WU-Net plus plus : A novel enhanced Weighted U-Net plus plus model for brain tumor detection and segmentation from multi-parametric magnetic resonance scans
MULTIMEDIA TOOLS AND APPLICATIONS(2024)
摘要
Brain tumor detection and segmentation from multi-parametric magnetic resonance (MR) scans are crucial for the prognosis and treatment planning of brain tumor patients in current clinical practice. With recent technological advancements, artificial intelligence-based deep learning has proven its indispensable image analysis capability in the most challenging tasks. This study proposes an automated WU-Net + + deep learning model for brain tumor segmentation using multiparametric structural MR scans obtained from the BraTS 2018 dataset. The model was validated through cross-dataset testing for intracranial hemorrhage (ICH) classification (ATLAS V2.1 dataset) and multi-organ segmentation from abdominal images of TCIA and BTCV datasets. The WU-Net++ model, a novel version of U-Net, was developed by adjusting its pooling operation as a weighted function of max and average pooling for brain tumor segmentation. The proposed model (WU-Net + +) achieved an F1 score of 0.94 +/- 0.124, a dice score of 0.91 +/- 0.132, and an AUC value of 0.915 for whole tumor segmentation. The model also achieved a high accuracy of 0.9949 +/- 0.121 in ICH classification and dice scores of 0.912 +/- 0.21, 0.844 +/- 0.25, and 0.893 +/- 0.17 for spleen, esophagus, and portal and splenic vein segmentation, respectively. Our study revealed that WU-Net + + has significant potential to improve the accuracy of segmentation and could be an effective method in the era of precision medicine.
更多查看译文
关键词
Brain tumor detection,Segmentation,WU-Net plus plus,Deep learning,Machine learning,Magnetic resonance imaging (MRI)
AI 理解论文
溯源树
样例
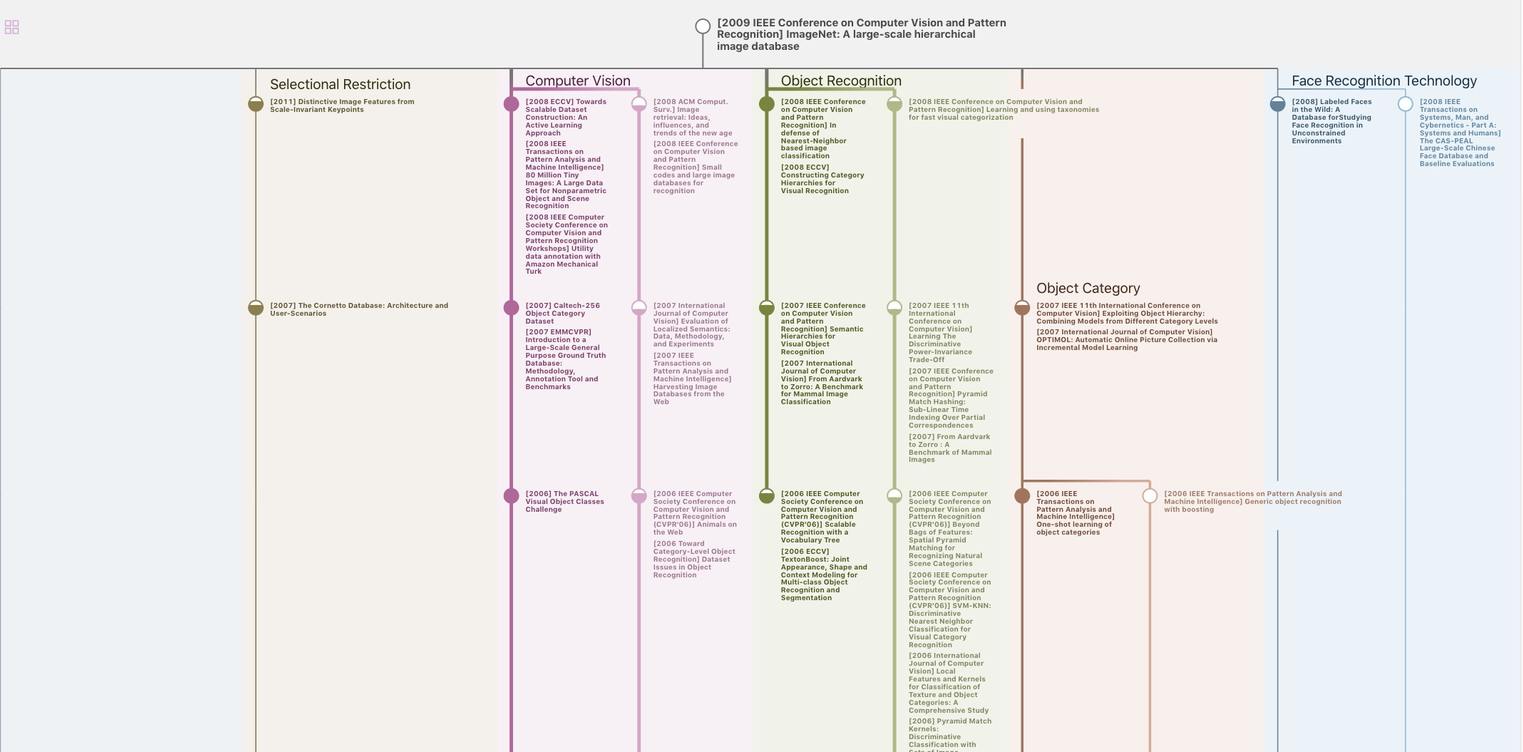
生成溯源树,研究论文发展脉络
Chat Paper
正在生成论文摘要