TagSleep3D: RF-based 3D Sleep Posture Skeleton Recognition.
Proc. ACM Interact. Mob. Wearable Ubiquitous Technol.(2024)
摘要
Sleep posture plays a crucial role in maintaining good morpheus quality and overall health. As a result, long-term monitoring of 3D sleep postures is significant for sleep analysis and chronic disease prevention. To recognize sleep postures, traditional methods either use cameras to record image data or require the user to wear wearable devices or sleep on pressure mattresses. However, these methods could raise privacy concerns and cause discomfort during sleep. Accordingly, the RF (Radio Frequency) based method has emerged as a promising alternative. Despite most of these methods achieving high precision in classifying sleep postures, they struggle to retrieve 3D sleep postures due to difficulties in capturing 3D positions of static body joints. In this work, we propose TagSleep3D to resolve all the above issues. Specifically, inspired by the concept of RFID tag sheets, we explore the possibility of recognizing 3D sleep posture by deploying an RFID tag array under the bedsheet. When a user sleeps in bed, the signals of some tags could be blocked or reflected by the sleep posture, which can produce a body imprint. We then propose a novel deep learning model composed of the attention mechanism, convolutional neural network, and together with two data augmentation methods to retrieve the 3D sleep postures by analyzing these body imprints. We evaluate TagSleep3D with 43 users and we totally collect 27,300 sleep posture samples. Our extensive experiments demonstrate that TagSleep3D can recognize each joint on the human skeleton with a median MPJPE (Mean Per Joint Position Error) of 4.76 cm for seen users and 7.58 cm for unseen users.
更多查看译文
关键词
3D Skeletons,Neural Network,RFIDs,Sleep Postures
AI 理解论文
溯源树
样例
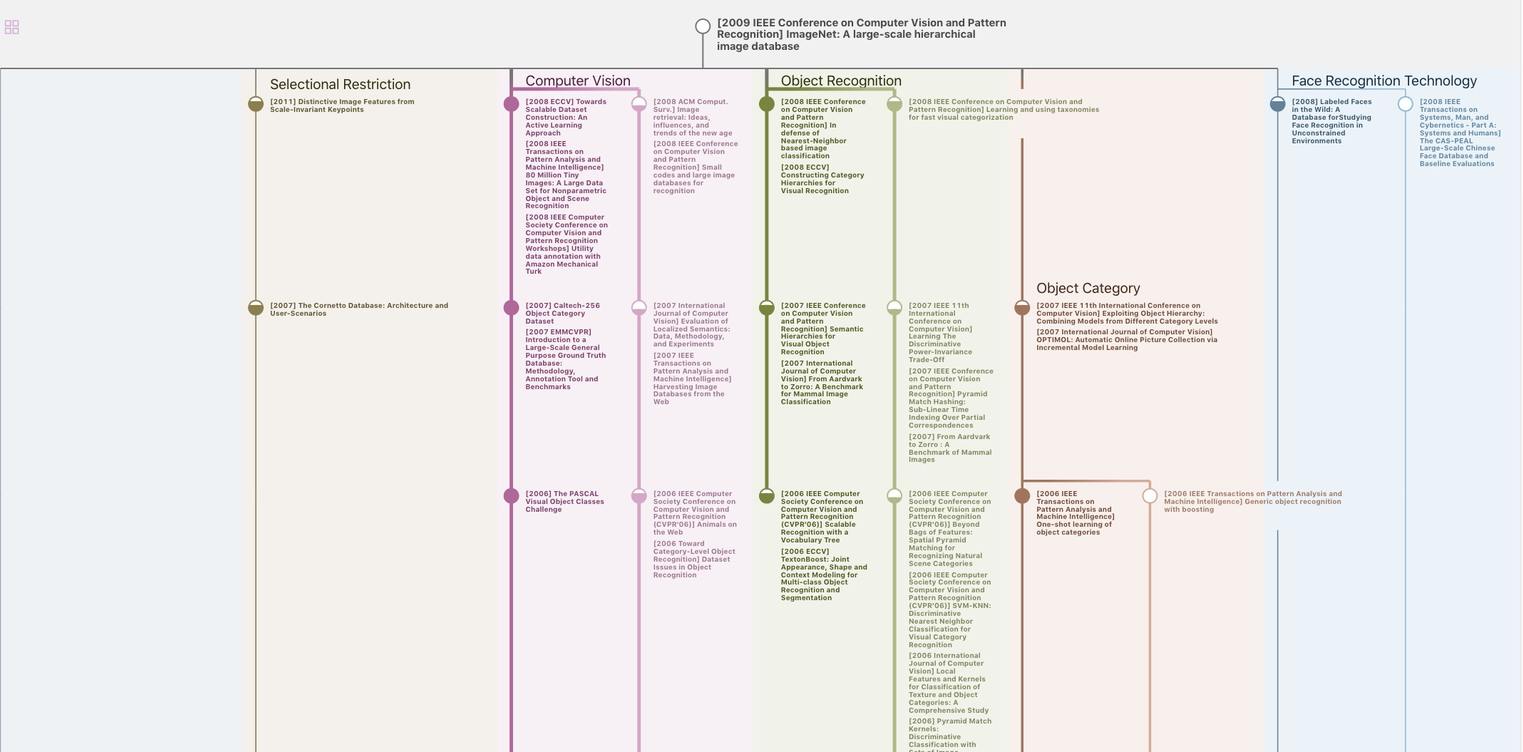
生成溯源树,研究论文发展脉络
Chat Paper
正在生成论文摘要