Two-step attribute reduction for AIoT networks
IET COMMUNICATIONS(2024)
摘要
The evolution of Artificial Intelligence of Things (AIoT) pushes connectivity from human-to-things and things-to-things, to AI-to-things, has resulted in more complex physical networks and logical associations. This has driven the demand for Internet of Things (IoT) devices with powerful edge data processing capabilities, leading to exponential growth in device quantity and data generation. However, conventional data preprocessing methods, such as data compression and encoding, often require edge devices to allocate computational resources for decoding. Additionally, some lossy compression methods, like JPEG, may result in the loss of important information, which has negative impact on the AI training. To address these challenges, this paper proposes a two-step attribute reduction approach, targeting devices and dimensions, to reduce the massive amount of data in the AIoT network while avoiding unnecessary utilization of edge device resources for decoding. The device-oriented and dimension-oriented attribute reductions identify important devices and dimensions, respectively, to mitigate the multimodal interference caused by the large-scale devices in the AIoT network and the curse of dimensionality associated with high-dimensional AIoT data. Numerical results and analysis show that this approach effectively eliminates redundant devices and numerous dimensions in the AIoT network while maintaining the basic data correlation. This paper focuses on the challenge of generating a substantial volume of data in AIoT networks, which arises from the widespread deployment of devices and the utilization of high-dimensional data. To alleviate the pressure on edge computing and communication channel resources, a novel approach is proposed. This approach involves device-oriented and dimension-oriented attribute reduction techniques, which not only reduce the workload on edge computing resources but also offer potential solutions to mitigate multimodal interference caused by the extensive deployment of devices in AIoT networks, as well as the curse of dimensionality associated with high-dimensional data in AIoT networks. image
更多查看译文
关键词
data aggregation,data communication digital communication,Internet of Things
AI 理解论文
溯源树
样例
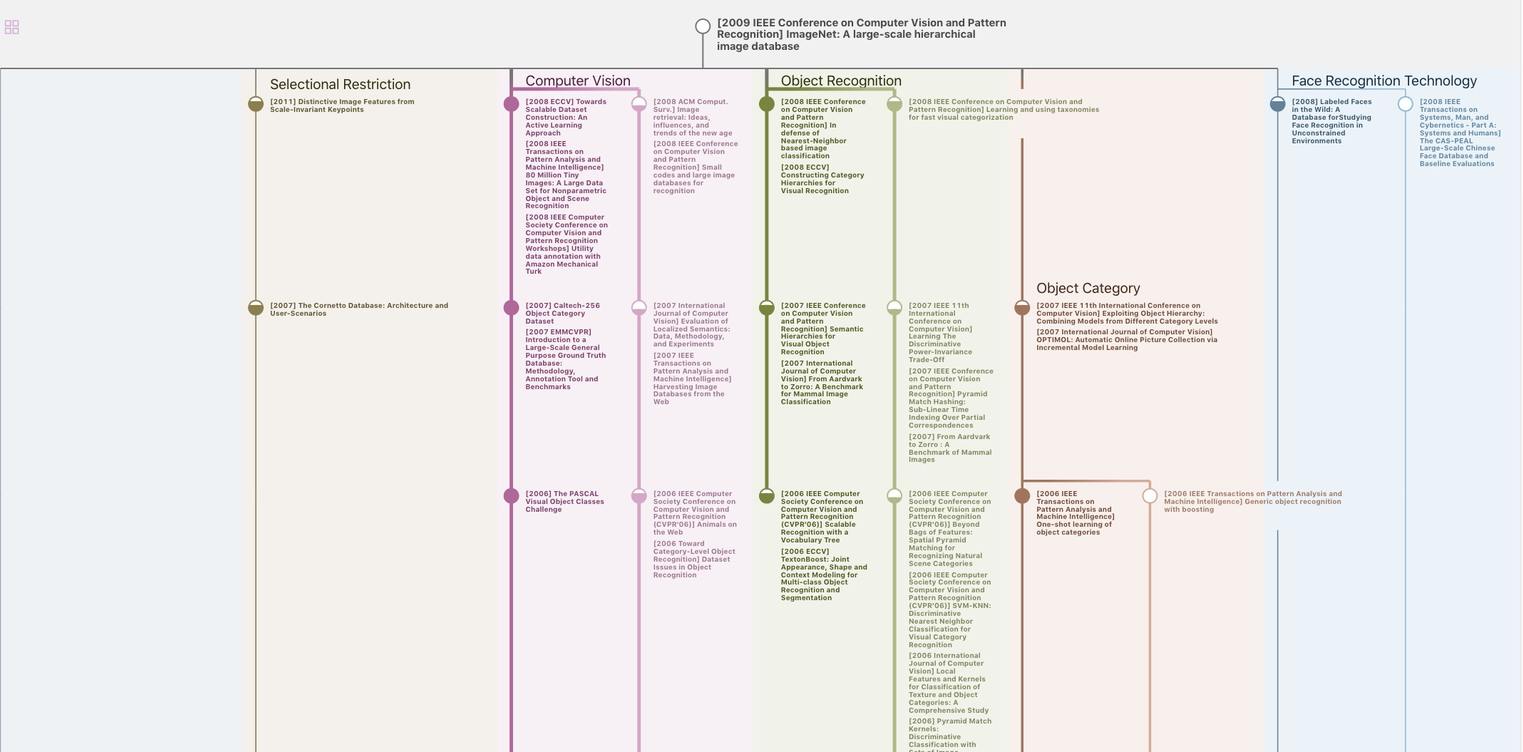
生成溯源树,研究论文发展脉络
Chat Paper
正在生成论文摘要