Dcl-Net: Dual Contrastive Learning Network for Semi-Supervised Multi-Organ Segmentation
ICASSP 2024 - 2024 IEEE International Conference on Acoustics, Speech and Signal Processing (ICASSP)(2024)
摘要
Semi-supervised learning is a sound measure to relieve the strict demand of
abundant annotated datasets, especially for challenging multi-organ
segmentation . However, most existing SSL methods predict pixels in a single
image independently, ignoring the relations among images and categories. In
this paper, we propose a two-stage Dual Contrastive Learning Network for
semi-supervised MoS, which utilizes global and local contrastive learning to
strengthen the relations among images and classes. Concretely, in Stage 1, we
develop a similarity-guided global contrastive learning to explore the implicit
continuity and similarity among images and learn global context. Then, in Stage
2, we present an organ-aware local contrastive learning to further attract the
class representations. To ease the computation burden, we introduce a mask
center computation algorithm to compress the category representations for local
contrastive learning. Experiments conducted on the public 2017 ACDC dataset and
an in-house RC-OARs dataset has demonstrated the superior performance of our
method.
更多查看译文
关键词
Semi-supervised learning,multi-organ segmentation,contrastive learning,deep learning
AI 理解论文
溯源树
样例
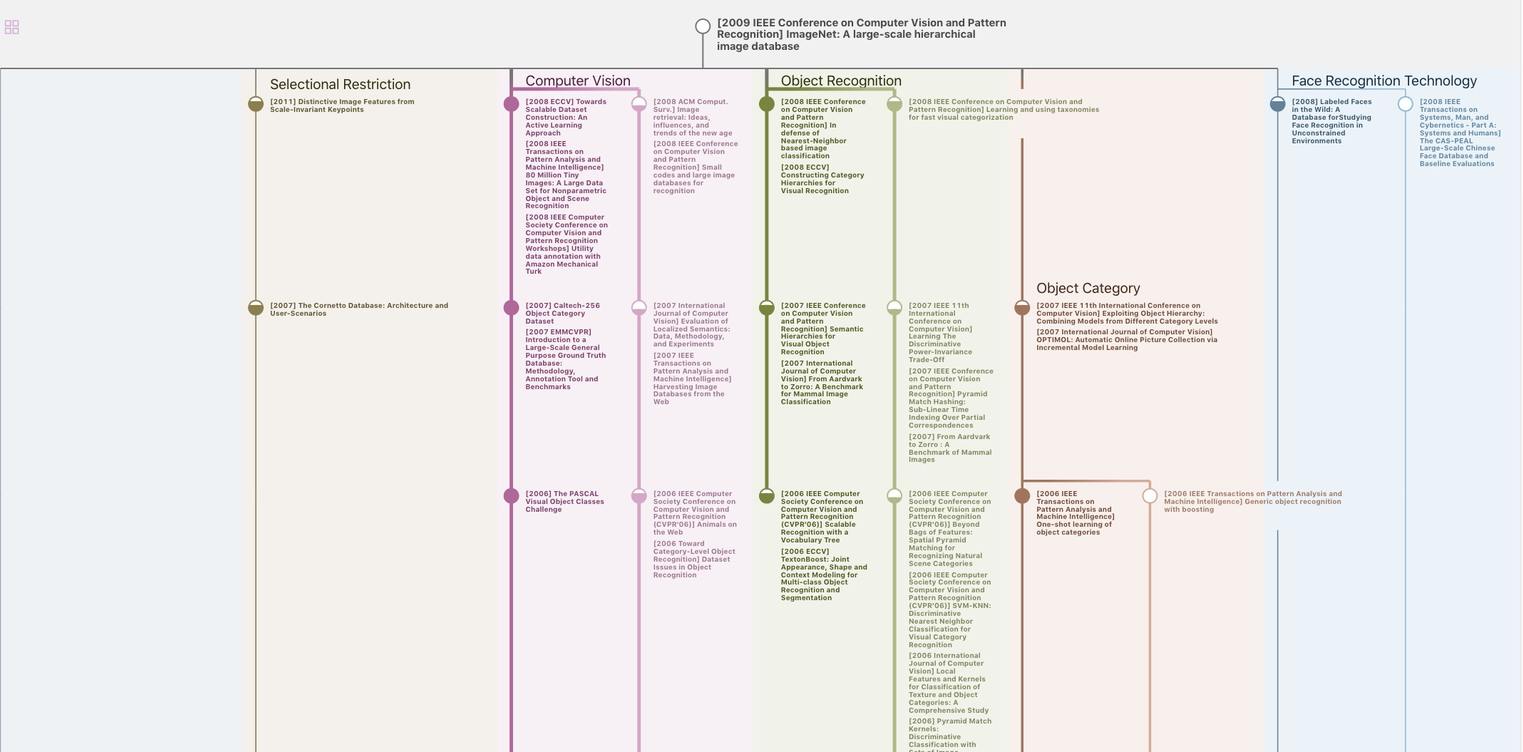
生成溯源树,研究论文发展脉络
Chat Paper
正在生成论文摘要