Actuation manifold from snapshot data
arxiv(2024)
摘要
We propose a data-driven methodology to learn a low-dimensional actuation
manifold of controlled flows. The starting point is resolving snapshot flow
data for a representative ensemble of actuations. Key enablers for the
actuation manifold are isometric mapping as encoder and k-nearest neighbour
regression as a decoder. This methodology is tested for the fluidic pinball, a
cluster of three parallel cylinders perpendicular to the oncoming uniform flow.
The centers of these cylinders are the vertices of an equilateral triangle
pointing upstream. The flow is manipulated by constant rotation of the
cylinders, i.e. described by three actuation parameters. The Reynolds number
based on a cylinder diameter is chosen to be 30. The unforced flow yields
statistically symmetric unforced periodic shedding represented by a
one-dimensional limit cycle. The proposed methodology yields a five-dimensional
manifold describing a wide range of dynamics with small representation error.
Interestingly, the manifold coordinates automatically unveil physically
meaningful parameters. Two of them describe the downstream periodic vortex
shedding. The other three ones describe the near-field actuation, i.e. the
strength of boat-tailing, the Magnus effect and forward stagnation point. The
manifold is shown to be a key enabler for control-oriented flow estimation.
更多查看译文
AI 理解论文
溯源树
样例
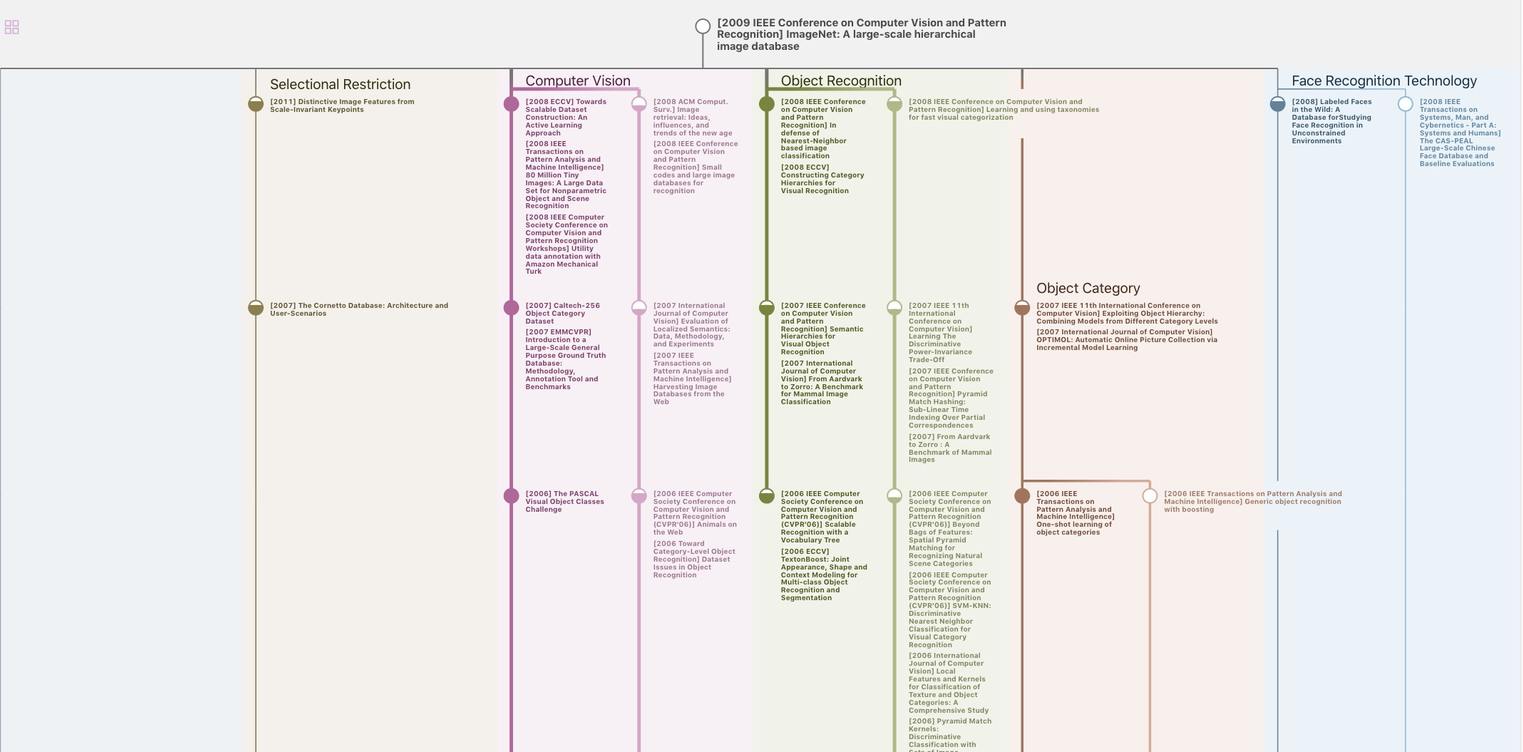
生成溯源树,研究论文发展脉络
Chat Paper
正在生成论文摘要