Enhancing Few-Shot 3D Point Cloud Semantic Segmentation through Bidirectional Prototype Learning
PROCEEDINGS OF 2023 9TH INTERNATIONAL CONFERENCE ON ROBOTICS AND ARTIFICIAL INTELLIGENCE, ICRAI 2023(2023)
摘要
In recent years, significant strides have been made in point cloud semantic segmentation, which, however, are unspectacular when the training is deprived of sufficient densely-annotated samples, especially with the face of new classes unseen during the training. Given limited data and unacquainted categories, learning efficiency becomes of great concern to the overall segmentation outcome. To obtain improved segmentation performance under this few-shot training condition, we introduce a bidirectional learning method that allows mutual prototype learning between support set and query set. Specifically, we manage to realize enhanced efficiency by exploiting the support and query sets to a larger extent, effectively extracting information and generating prototypes in two opposite learning orientations. Refined by our method, models are able to achieve better performance in few-shot 3D semantic segmentation tasks without the need of further introducing more parameters that may lead to higher model complexity. To validate our method, we respectively test different models for 1-shot and 5-shot settings on the S3DIS [23] dataset. The remarkably improved IoU scores on unseen classes in the evaluation tests show the effectiveness of our proposed method.
更多查看译文
关键词
semantic segmentation,few-shot learning,prototype learning,3D point cloud
AI 理解论文
溯源树
样例
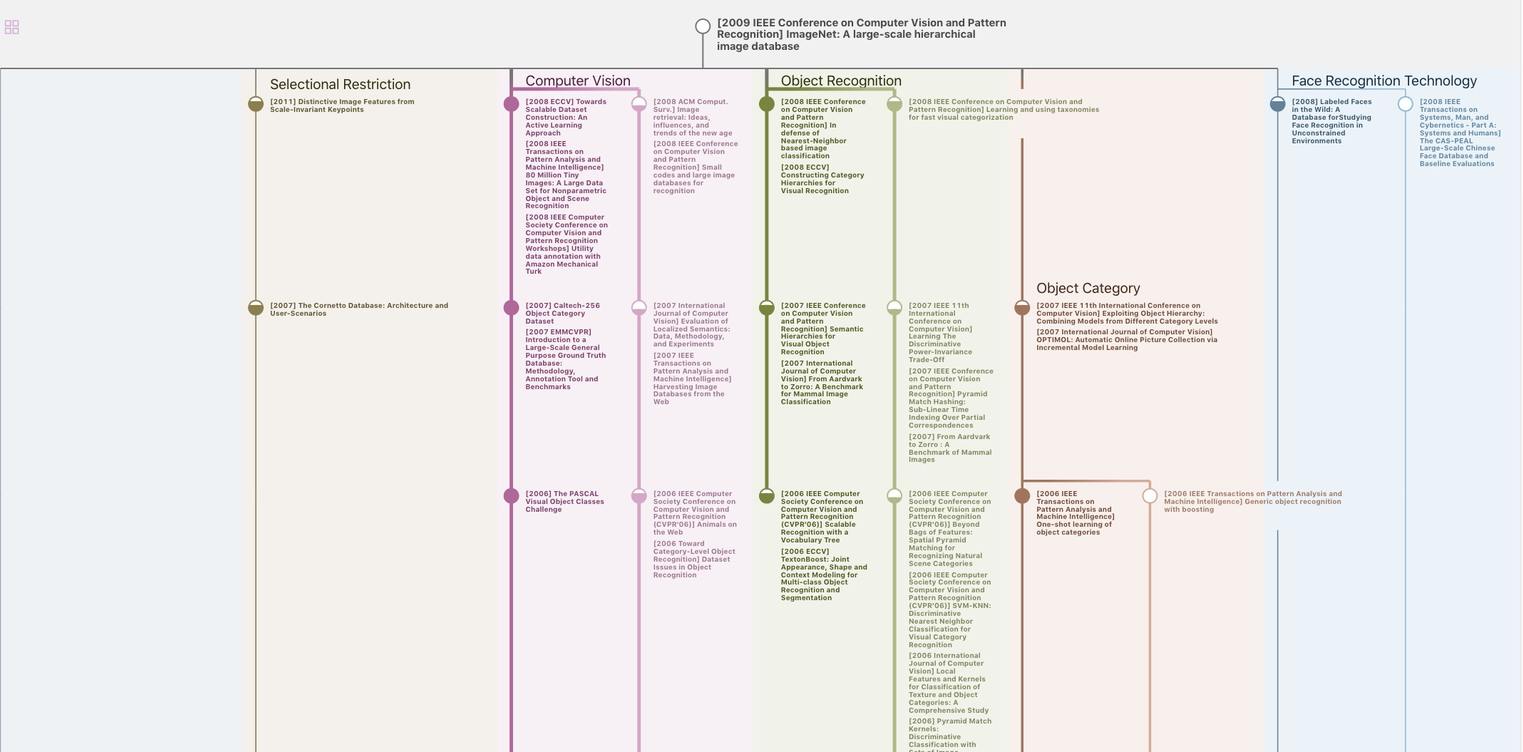
生成溯源树,研究论文发展脉络
Chat Paper
正在生成论文摘要