Task Sub-type States Decoding Via Group Deep Bidirectional Recurrent Neural Network
Medical Image Analysis(2024)
摘要
Decoding brain states under different cognitive tasks from functional magnetic resonance imaging (fMRI) data has attracted great attention in the neuroimaging filed. However, the well-known temporal dependency in fMRI sequences has not been fully exploited in existing studies, due to the limited temporal-modeling capacity of the backbone machine learning algorithms and rigid training sample organization strategies upon which the brain decoding methods are built. To address these limitations, we propose a novel method for fine-grain brain state decoding, namely, group deep bidirectional recurrent neural network (Group-DBRNN) model. We first propose a training sample organization strategy that consists of a group-task sample generation module and a multiple-scale random fragment strategy (MRFS) module to collect training samples that contain rich task-relevant brain activity contrast (i.e., the comparison of neural activity patterns between different tasks) and maintain the temporal dependency. We then develop a novel decoding model by replacing the unidirectional RNNs that are widely used in existing brain state decoding studies with bidirectional stacked RNNs to better capture the temporal dependency, and by introducing a multi-task interaction layer (MTIL) module to effectively model the task-relevant brain activity contrast. Our experimental results on the Human Connectome Project task fMRI dataset (7 tasks consisting of 23 task sub-type states) show that the proposed model achieves an average decoding accuracy of 94.7% over the 23 fine-grain sub-type states. Meanwhile, our extensive interpretations of the intermediate features learned in the proposed model via visualizations and quantitative assessments of their discriminability and inter-subject alignment evidence that the proposed model can effectively capture the temporal dependency and task-relevant contrast.
更多查看译文
关键词
Brain stage decoding,functional magnetic resonance imaging,temporal dependency,task-relevant brain activity contrast,bidirectional stacked RNNs
AI 理解论文
溯源树
样例
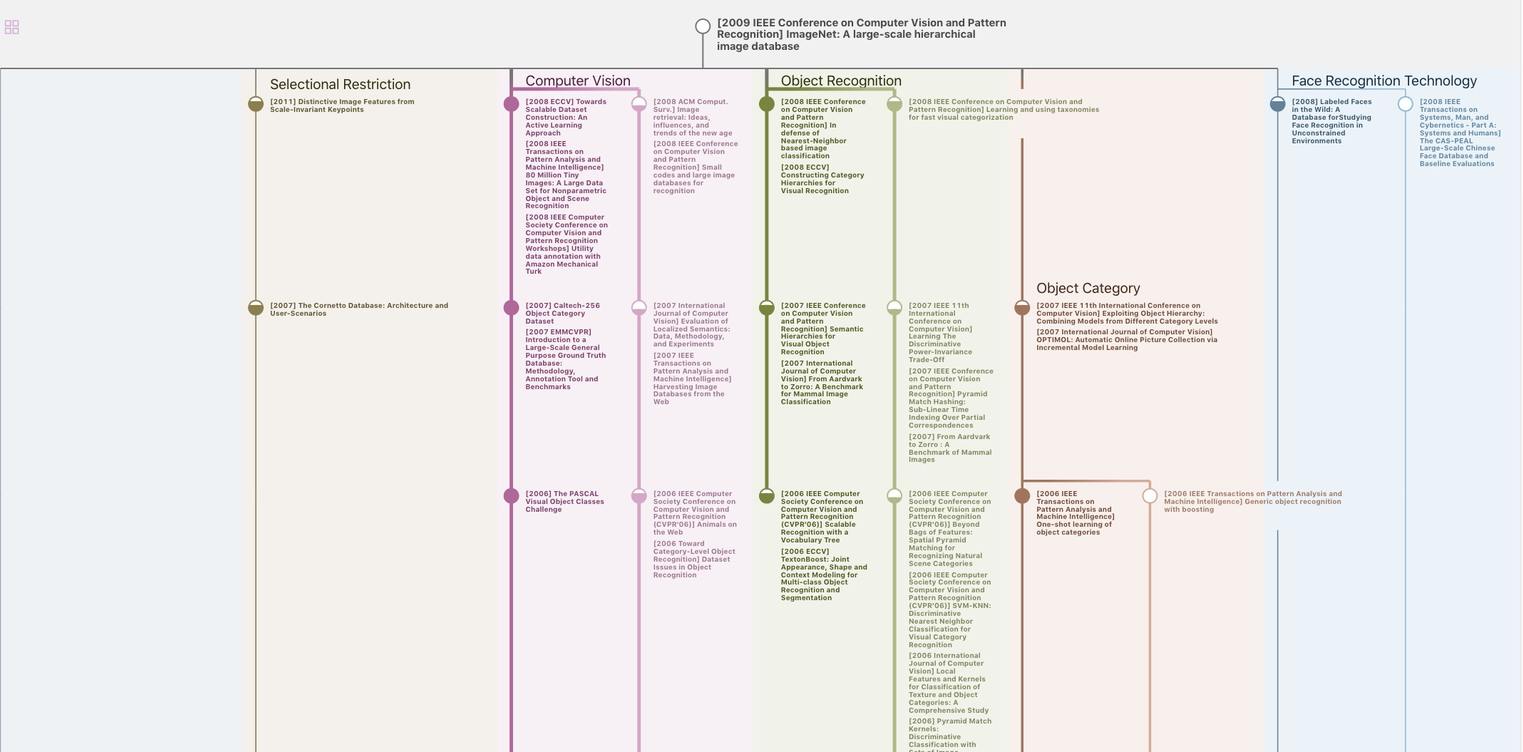
生成溯源树,研究论文发展脉络
Chat Paper
正在生成论文摘要