Learnable product quantization for anomaly detection
Neurocomputing(2024)
摘要
In many anomaly detection applications, anomaly samples are difficult to obtain. We propose a novel product quantization (PQ)-based anomaly detection scheme: Learnable Product Quantization (LPQ), which only requires very few abnormal samples to train the model. The scheme extracts feature from high-dimensional data using the deep learning network, decomposes the feature space into a Cartesian product of low dimensional subspaces using PQ, and then produces sub-codebooks consisting of sub-codewords with clustering techniques. As a result, the extracted features with similar sub-vector are mapped into the same bucket, which reduces the time complexity of nearest neighbor retrieval significantly. In order to achieve reasonable codebooks, a PQ Table is embedded into the network. While training, we propose a novel metric learning strategy that makes the semantically similar (normal) samples closer and dissimilar (outlier) samples farther. The experimental results on benchmark datasets demonstrate that our metric learning strategy is better than the triplet loss and the sigmoid cross-entropy loss on the anomaly detection task. In general, LPQ shows excellent performance and high efficiency in anomaly detection.
更多查看译文
关键词
Anomaly detection,Product quantization,Deep learning,Quantization error
AI 理解论文
溯源树
样例
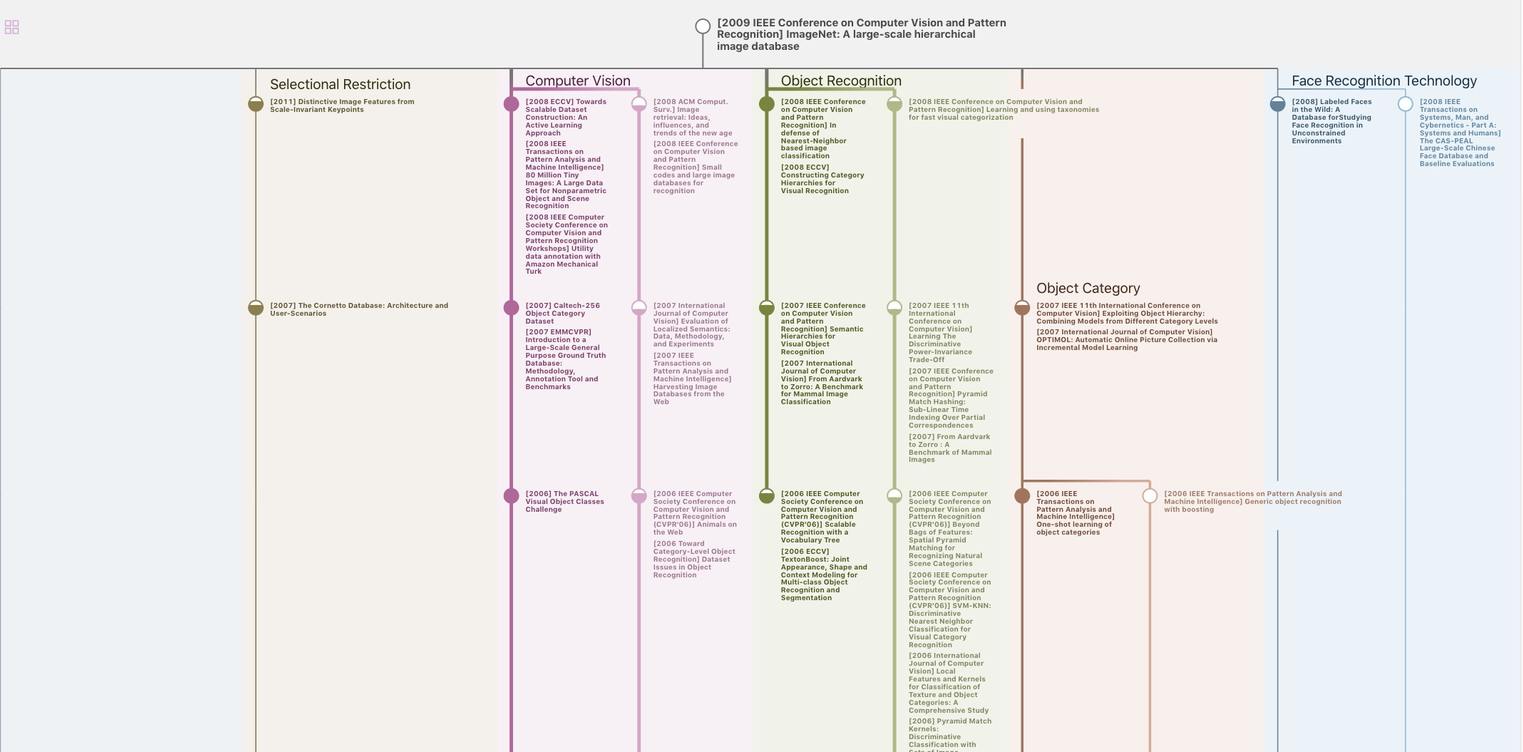
生成溯源树,研究论文发展脉络
Chat Paper
正在生成论文摘要