Coronary Artery Stenosis and High-Risk Plaque Assessed With an Unsupervised Fully Automated Deep Learning Technique
JACC: Advances(2024)
摘要
Background
Coronary computed tomography angiography (CCTA) has emerged as a reliable noninvasive modality to assess coronary artery stenosis and high-risk plaque (HRP). However, CCTA assessment of stenosis and HRP is time-consuming and requires specialized training, limiting its clinical translation.
Objectives
The aim of this study is to develop and validate a fully automated deep learning system capable of characterizing stenosis severity and HRP on CCTA.
Methods
A deep learning system was trained to assess stenosis and HRP on CCTA scans from 570 patients in multiple centers. Stenosis severity was categorized as >0%, 1 to 49%, ≥50%, and ≥70%. HRP was defined as low attenuation plaque (≤30 HU), positive remodeling (≥10% diameter), and spotty calcification (<3 mm). The model was then tested on 769 patients (3,012 vessels) for stenosis severity and 45 patients (325 vessels) for HRP.
Results
Our deep learning system achieved 93.5% per-vessel agreement within 1 Coronary Artery Disease-Reporting and Data System (CAD-RADS) category for stenosis. Diagnostic performance for per-vessel stenosis was very good for sensitivity, specificity, positive predictive value, negative predictive value, and area under the curve with: >0% stenosis: 90.6%, 88.8%, 83.4%, 93.9%, 89.7%, respectively; ≥50% stenosis: 87.1%, 92.3%, 60.9%, 98.1%, 89.7%, respectively. Similarly, the per-vessel HRP feature achieved very good diagnostic performance with an area under the curve of 0.80, 0.79, and 0.77 for low attenuation plaque, spotty calcification, and positive remodeling, respectively.
Conclusions
A fully automated unsupervised deep learning system can rapidly evaluate stenosis severity and characterize HRP with very good diagnostic performance on CCTA.
更多查看译文
关键词
artificial intelligence,cardiac computed tomography,coronary artery disease,deep learning
AI 理解论文
溯源树
样例
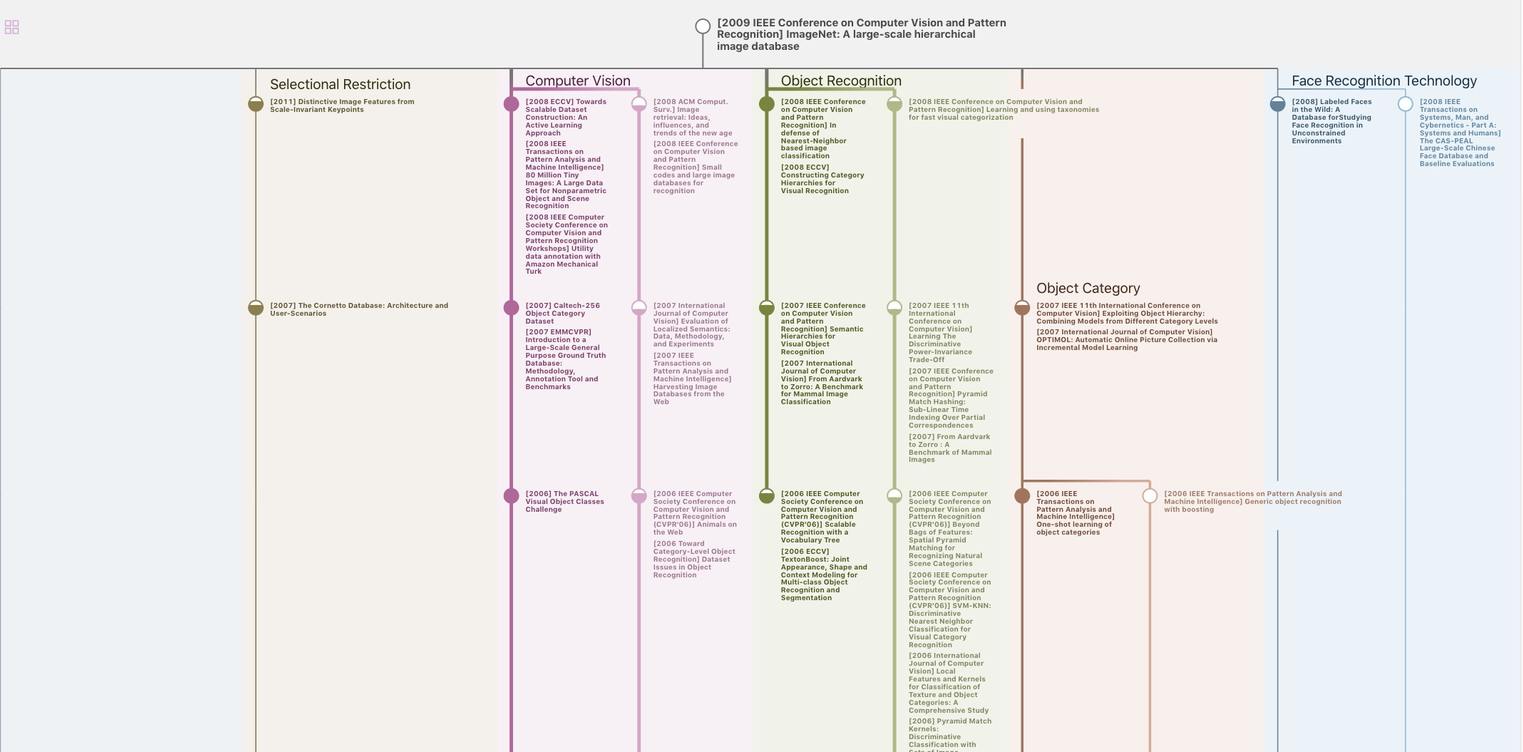
生成溯源树,研究论文发展脉络
Chat Paper
正在生成论文摘要