Development and Evaluation of Deep Learning Models for Cardiotocography Interpretation
crossref(2024)
摘要
The inherent variability in the visual interpretation of cardiotocograms (CTGs) by obstetric clinical experts, both intra- and inter-observer, presents a substantial challenge in obstetric care. In response, we investigate automated CTG interpretation as a potential solution to enhance the early detection of fetal hypoxia during labor, which has the potential to reduce unnecessary operative interventions and improve overall maternal and neonatal care. This study employs deep learning techniques to reduce the subjectivity associated with visual CTG interpretation. Our results demonstrate that using objective umbilical cord blood pH outcome measurements, rather than clinician-defined Apgar scores, yields more consistent and robust model performance. Additionally, through a series of ablation studies, we explore the impact of temporal distribution shifts on the performance of these deep learning models. We examine tradeoffs between performance and fairness, specifically evaluating performance across demographic and clinical subgroups. Finally, we discuss the practical implications of our findings for the real-world deployment of such systems, emphasizing their potential utility in medical settings with limited resources.
### Competing Interest Statement
All authors except N.C. are currently employed by Google, a for-profit entity which may stand to benefit financially from the models developed and presented in the paper. Authors have no other competing interests
### Funding Statement
This study was funded by Google Research
### Author Declarations
I confirm all relevant ethical guidelines have been followed, and any necessary IRB and/or ethics committee approvals have been obtained.
Yes
The details of the IRB/oversight body that provided approval or exemption for the research described are given below:
The study used (or will use) ONLY openly available human data that were originally located at CTU-CHB Intrapartum Cardiotocography Database on Physionet: https://physionet.org/content/ctu-uhb-ctgdb/1.0.0/
I confirm that all necessary patient/participant consent has been obtained and the appropriate institutional forms have been archived, and that any patient/participant/sample identifiers included were not known to anyone (e.g., hospital staff, patients or participants themselves) outside the research group so cannot be used to identify individuals.
Yes
I understand that all clinical trials and any other prospective interventional studies must be registered with an ICMJE-approved registry, such as ClinicalTrials.gov. I confirm that any such study reported in the manuscript has been registered and the trial registration ID is provided (note: if posting a prospective study registered retrospectively, please provide a statement in the trial ID field explaining why the study was not registered in advance).
Yes
I have followed all appropriate research reporting guidelines, such as any relevant EQUATOR Network research reporting checklist(s) and other pertinent material, if applicable.
Yes
We used an open source dataset found at: https://physionet.org/content/ctu-uhb-ctgdb/1.0.0/ All additional data produced in the present study are available upon reasonable request to the authors
更多查看译文
AI 理解论文
溯源树
样例
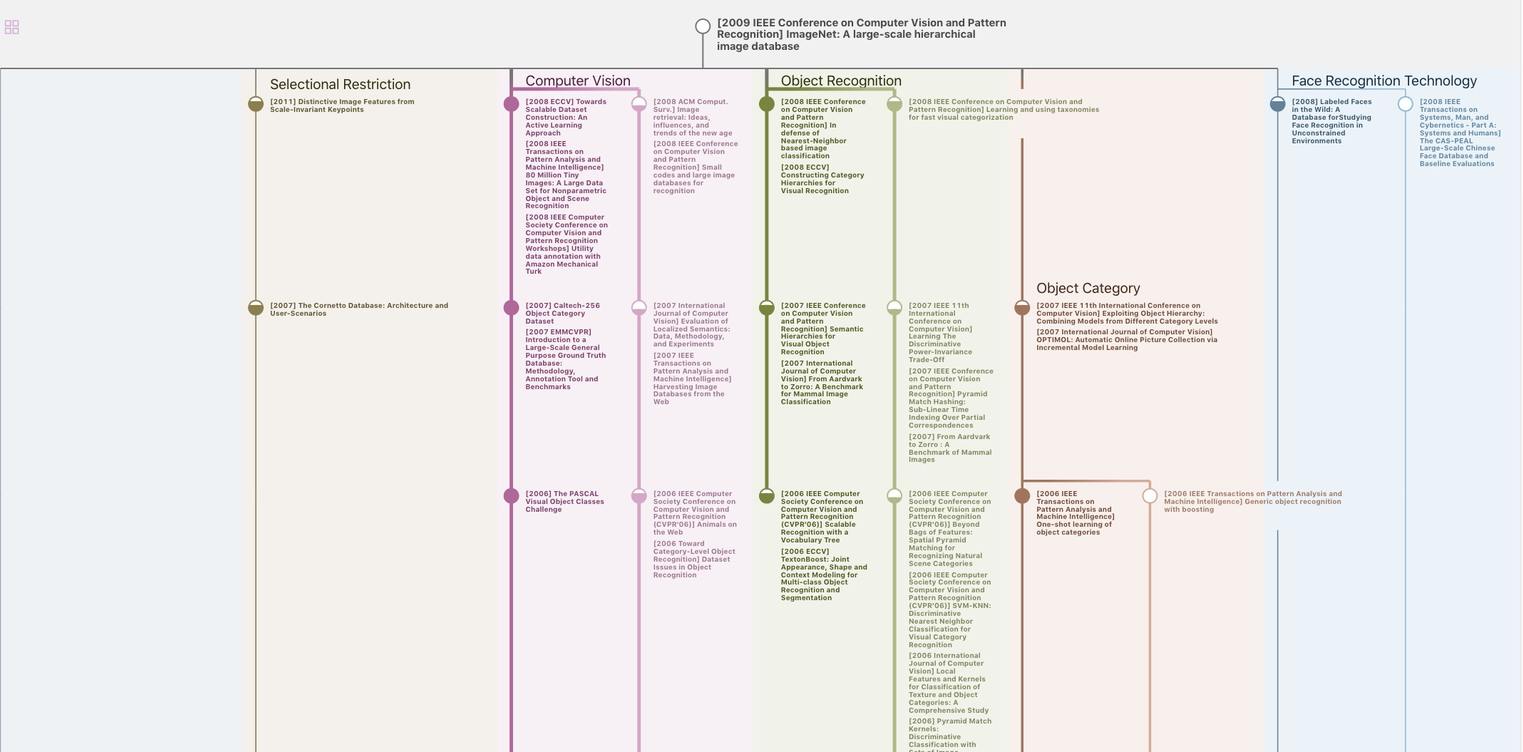
生成溯源树,研究论文发展脉络
Chat Paper
正在生成论文摘要