Bayesian small area models under inequality constraints with benchmarking and double shrinkage
SURVEY METHODOLOGY(2023)
摘要
We present a novel methodology to benchmark county -level estimates of crop area totals to a preset state total subject to inequality constraints and random variances in the Fay-Herriot model. For planted area of the National Agricultural Statistics Service (NASS), an agency of the United States Department of Agriculture (USDA), it is necessary to incorporate the constraint that the estimated totals, derived from survey and other auxiliary data, are no smaller than administrative planted area totals prerecorded by other USDA agencies except NASS. These administrative totals are treated as fixed and known, and this additional coherence requirement adds to the complexity of benchmarking the county -level estimates. A fully Bayesian analysis of the Fay-Herriot model offers an appealing way to incorporate the inequality and benchmarking constraints, and to quantify the resulting uncertainties, but sampling from the posterior densities involves difficult integration, and reasonable approximations must be made. First, we describe a single -shrinkage model, shrinking the means while the variances are assumed known. Second, we extend this model to accommodate double shrinkage, borrowing strength across means and variances. This extended model has two sources of extra variation, but because we are shrinking both means and variances, it is expected that this second model should perform better in terms of goodness of fit (reliability) and possibly precision. The computations are challenging for both models, which are applied to simulated data sets with properties resembling the Illinois corn crop.
更多查看译文
关键词
Devroye method,Fay-Herriot model,Grid method,Hierarchical Bayesian model,Metropolis sampler
AI 理解论文
溯源树
样例
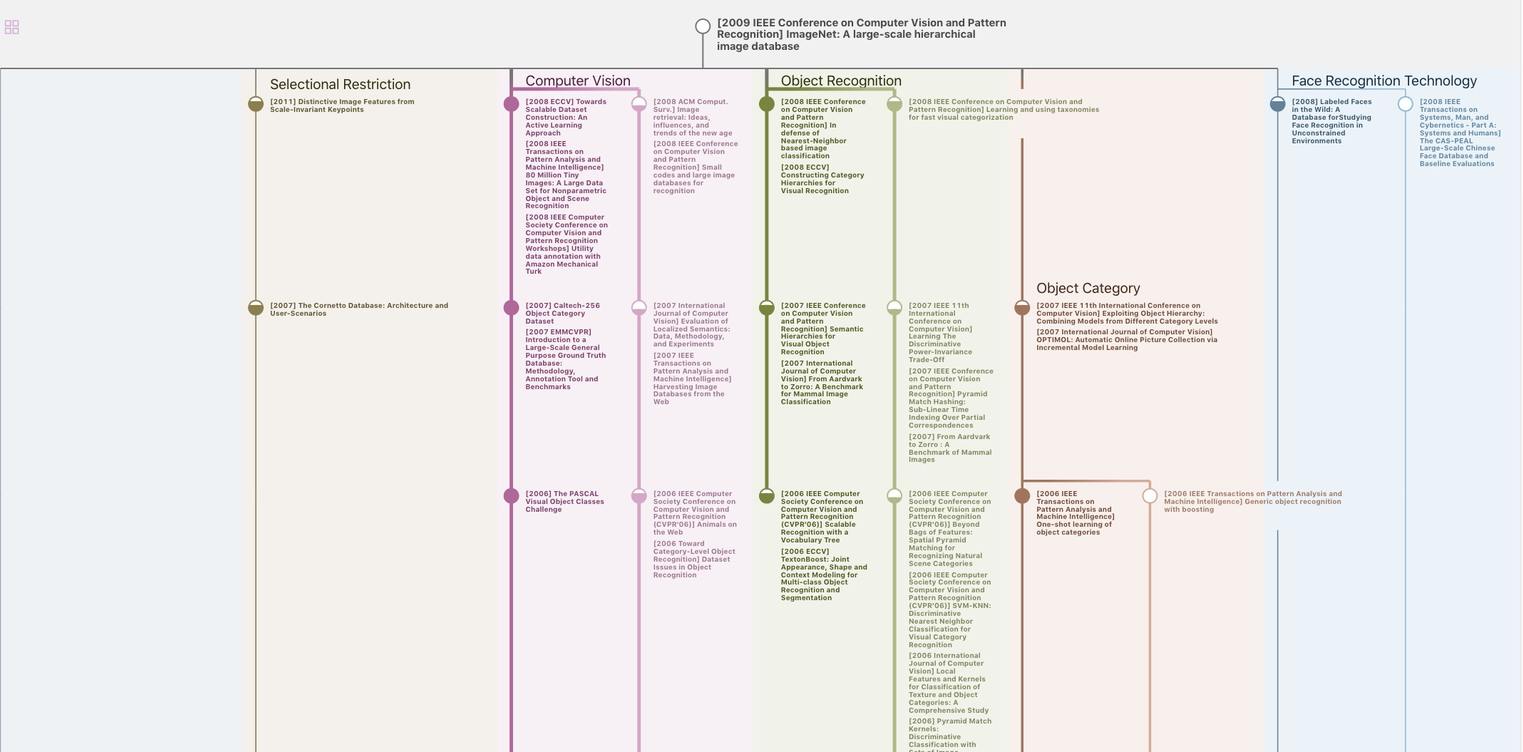
生成溯源树,研究论文发展脉络
Chat Paper
正在生成论文摘要