Development and validation of a deep learning radiomics model with clinical-radiological characteristics for the identification of occult peritoneal metastases in patients with pancreatic ductal adenocarcinoma.
International journal of surgery (London, England)(2024)
摘要
BACKGROUND:Occult peritoneal metastases (OPM) in patients with pancreatic ductal adenocarcinoma (PDAC) are frequently overlooked during imaging. We aimed to develop and validate a CT-based deep learning-based radiomics (DLR) model to identify OPM in PDAC before treatment.
METHODS:This retrospective, bicentric study included 302 patients with PDAC (training: n=167, OPM-positive, n=22; internal test: n=72, OPM-positive, n=9: external test, n=63, OPM-positive, n=9) who had undergone baseline CT examinations between January 2012 and October 2022. Handcrafted radiomics (HCR) and DLR features of the tumor and HCR features of peritoneum were extracted from CT images. Mutual information and least absolute shrinkage and selection operator algorithms were used for feature selection. A combined model, which incorporated the selected clinical-radiological, HCR, and DLR features, was developed using a logistic regression classifier using data from the training cohort and validated in the test cohorts.
RESULTS:Three clinical-radiological characteristics (carcinoembryonic antigen 19-9 and CT-based T and N stages), nine HCR features of the tumor, 14 DLR features of the tumor and three HCR features of the peritoneum were retained after feature selection. The combined model yielded satisfactory predictive performance, with an area under the curve (AUC) of 0.853 (95% confidence interval [CI], 0.790-0.903), 0.845 (95% CI, 0.740-0.919), and 0.852 (95% CI, 0.740-0.929) in the training, internal test, and external test cohorts, respectively (all P<0.05). The combined model showed better discrimination than the clinical-radiological model in the training (AUC=0.853 vs. 0.612, P<0.001) and the total test (AUC=0.842 vs. 0.638, P<0.05) cohorts. The decision curves revealed that the combined model had greater clinical applicability than the clinical-radiological model.
CONCLUSIONS:The model combining CT-based deep learning radiomics and clinical-radiological features showed satisfactory performance for predicting occult peritoneal metastases in patients with pancreatic ductal adenocarcinoma.
更多查看译文
AI 理解论文
溯源树
样例
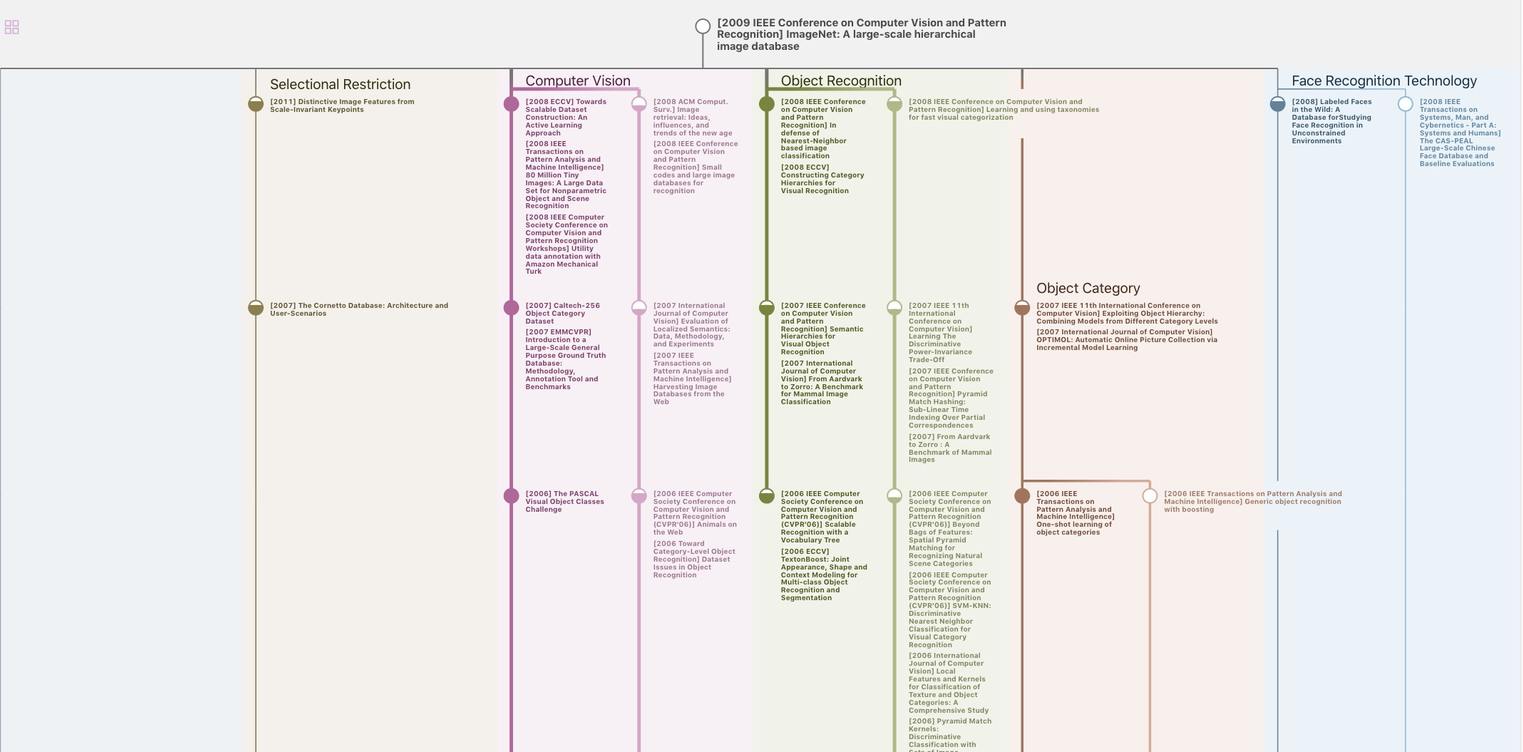
生成溯源树,研究论文发展脉络
Chat Paper
正在生成论文摘要