A Comprehensive Review: Analysis of Machine Learning, Deep Learning, and Large Language Model Techniques for Revolutionizing Driver Identification
crossref(2024)
摘要
Driver identification is crucial for various applications including automotive security, law enforcement, and the ride-sharing industry, as well as for advanced driver assistance systems, fleet management, and usage-based insurance. Machine learning and deep learning techniques have emerged as promising approaches for accurate identification, yet a comprehensive analysis of existing methods remains unattainable. Despite significant research efforts, no in-depth survey has thoroughly evaluated and compared existing driver identification techniques, impeding the development of optimal solutions. This gap restricts our understanding of their strengths, weaknesses, and potential impact. This paper addresses this critical gap by comprehensively reviewing and analyzing existing driver identification techniques. We delve into various methodologies, including preprocessing feature extraction, classification algorithms, and deep learning architectures. We critically evaluate their performance, highlighting unique features, potential advantages, and limitations. Additionally, we proposed the future framework for driver identification with Large Language Model (LLM) and explored the developing potential of LLM in this domain. We employ relevant research articles published in prominent scientific databases. Our analysis reveals diverse driver identification techniques, each offering unique advantages and disadvantages. Traditional methods like Support Vector Machines and Random Forests provide reliable performance, while deep learning architectures achieve higher accuracy but require larger datasets and computational resources. We also identify potential synergies between established techniques and emerging technologies like LLM, suggesting promising avenues for future research. We propose several key research directions based on our findings to further advance driver identification accuracy and robustness. These include exploring hybrid approaches combining traditional and deep learning methods and investigating transfer learning techniques for efficient adaptation to new data sets. This comprehensive review provides a valuable resource for researchers and practitioners interested in driver identification. We highlight the strengths and weaknesses of existing techniques, identify potential for future advancements, and suggest promising research directions to ultimately achieve highly accurate and reliable driver identification systems.
更多查看译文
AI 理解论文
溯源树
样例
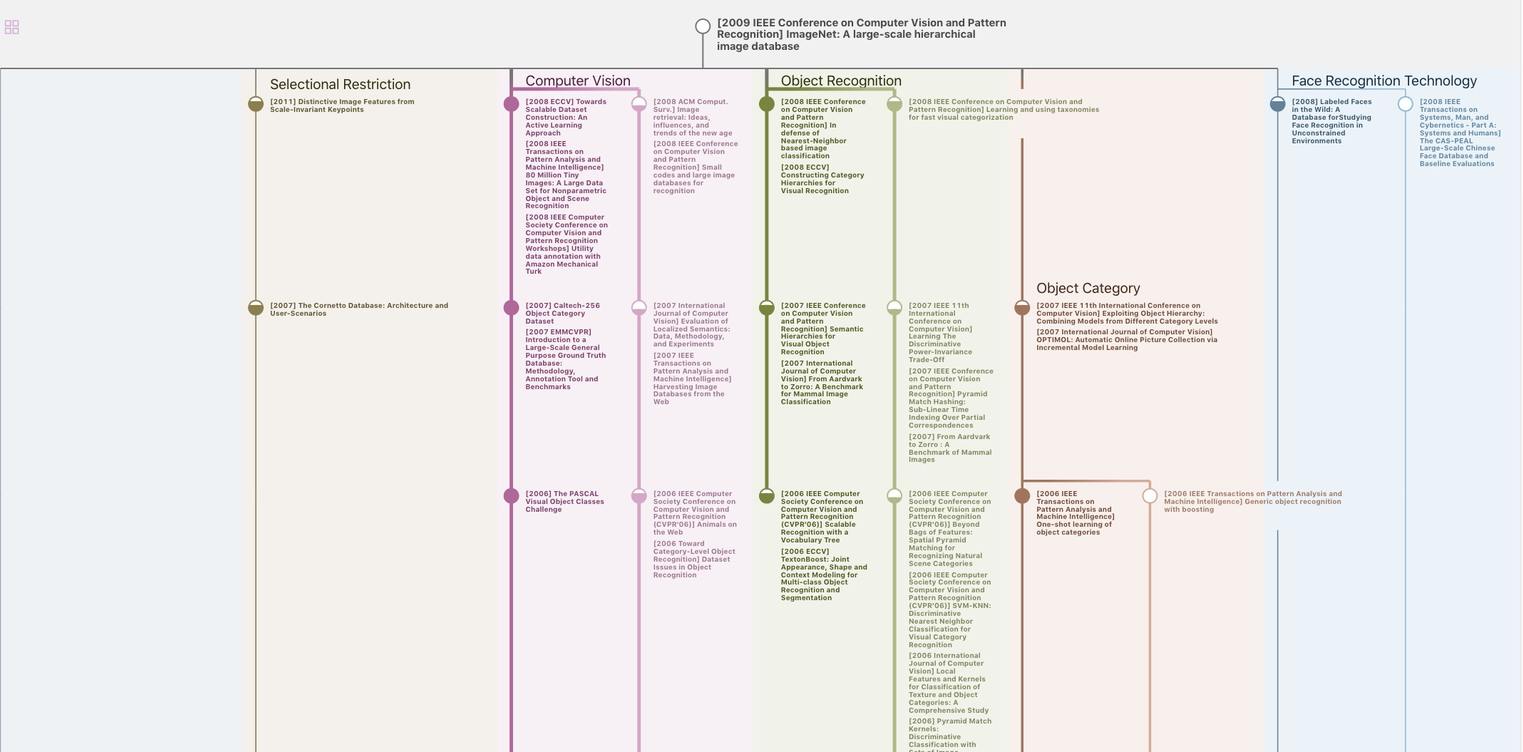
生成溯源树,研究论文发展脉络
Chat Paper
正在生成论文摘要