Black-Box k-to-1-PCA Reductions: Theory and Applications
arxiv(2024)
摘要
The k-principal component analysis (k-PCA) problem is a fundamental
algorithmic primitive that is widely-used in data analysis and dimensionality
reduction applications. In statistical settings, the goal of k-PCA is to
identify a top eigenspace of the covariance matrix of a distribution, which we
only have implicit access to via samples. Motivated by these implicit settings,
we analyze black-box deflation methods as a framework for designing k-PCA
algorithms, where we model access to the unknown target matrix via a black-box
1-PCA oracle which returns an approximate top eigenvector, under two popular
notions of approximation. Despite being arguably the most natural
reduction-based approach to k-PCA algorithm design, such black-box methods,
which recursively call a 1-PCA oracle k times, were previously
poorly-understood.
Our main contribution is significantly sharper bounds on the approximation
parameter degradation of deflation methods for k-PCA. For a quadratic form
notion of approximation we term ePCA (energy PCA), we show deflation methods
suffer no parameter loss. For an alternative well-studied approximation notion
we term cPCA (correlation PCA), we tightly characterize the parameter regimes
where deflation methods are feasible. Moreover, we show that in all feasible
regimes, k-cPCA deflation algorithms suffer no asymptotic parameter loss for
any constant k. We apply our framework to obtain state-of-the-art k-PCA
algorithms robust to dataset contamination, improving prior work both in sample
complexity and approximation quality.
更多查看译文
AI 理解论文
溯源树
样例
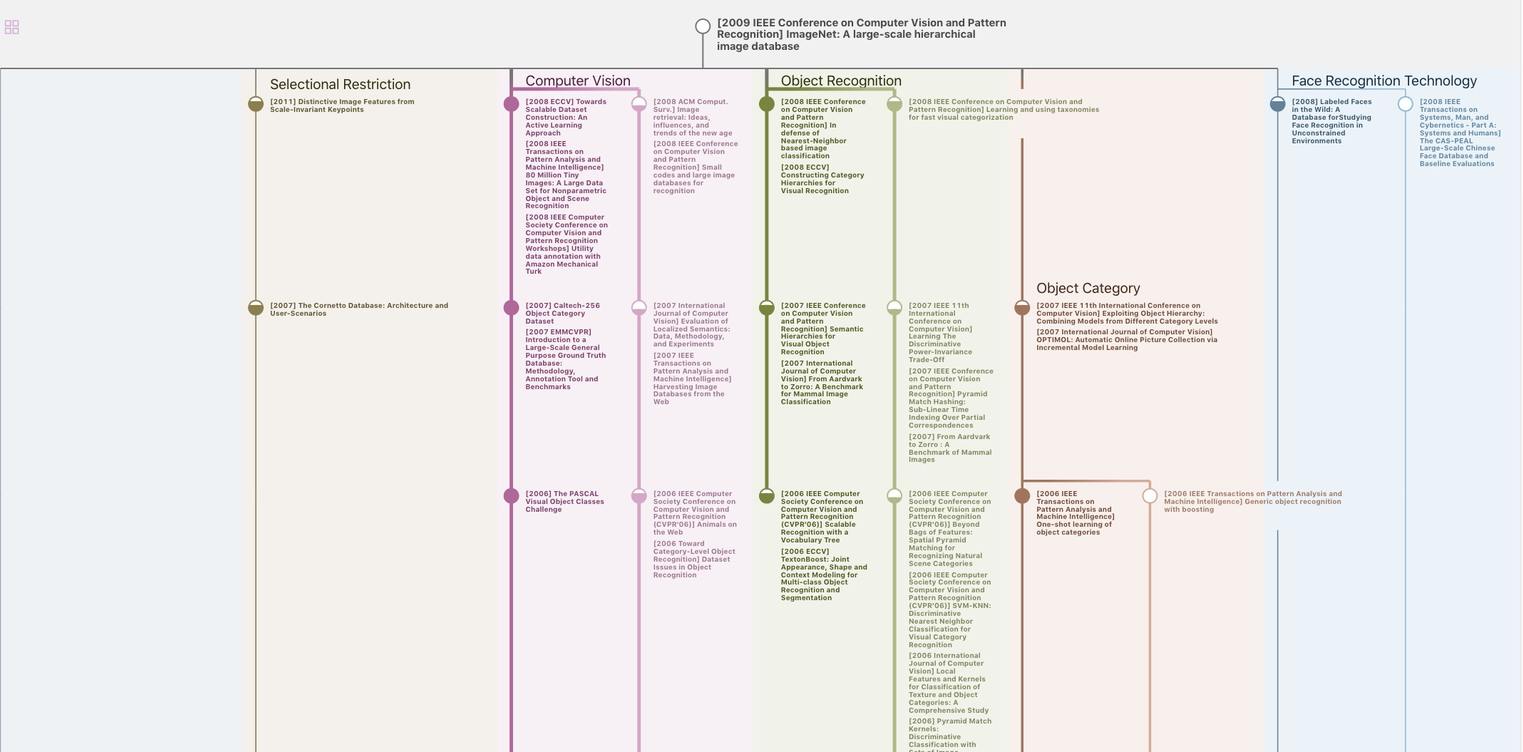
生成溯源树,研究论文发展脉络
Chat Paper
正在生成论文摘要