Synthesising realistic 2D microstructures of unidirectional fibre-reinforced composites with a generative adversarial network
COMPOSITES SCIENCE AND TECHNOLOGY(2024)
摘要
The microstructure governs the behaviour of unidirectional fibre-reinforced composites. In this study, we developed a Deep Convolutional Generative Adversarial Network (DCGAN) to generate realistic 2D transverse microstructures of such composites. We evaluated the DCGAN-generated microstructures using three different methods: Fre ' chet inception distance, walking through the latent space, and feature matching. The results from these evaluations confirmed that the generated microstructures are distinct and not simply a replication of the training data. The generated microstructures were then compared to real microstructures, confirming that they match qualitatively and quantitatively with respect to detailed statistical characteristics, including fibre diameters, fibre volume fraction, fibre spatial distribution, and resin-rich pockets. We also found that microstructures created by traditional generators could not capture the real resin-rich pockets. This illustrates the capability and value of DCGAN to generate realistic transverse microstructures and provides insights for modelling methods based on real images.
更多查看译文
关键词
A. Polymer-matrix composites (PMCs),C. Statistics,D. X-ray computed tomography,Deep-learning image synthesis
AI 理解论文
溯源树
样例
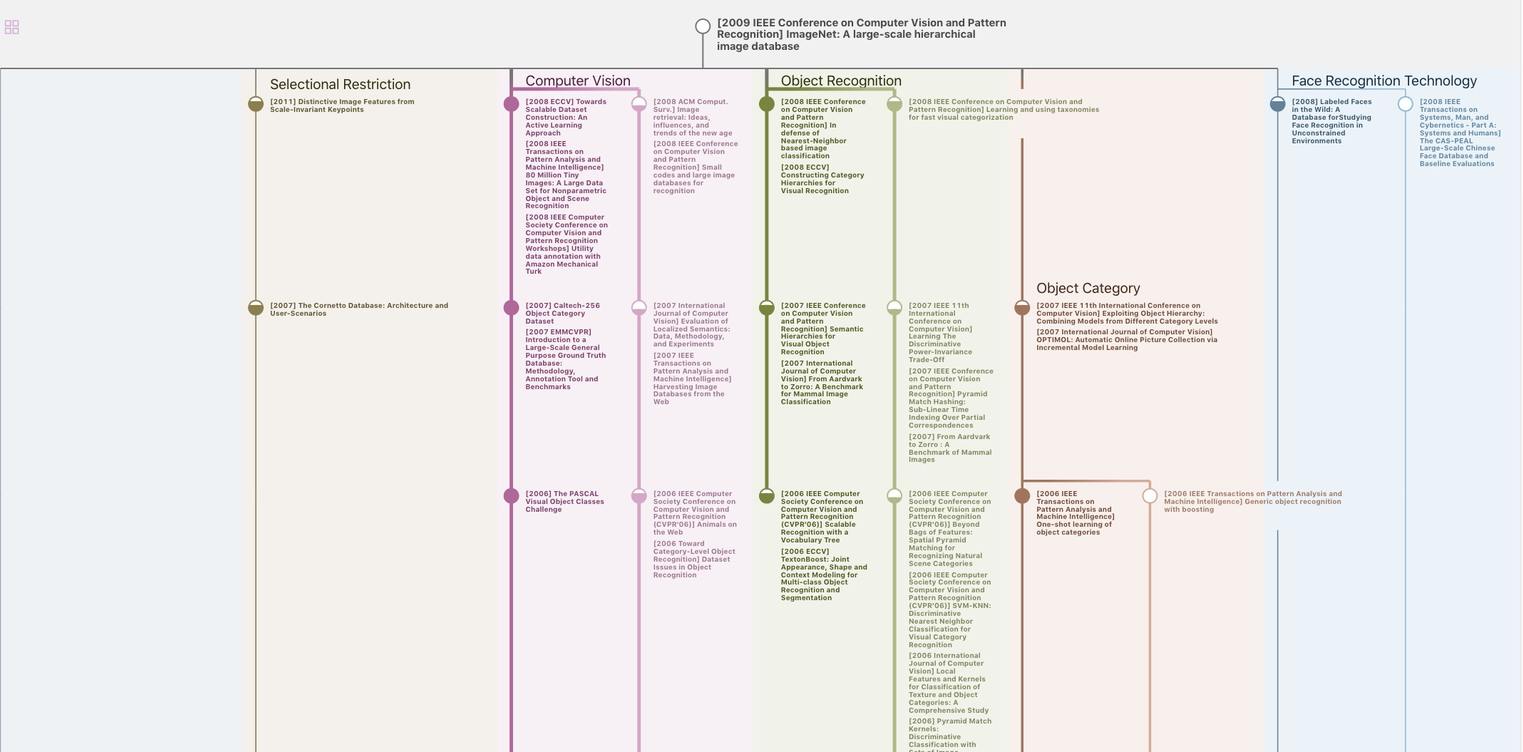
生成溯源树,研究论文发展脉络
Chat Paper
正在生成论文摘要