Sediment Characterization Based on Portable Free Fall Penetrometer Measurements Using a Deep Neural Network
GEO-CONGRESS 2024: GEOTECHNICAL DATA ANALYSIS AND COMPUTATION(2024)
摘要
Characterizing seabed sediment is vital for offshore and nearshore engineering projects, often relying on in situ tests due to challenges in obtaining seabed samples. While cone penetration testing (CPT) is the conventional choice for seabed characterization, portable free fall penetrometers (PFFP) offer a cost-effective alternative in challenging environments, focusing on surficial seabed data. However, streamlined soil classification methods for PFFP data are lacking, as CPT-based approaches may not directly apply due to different penetration rates. This study introduces a 1D convolutional neural network (CNN) to predict sediment classes using the deceleration curve from a PFFP. Soil samples from deployment sites (Sequim Bay, WA; York and Potomac Rivers, VA) underwent laboratory testing for true classification labels. Initial results exhibit an 80% accuracy, suggesting potential practical applicability with further refinement and additional training data. This approach holds promise for reliable and practical seabed sediment classification in geotechnical applications.
更多查看译文
AI 理解论文
溯源树
样例
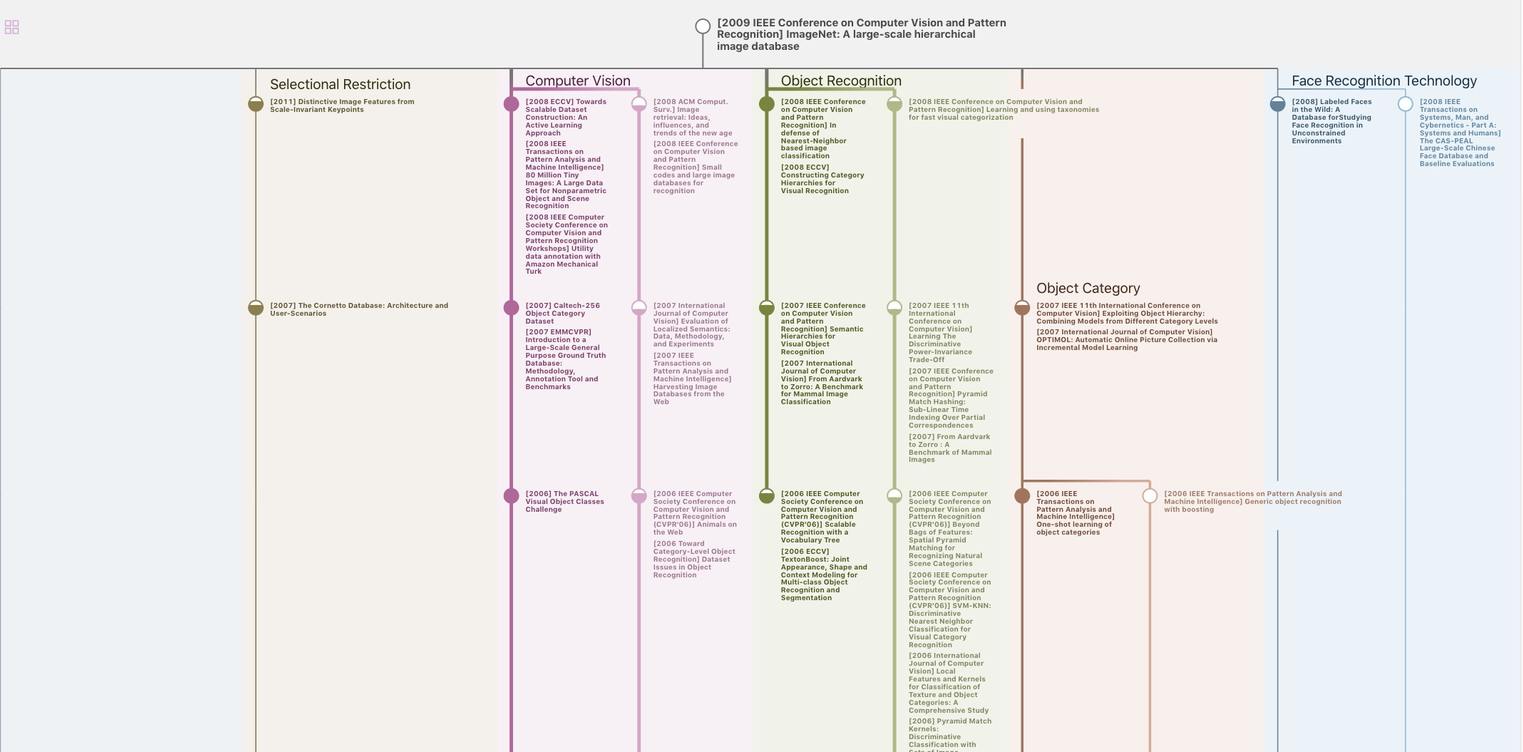
生成溯源树,研究论文发展脉络
Chat Paper
正在生成论文摘要