Continual Learning With Siamese Neural Networks for Sustainable Network Management
IEEE Transactions on Network and Service Management(2024)
摘要
With emerging ML techniques showing great potential in prediction performance, there is an inclination towards ML for network management. Although modern mobile network deployments have access to essential data storage and computational resources, they would benefit remarkably from more sustainable and cost-effective approaches for ML applications. Data-efficient AI allows network vendors to train ML models on less data, which relieves memory and computational requirements. To explore a solution to these problems, this paper dives deeper into SNN, a promising data-efficient ML technique. Furthermore, to improve data efficiency, an extension of the SNN framework is proposed by applying a memory-based continual learning algorithm, Experience Replay. Empirical evaluations are conducted using several datasets, including a dataset from real live mobile networks, to illustrate the potential of SNNs and to highlight the contribution to sustainable AI for mobile networks. The findings show that in this context, SNNs can outperform standard classification neural networks, under both the standard multi-class classification case and the continual learning case, while trained on significantly less data.
更多查看译文
关键词
Machine learning,Telecommunication network management,Sustainable computing
AI 理解论文
溯源树
样例
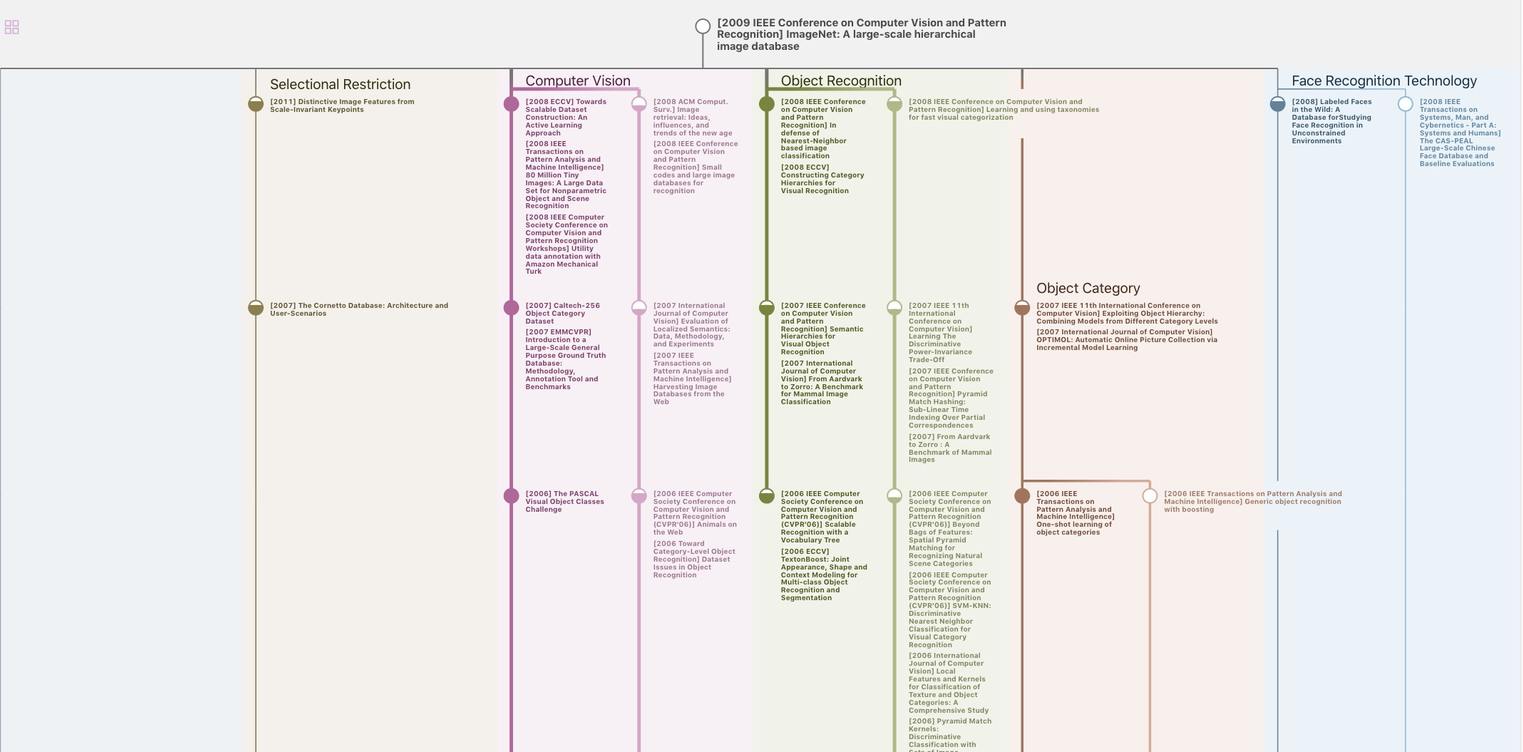
生成溯源树,研究论文发展脉络
Chat Paper
正在生成论文摘要