the multilevel left-right feature consistency in an unsupervised manner to improve the disparity accuracy. The experimental results show that our method outperforms some previous CNN methods on the Scene Flow and KITTI datasets with lower computational time complexity. Moreover, it generalizes well in some unknown and challenging real-world scenarios.
更多查看译文
关键词
Costs,Feature extraction,Transformers,Computational modeling,Task analysis,Image reconstruction,Unsupervised learning,Disparity estimation,feature consistency,stereo matching,transformer
AI 理解论文
溯源树
样例
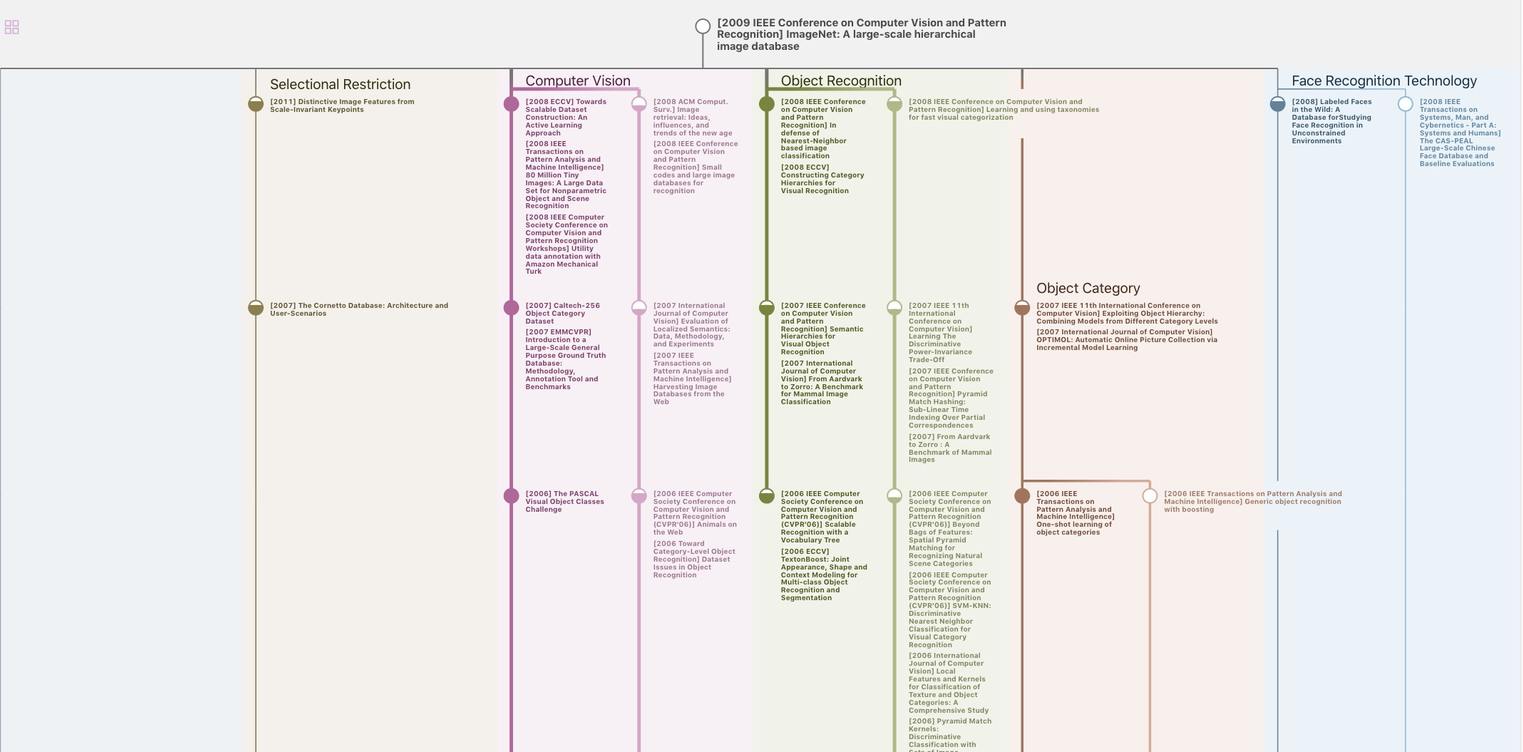
生成溯源树,研究论文发展脉络
Chat Paper
正在生成论文摘要