Learning Cooperative Beamforming with Edge-Update Empowered Graph Neural Networks
ICC 2023 - IEEE INTERNATIONAL CONFERENCE ON COMMUNICATIONS(2023)
摘要
Cooperative beamforming has been recognized as an effective approach to meet the dramatically increasing demand of various wireless data traffics. Conventionally, the beamforming design problem is posed as an optimization problem and solved through iterative algorithms, which are difficult for real-time implementations. Recent advances in the field have witnessed the emergence of learning-based methods for beamforming design in real-time. Graph Neural Networks (GNNs) have been demonstrated to leverage the graph topology in wireless networks and generalize to unseen problem sizes. However, the current implementations of GNNs suffer from a limitation in modeling more complex cooperative beamforming, where the beamformers are on the graph edges. To address this shortcoming, this paper presents a novel Edge-Graph-Neural-Network (Edge-GNN) which incorporates an edge-update mechanism, thus allowing for the learning of cooperative beamforming on graph edges. Simulation results affirm the superiority of the proposed Edge-GNN over state-of-the-art approaches. The Edge-GNN achieves a higher sum rate with reduced computation time and exhibits excellent generalization to different numbers of base stations and user equipments.
更多查看译文
关键词
Cooperative beamforming,edge-update mechanism,graph neural network (GNN),permutation equivariance (PE)
AI 理解论文
溯源树
样例
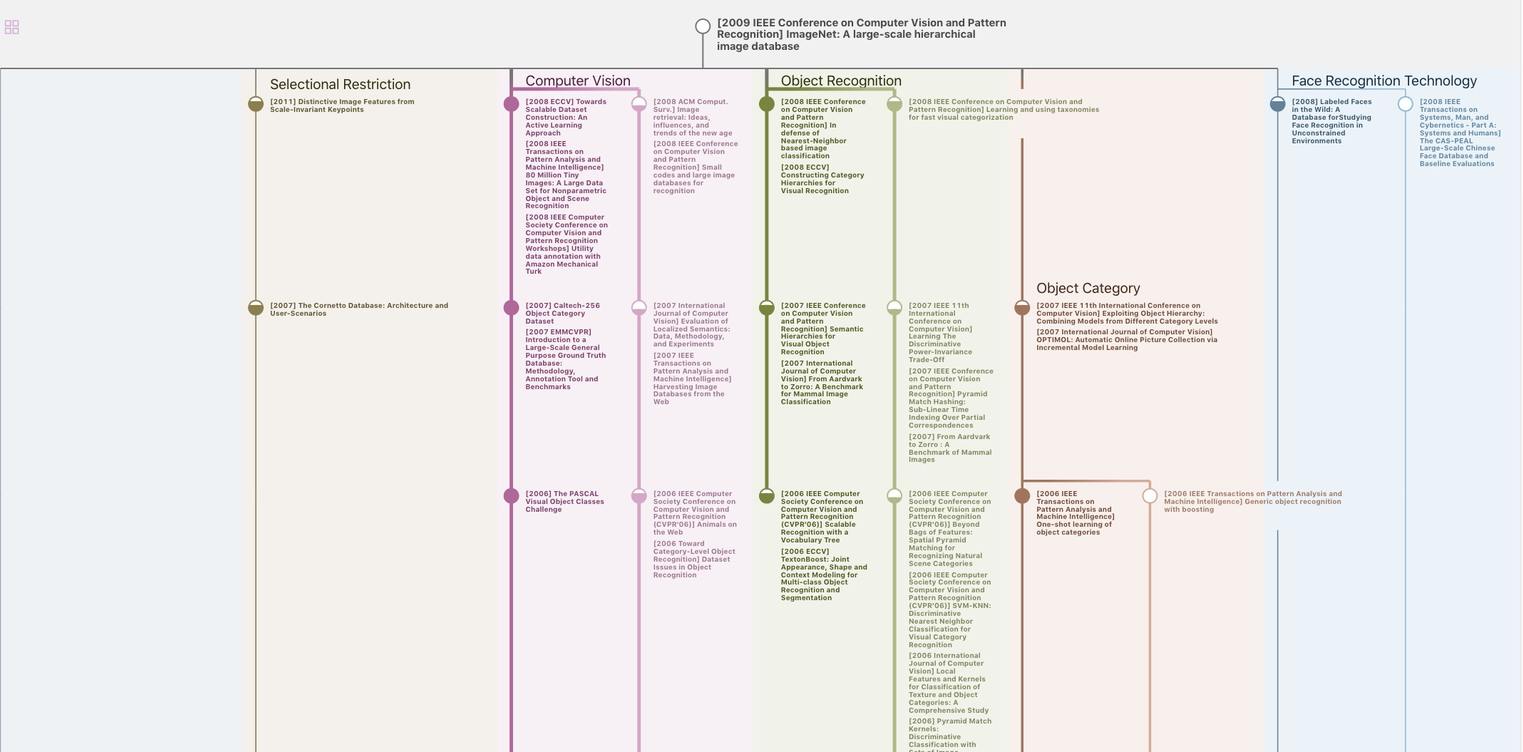
生成溯源树,研究论文发展脉络
Chat Paper
正在生成论文摘要